Spatial conservation prioritisation in data-poor countries: a quantitative sensitivity analysis using multiple taxa
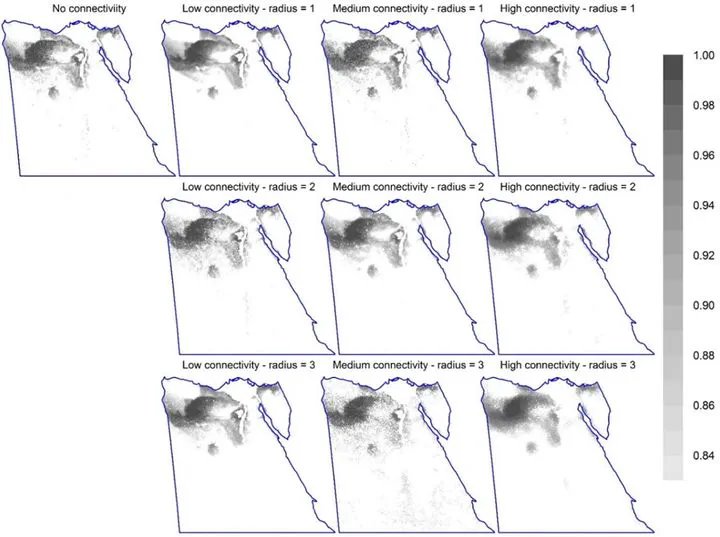
Background: Spatial conservation prioritisation (SCP) is a set of computational tools designed to support the efficient spatial allocation of priority areas for conservation actions, but it is subject to many sources of uncertainty which should be accounted for during the prioritisation process. We quantified the sensitivity of an SCP application (using software Zonation) to possible sources of uncertainty in data-poor situations, including the use of different surrogate options; correction for sampling bias; how to integrate connectivity; the choice of species distribution modelling (SDM) algorithm; how cells are removed from the landscape; and two methods of assigning weights to species (red-list status or prediction uncertainty). Further, we evaluated the effectiveness of the Egyptian protected areas for conservation, and spatially allocated the top priority sites for further on-the-ground evaluation as potential areas for protected areas expansion.
Results: Focal taxon (butterflies, reptiles, and mammals), sampling bias, connectivity and the choice of SDM algorithm were the most sensitive parameters; collectively these reflect data quality issues. In contrast, cell removal rule and species weights contributed much less to overall variability. Using currently available species data, we found the current effectiveness of Egypt’s protected areas for conserving fauna was low.
Conclusions: For SCP to be useful, there is a lower limit on data quality, requiring data-poor countries to improve sampling strategies and data quality to obtain unbiased data for as many taxa as possible. Since our sensitivity analysis may not generalise, conservation planners should use sensitivity analyses more routinely, particularly relying on more than one combination of SDM algorithm and surrogate group, consider correction for sampling bias, and compare the spatial patterns of predicted priority sites using a variety of settings. The sensitivity of SCP to connectivity parameters means that the responses of each species to habitat loss are important knowledge gaps.
See also:
- Appendix 2
- A shiny app for priotization results of the 2,560 zonation runs and interactive spatial distribution of Egyptian fauna.
References:
- Polasky S, Camm JD, Solow AR, Csuti B, White D, Ding R. Choosing reserve networks with incomplete species information. Biol Conserv. 2000;94:1–10. doi:10.1016/S0006-3207(99)00171-8.
- Moilanen A, Franco AMA, Early RI, Fox R, Wintle B, Thomas CD. Prioritizing multiple-use landscapes for conservation. Proc. Biol. Sci. 2005;272:1885–91. doi:10.1098/rspb.2005.3164.
- Wilson KA, Carwardine J, Possingham HP. Setting conservation priorities. Ann. N. Y. Acad. Sci. 2009;1162:237–64. doi:10.1111/j.1749-6632.2009.04149.x.
- Butchart SHM, Scharlemann JPW, Evans MI, Quader S, Aricò S, Arinaitwe J, et al. Protecting important sites for biodiversity contributes to meeting global conservation targets. PLoS One. 2012;7:e32529. doi:10.1371/journal.pone.0032529.
- Brum FT, Graham CH, Costa GC, Hedges SB, Penone C, Radeloff VC, et al. Global priorities for conservation across multiple dimensions of mammalian diversity. Proceedings of the National Academy of Sciences USA. 2017;114:7641–6. doi:10.1073/pnas.1706461114.
- UNEP-WCMC, IUCN. Protected planet report 2016: UNEP-WCMC and IUCN: Cambridge UK and Gland, Switzerland.; 2016.
- Franco AMA, Anderson BJ, Roy DB, Gillings S, Fox R, Moilanen A, Thomas CD. Surrogacy and persistence in reserve selection: landscape prioritization for multiple taxa in Britain. J Appl Ecol. 2009;46:82–91. doi:10.1111/j.1365-2664.2008.01598.x.
- Moilanen A. Spatial conservation prioritization in data-poor areas of the world. Nat. Conserv. 2012;10:12–9. doi:10.4322/natcon.2012.003.
- Andrello M, Jacobi MN, Manel S, Thuiller W, Mouillot D. Extending networks of protected areas to optimize connectivity and population growth rate. Ecography. 2015;38:273–82. doi:10.1111/ecog.00975.
- Pressey RL. Ad hoc reservations: forward or backward steps in developing representative reserve systems? Conserv. Biol. 1994;8:662–8. doi:10.1046/j.1523-1739.1994.08030662.x.
- Freitag S, Nicholls AO, Van Jaarsveld AS. Dealing with established reserve networks and incomplete distribution data sets in conservation planning. South African Journal of Science. 1998;94.
- Kukkala AS, Moilanen A. Core concepts of spatial prioritisation in systematic conservation planning. Biological Reviews. 2013;88:443–64. doi:10.1111/brv.12008.
- Margules CR, Pressey RL. Systematic conservation planning. Nature. 2000;405:243–53. doi:10.1038/35012251.
- Venter O, Magrach A, Outram N, Klein CJ, Possingham HP, Di Marco M, Watson. Bias in protected-area location and its effects on long-term aspirations of biodiversity conventions. Conserv. Biol. 2018;32:127–34. doi:10.1111/cobi.12970.
- CBD. Aichi biodiversity targets of the strategic plan 2011–2020. http://www.cbd.int/sp/targets/. 2010.
- Di Minin E, Toivonen T. Global protected area expansion: creating more than paper parks. Bioscience. 2015;65:637–8. doi:10.1093/biosci/biv064.
- Di Minin E, Moilanen A. Improving the surrogacy effectiveness of charismatic megafauna with well-surveyed taxonomic groups and habitat types. J Appl Ecol. 2014;51:281–8. doi:10.1111/1365-2664.12203.
- Possingham H, Wilson KA, Andelman SJ, Vynne CH. Protected areas: goals, limitations, and design. Principles of conservation biology, 3rd edition (ed M.J. Groom et al.). Sinauer, Mass. In:
- Lentini PE, Wintle BA. Spatial conservation priorities are highly sensitive to choice of biodiversity surrogates and species distribution model type. Ecography. 2015;38:1101–11. doi:10.1111/ecog.01252.
- Guisan A, Tingley R, Baumgartner JB, Naujokaitis-Lewis I, Sutcliffe PR, Tulloch AIT, et al. Predicting species distributions for conservation decisions. Ecol. Lett. 2013;16:1424–35. doi:10.1111/ele.12189.
- Guisan A, Zimmermann NE. Predictive habitat distribution models in ecology. Ecol Model. 2000;135:147–86. doi:10.1016/S0304-3800(00)00354-9.
- Dormann CF, Purschke O, Márquez RGJ, Lautenbach S, Schröder B. Components of uncertainty in species distribution analysis. Ecology. 2008;89:3371–86. doi:10.1890/07-1772.1.
- Phillips SJ, Dudík M, Elith J, Graham CH, Lehmann A, Leathwick J, Ferrier S. Sample selection bias and presence-only distribution models. Ecological Applications. 2009;19:181–97. doi:10.1890/07-2153.1.
- Rondinini C, Wilson KA, Boitani L, Grantham H, Possingham HP. Tradeoffs of different types of species occurrence data for use in systematic conservation planning. Ecol. Lett. 2006;9:1136–45. doi:10.1111/j.1461-0248.2006.00970.x.
- Warton DI, Renner IW, Ramp D. Model-based control of observer bias for the analysis of presence-only data in ecology. PLoS One. 2013;8:e79168. doi:10.1371/journal.pone.0079168.
- Tulloch AIT, Sutcliffe P, Naujokaitis-Lewis I, Tingley R, Brotons L, Ferraz KMPMB, et al. Conservation planners tend to ignore improved accuracy of modelled species distributions to focus on multiple threats and ecological processes. Biol Conserv. 2016;199:157–71. doi:10.1016/j.biocon.2016.04.023.
- Kool JT, Moilanen A, Treml EA. Population connectivity: recent advances and new perspectives. Landscape Ecol. 2013;28:165–85. doi:10.1007/s10980-012-9819-z.
- Possingham H, Ball I, Andelman S. Mathematical methods for identifying representative reserve networks. In: Ferson S, Burgman M, editors. Quantitative methods for conservation biology. New York: Springer; 2000. p. 291–306. doi:10.1007/0-387-22648-6_17.
- Briers RA. Incorporating connectivity into reserve selection procedures. Biol Conserv. 2002;103:77–83. doi:10.1016/S0006-3207(01)00123-9.
- Moilanen A, Wintle BA. The boundary-quality penalty: a quantitative method for approximating species responses to fragmentation in reserve selection. Conserv. Biol. 2007;21:355–64. doi:10.1111/j.1523-1739.2006.00625.x.
- Moilanen A, Wintle BA, Elith J, Burgman M. Uncertainty analysis for regional-scale reserve selection. Conserv. Biol. 2006;20:1688–97. doi:10.1111/j.1523-1739.2006.00560.x.
- Wilson KA. Dealing with data uncertainty in conservation planning. Natureza & Conservação. 2010;08:145–50. doi:10.4322/natcon.00802007.
- Gilbert F, Zalat S. Butterflies of Egypt. EEAA, Cairo.; 2007.
- El-Gabbas A, Baha El Din S, Zalat S, Gilbert F. Conserving Egypt’s reptiles under climate change. Journal of Arid Environments. 2016;127:211–21. doi:10.1016/j.jaridenv.2015.12.007.
- Basuony MI, Gilbert F, Zalat S. Mammals of Egypt. BioMAP, EEAA, Cairo.; 2010.
- Phillips SJ, Anderson RP, Schapire RE. Maximum entropy modeling of species geographic distributions. Ecol Model. 2006;190:231–59. doi:10.1016/j.ecolmodel.2005.03.026.
- Friedman JH, Hastie T, Tibshiani R. Regularization paths for generalized linear models via coordinate descent. Journal of Statistical Software. 2010;33.
- Renner IW, Elith J, Baddeley A, Fithian W, Hastie T, Phillips SJ, et al. Point process models for presence-only analysis. Methods Ecol Evol. 2015;6:366–79. doi:10.1111/2041-210X.12352.
- El-Gabbas A, Dormann CF. Improved species-occurrence predictions in data-poor regions: using large-scale data and bias correction with down-weighted Poisson regression and Maxent. Ecography. 2017;41:1161–72. doi:10.1111/ecog.03149.
- Schoener TW. The Anolis lizards of Bimini: resource partitioning in a complex fauna. Ecology. 1968;49:704. doi:10.2307/1935534.
- Moilanen A. Landscape Zonation, benefit functions and target-based planning. Biol Conserv. 2007;134:571–9. doi:10.1016/j.biocon.2006.09.008.
- Lehtomäki J, Moilanen A. Methods and workflow for spatial conservation prioritization using Zonation. Environmental Modelling & Software. 2013;47:128–37. doi:10.1016/j.envsoft.2013.05.001.
- Bode M, Watson J, Iwamura T, Possingham HP. The cost of conservation. Science. 2008;321:340. doi:10.1126/science.321.5887.340a.
- Naidoo R, Balmford A, Ferraro PJ, Polasky S, Ricketts TH, Rouget M. Integrating economic costs into conservation planning. Trends Ecol. Evol. 2006;21:681–7. doi:10.1016/j.tree.2006.10.003.
- Newbold T, Gilbert F, Zalat S, El-Gabbas A, Reader T. Climate-based models of spatial patterns of species richness in Egypt’s butterfly and mammal fauna. J Biogeogr. 2009;36:2085–95. doi:10.1111/j.1365-2699.2009.02140.x.
- Leathwick J, Moilanen A, Francis M, Elith J, Taylor P, Julian K, et al. Novel methods for the design and evaluation of marine protected areas in offshore waters. Conservation Letters. 2008;1:91–102. doi:10.1111/j.1755-263X.2008.00012.x.
- Gordon A, Simondson D, White M, Moilanen A, Bekessy SA. Integrating conservation planning and landuse planning in urban landscapes. Landscape Urban Plan (Landscape & Urban Planning). 2009;91:183–94. doi:10.1016/j.landurbplan.2008.12.011.
- Moilanen A, Anderson BJ, Arponen A, Pouzols FM, Thomas CD. Edge artefacts and lost performance in national versus continental conservation priority areas. Divers Distrib. 2013;19:171–83. doi:10.1111/Ddi.12000.
- El-Gabbas A, Dormann CF. Wrong, but useful: regional species distribution models may not be improved by range-wide data under biased sampling. Ecol Evol (Ecology & Evolution). 2018;8:2196–206. doi:10.1002/ece3.3834.
- Grand J, Cummings MP, Rebelo TG, Ricketts TH, Neel MC. Biased data reduce efficiency and effectiveness of conservation reserve networks. Ecol. Lett. 2007;10:364–74. doi:10.1111/j.1461-0248.2007.01025.x.
- Mikkonen N, Moilanen A. Identification of top priority areas and management landscapes from a national Natura 2000 network. Environmental Science & Policy. 2013;27:11–20. doi:10.1016/j.envsci.2012.10.022.
- Baha El Din S. Towards establishing a network plan for protected areas in Egypt. EEAA, Cairo.; 1998.
- Kaky E, Gilbert F. Using species distribution models to assess the importance of Egypt’s protected areas for the conservation of medicinal plants. Journal of Arid Environments. 2016;135:140–6. doi:10.1016/j.jaridenv.2016.09.001.
- Kaky E, Gilbert F. Predicting the distributions of Egypt’s medicinal plants and their potential shifts under future climate change. PLoS One. 2017;12:e0187714. doi:10.1371/journal.pone.0187714.
- Yang W, Ma K, Kreft H. Geographical sampling bias in a large distributional database and its effects on species richness–environment models. J Biogeogr. 2013;40:1415–26. doi:10.1111/jbi.12108.
- Brooks TM, Mittermeier RA, da Fonseca GAB, Gerlach J, Hoffmann M, Lamoreux JF, et al. Global biodiversity conservation priorities. Science. 2006;313:58–61. doi:10.1126/science.1127609.
- Carroll C, Dunk JR, Moilanen A. Optimizing resiliency of reserve networks to climate change. Global Change Biol. 2010;16:891–904. doi:10.1111/j.1365-2486.2009.01965.x.
- Leach K, Zalat S, Gilbert F. Egypt’s protected area network under future climate change. Biol Conserv. 2013;159:490–500. doi:10.1016/j.biocon.2012.11.025.
- Kujala H, Moilanen A, Gordon A. Spatial characteristics of species distributions as drivers in conservation prioritization. Methods Ecol Evol. 2018;9:1121–32. doi:10.1111/2041-210X.12939.
Figure 1: Permutation importance of factors affecting Zonation sensitivity across 2,560 option combinations (randomForest model). The dependent variable is the mean species representation in the top 17% priority cells. (For the top 10% and 25%, see Fig. S5.) Statistical interactions are included in the measure of variable importance. Figure 2: The spatial distribution of the top 17% priority cells for different connectivity options (using options CAZ, bias-free predictions, all study species, Maxent). The top-left map shows the pattern of important sites without connectivity integration. The second to fourth columns are for equivalent maps with steeper response curves (low, medium, and high connectivity; curves 2-4 in Fig. S3, respectively); while rows are for different numbers of effective neighbour cells (1 to 3). (Equivalent maps using ABF are shown in Fig. S4.). Figure 3: The spatial distribution of the top 12% priority cells (the darker, the higher the priority) for the four surrogates using core-area zonation, overlaid with current protected areas in Egypt (blue borders) (using bias-free predictions from Maxent). Equivalent maps using additive-benefit function are shown in Fig. S7.). Figure 4: The fraction of cells protected per Zonation rank for different surrogates using core-area zonation (using bias-free predictions from Maxent). The number on each panel represents Kendall’s correlation coefficient. (For equivalent results using additive-benefit function or elastic net, see Figs. S8-9). Figure 5: Performance curves for Zonation analyses (Maxent, CAZ, all species together). Left panel is without PA integration. Solid curves represent the average performance curve for all species or per species-group; while dashed lines represent the overall minimum and maximum performance curve per species group. The vertical grey line is for urban and agricultural areas (Fig. S2) forced to have low priority value; while the dashed vertical green line represents top priority sites existent in an area equals to the area covered by PAs. The right panel represents equivalent analysis with Egyptian PAs forced to have the highest priority scores. The vertical green line represents the area covered by PAs; while the vertical orange line represents the proposed areas for PAs expansion to 17% of Egypt. Performance curves are described in Fig. S1 and in the main text. For equivalent curves using ABF, see Fig. S10. Results for elastic net are shown in Fig. S11. Figure 6: The overall pattern of top priority sites using CAZ (left) or ABF (right), both using bias-free predictions from Maxent. Each map shows the summed rankings of the top 17% sites from the four surrogates used. Shading within current PAs (blue borders) are not shown to highlight the pattern for PA expansion (darker shading indicates higher rankings). The pattern for each species group is shown in Fig. S12. For equivalent maps using elastic net, see Fig. S13.
Supporting Information for “Spatial conservation prioritisation in data-poor countries: a quantitative sensitivity analysis using multiple taxa” – BMC Ecology Ahmed El-Gabbas1*, Francis Gilbert2, Carsten F. Dormann1 1 Department of Biometry and Environmental System Analysis, University of Freiburg, D-79106 Freiburg, Germany 2 School of Life Sciences, University of Nottingham, Nottingham, United Kingdom ∗ To whom correspondence should be addressed; E-mail: elgabbas@outlook.com
Table S1: List of species used or excluded in this study. RL represents per-species national Red List status; while W represent the assigned weight
(A) Study species
Species RL W
Butterflies
1 Agrodiaetus loewii VU 3 2 Apharitis acamas VU 3 3 Azanus ubaldus LC 1 4 Belenois aurota NA 1 5 Borbo borbonica NA 1 6 Catopsilia florella NA 1 7 Chilades trochylus LC 1 8 Colias croceus NA 1 9 Colotis fausta VU 3 10 Danaus chrysippus LC 1 11 Deudorix livia LC 1 12 Euchloe aegyptiaca EN 4 13 Euchloe belemia DD 1 14 Gegenes nostrodamus LC 1 15 Hypolimnas misippus NA 1 16 Iolana alfierii LC 1 17 Junonia hierta LC 1 18 Lampides boeticus LC 1 19 Leptotes pirithous LC 1 20 Lycaena phlaeas NA 1 21 Melitaea deserticola VU 3 22 Papilio saharae VU 3 23 Pelopidas thrax LC 1 24 Pieris rapae LC 1 25 Plebejus philbyi VU 3 26 Pontia daplidice LC 1 27 Pontia glauconome LC 1 28 Spialia doris LC 1 29 Tarucus rosaceus LC 1 30 Vanessa atalanta NA 1 31 Vanessa cardui NA 1 32 Zizeeria karsandra LC 1
Reptiles
33 Acanthodactylus aegyptius LC 1 34 Acanthodactylus boskianus LC 1 35 Acanthodactylus longipes VU 3 36 Acanthodactylus pardalis VU 3 37 Acanthodactylus scutellatus LC 1 38 Agama spinosa LC 1 39 Cerastes cerastes LC 1 40 Cerastes vipera LC 1 41 Chalcides cf. humilis LC 1 42 Chalcides ocellatus LC 1 43 Chamaeleo africanus EN 4 44 Chamaeleo chamaeleon LC 1 45 Cyrtopodion scabrum LC 1 46 Echis coloratus LC 1 47 Echis pyramidum LC 1 48 Eirenis coronella VU 3 49 Eryx colubrinus VU 3 50 Eryx jaculus LC 1 51 Eumeces schneiderii LC 1 52 Hemidactylus flaviviridis VU 3 53 Hemidactylus robustus VU 3 54 Hemidactylus turcicus LC 1 55 Laudakia stellio LC 1 56 Leptotyphlops cairi EN 4 57 Lytorhynchus diadema LC 1 58 Macroprotodon cucullatus VU 3 59 Malpolon moilensis LC 1 60 Malpolon monspessulanus LC 1 61 Mesalina bahaeldini VU 3 62 Mesalina guttulata LC 1 63 Mesalina olivieri LC 1 64 Mesalina pasteuri VU 3 65 Mesalina rubropunctata LC 1 66 Naja haje LC 1 67 Naja nubiae VU 3 68 Natrix tessellata VU 3 69 Ophisops occidentalis VU 3 70 Platyceps florulentus LC 1 71 Platyceps rogersi LC 1 72 Platyceps saharicus LC 1 73 Pristurus flavipunctatus VU 3 74 Psammophis aegyptius LC 1 75 Psammophis schokari LC 1 76 Psammophis sibilans LC 1 77 Pseudotrapelus sinaitus LC 1 78 Ptyodactylus guttatus LC 1 79 Ptyodactylus hasselquistii LC 1 80 Ptyodactylus siphonorhina LC 1 81 Ramphotyphlops braminus NA 1 82 Scincus scincus LC 1 83 Spalerosophis diadema LC 1 84 Sphenops sepsoides LC 1 85 Stenodactylus mauritanicus VU 3 86 Stenodactylus petrii LC 1 87 Stenodactylus sthenodactylus LC 1 88 Tarentola annularis LC 1 89 Tarentola mauritanica LC 1 90 Tarentola mindiae VU 3 91 Telescopus dhara LC 1 92 Testudo kleinmanni VU 3 93 Trachylepis quinquetaeniata LC 1 94 Trachylepis vittata VU 3 95 Trapelus mutabilis LC 1 96 Trapelus pallidus LC 1 97 Trapelus savignii VU 3 98 Tropiocolotes bisharicus VU 3 99 Tropiocolotes nattereri LC 1 100 Tropiocolotes steudneri LC 1 101 Tropiocolotes tripolitanus LC 1 102 Uromastyx aegyptia LC 1 103 Uromastyx ocellata EN 4 104 Uromastyx ornata VU 3 105 Varanus griseus LC 1 106 Varanus niloticus VU 3 107 Walterinnesia aegyptia VU 3
Mammals
108 Hemiechinus auritus LC 1 109 Paraechinus aethiopicus LC 1 110 Crocidura olivieri VU 3 111 Rousettus aegyptiacus LC 1 112 Taphozous nudiventris VU 3 113 Taphozous perforatus LC 1 114 Asellia tridens LC 1 115 Rhinolophus clivosus LC 1 116 Rhinolophus mehelyi EN 4 117 Rhinopoma cystops LC 1 118 Rhinopoma microphyllum VU 3 119 Tadarida aegyptiaca VU 3 120 Tadarida teniotis VU 3 121 Nycteris thebaica LC 1 122 Eptesicus bottae VU 3 123 Hypsugo ariel VU 3 124 Otonycteris hemprichii LC 1 125 Pipistrellus kuhlii LC 1 126 Pipistrellus rueppellii VU 3 127 Plecotus christii LC 1 128 Lepus capensis LC 1 129 Allactaga tetradactyla EN 4 130 Jaculus jaculus LC 1 131 Jaculus orientalis VU 3 132 Eliomys melanurus EN 4 133 Acomys cahirinus LC 1 134 Acomys dimidiatus LC 1 135 Acomys russatus LC 1 136 Arvicanthis niloticus LC 1 137 Dipodillus campestris VU 3 138 Dipodillus dasyurus LC 1 139 Dipodillus mackilligini VU 3 140 Dipodillus simoni VU 3 141 Gerbillus amoenus LC 1 142 Gerbillus andersoni VU 3 143 Gerbillus floweri VU 3 144 Gerbillus gerbillus LC 1 145 Gerbillus henleyi LC 1 146 Gerbillus perpallidus LC 1 147 Gerbillus pyramidum LC 1 148 Meriones crassus LC 1 149 Meriones libycus LC 1 150 Meriones shawi EN 4 151 Mus musculus NA 1 152 Nesokia indica EN 4 153 Pachyuromys duprasi VU 3 154 Psammomys obesus LC 1 155 Rattus norvegicus NA 1 156 Rattus rattus NA 1 157 Sekeetamys calurus LC 1 158 Spalax aegyptiacus EN 4 159 Canis aureus DD 1 160 Vulpes rueppellii LC 1 161 Vulpes vulpes LC 1 162 Vulpes zerda EN 4 163 Acinonyx jubatus CR 5 164 Caracal caracal DD 1 165 Felis chaus LC 1 166 Felis margarita VU 3 167 Felis silvestris LC 1 168 Panthera pardus CR 5 169 Herpestes ichneumon LC 1 170 Hyaena hyaena LC 1 171 Ictonyx libyca EN 4 172 Mustela subpalmata VU 3 173 Procavia capensis LC 1 174 Equus asinus CR 5 175 Ammotragus lervia CR 5 176 Capra nubiana EN 4 177 Gazella dorcas VU 3 178 Gazella leptoceros EN 4
(B) Excluded species
Butterflies Azanus jesous Chilades eleusis Colotis phisadia Elphinstonia charlonia Lycaena thersamon Carcharodus alceae Carcharodus stauderi Colotis danae Pseudotergumia pisidice Anthene amarah Apharitis myrmecophila Calopieris eulimene Charaxes hansali Chazara persephone Colotis chrysonome Colotis liagore Euchloe falloui Gomalia elma Melitaea trivia Pieris brassicae Polyommatus icarus Pseudophilotes abencerragus Pseudophilotes sinaicus Sarangesa phidyle Satyrium jebelia Tomares ballus Zegris eupheme Zizina otis
Reptiles Ablepharus rueppellii Atractaspis engaddensis Dasypeltis scabra Dolichophis jugularis Hemidactylus foudaii Hemidactylus mindiae Hemidactylus sinaitus Hemorrohis algirus Hemorrohis nummifer Latastia longicaudata Leptotyphlops macrorhynchus Leptotyphlops nursii Lycophidion capense Mesalina brevirostris Mesalina martini Ophisops elbaensis Ophisops elegans Philochortus zolii Platyceps sinai Psammophis punctulatus Pseuderemias mucronata Pseudocerastes persicus Ptyodactylus ragazzi Rhynchocalamus melanocephalus Telescopus hoogstraali Tropiocolotes nubicus Typhlops vermicularis
Mammals Crocidura floweri Crocidura suaveolens Pipistrellus deserti Crocidura religiosa Rhinolophus hipposideros Atelerix algirus Suncus murinus Barbastella leucomelas Nycticeinops schlieffeni Hystrix cristata Hystrix indica Gerbillus nanus Meriones sacramenti Meriones tristrami Vulpes cana Proteles cristata Ictonyx striatus Meles meles Vormela peregusna Genetta genetta Gazella gazella
Table S2: Kendall’s correlation between priority rankings of different surrogate groups (bias-free predictions) using core-area zonation (light grey) and additive-benefit function (dark grey); using Maxent (A) and elastic net (B).
(A) Maxent
Butterflies Reptiles Mammals All species
Butterflies 0.28 0.21 0.30 Reptiles 0.47 0.40 0.49 Mammals 0.43 0.44 0.39 All species 0.41 0.49 0.44
(B) elastic net
Butterflies Reptiles Mammals All species
Butterflies 0.31 0.20 0.31 Reptiles 0.54 0.40 0.54 Mammals 0.38 0.48 0.43 All species 0.47 0.56 0.43
Figure S1: An example performance curve produced during the Zonation analysis. This curve quantifies the proportion of features’ original distribution remaining (here, 14 species; y-axis) at each top fraction of the cells (x-axis). The x-axis denotes the priority ranking of the solution, with the highest rank site has a value of one, while the lowest rank site has a value of zero. The per-species performance curve is shown as grey curves, with their overall average in blue. It is possible in some Zonation analyses to handle hundreds of species, and hence the per-species performance curve can be hard to interpret. The overall mean (or per taxonomic group) performance curve is more appropriate in many situations. It is possible for any percentage of the top (or low) priority fraction of the landscape to get representation level of each species. In this figure, the red area represents the top 17% priority cells. The minimum (1), maximum (2), and the overall mean (3) species representation are shown in the figure. Similarly, the green area represents the urban and agricultural areas in Egypt (Fig. S2.2) which were forced in our analyses to have the lowest priority ranking. The mean species representation in this area is also shown (4). For an example performance curve using the full species list, see Fig. 5.
Figure S2: Urban and agricultural areas masked during Zonation prioritisation. These areas have high human population density, more polluted, and are of exceptionally high economic value (replacement cost), and hence it is challenging to apply strict conservation actions or construct new PAs in these areas.
Figure S3: Response curves used in the boundary-quality penalty connectivity analyses. These curves describe a range of species sensitivity to habitat loss in neighbour cells, ranging from no response (1) to strong response (4). The x-axis shows the percentage of neighbour cells (specified by three values of radii) remaining: 100 represents no habitat loss; while 0 represents total habitat loss of all neighbour cells. The y-axis shows the percentage reduction in local cell value in response to habitat loss.
Figure S4: The spatial distribution of the top 17% priority cells at different options of connectivity (additive-benefit function; using bias-free predictions of all the study species for Maxent). The top-left map shows the pattern of important sites without connectivity integration. The second to the fourth column is for equivalent maps with steeper response curves (low, medium, and high connectivity; curves 2-4 in Fig. S2.3, respectively); while rows are for different number of effective neighbour cells used (1 to 3). Equivalent maps using core-area zonation are shown in Fig. 2.
Figure S5: Permutation importance of factors affecting the Zonation output’s uncertainty, calculated from a randomForest model (varimp function using party package). Here, the dependent variable is the mean species representation at the top 10% (above) and 25% (below) priority cells.
Figure S6: The relative importance of various factors affecting the uncertainty of Zonation’s prioritisation (and their first order interactions) using the mean sum of squares from a generalised linear model. Only variables having more than 1% importance are shown.
Figure S7: The spatial distribution of the top priority cells of equal area to current PAs (the darker, the higher the priority) for different surrogates using additive-benefit function, overlaid with current protected areas in Egypt (blue borders). These maps were prepared using bias-free predictions from Maxent. Equivalent maps using core-area zonation are shown in Fig. 3.
Figure S8: The fraction of cells protected at each 1% intervals of Zonation rank for different surrogates using additive-benefit function (using bias-free predictions from Maxent). The number at each panel represents Kendall’s correlation coefficient. Equivalent results for core-area zonation are shown in Fig. 4; while results using elastic net are shown in Fig. S2.9.
Figure S9: The fraction of cells protected at each 1% intervals of Zonation rank for different surrogates using core-area zonation (top row) or additive-benefit function (bottom row), using bias-free predictions for elastic net. The numbers at each panel represent Kendall’s correlation coefficient.
Figure S10: Performance curves for Zonation analyses (Maxent – additive-benefit function – all species together). Left panel represents analysis without Egyptian PAs integration. Solid curves represent the average performance curve for all species or per species-group; while dashed lines represent the overall minimum and maximum performance curves per species group. The vertical grey line is for urban and agricultural areas (Fig. S2.2) forced to have low priority value; while the dashed vertical green line represents top priority sites existent in an area equals to the area covered by PAs. The right panel represents equivalent analysis with Egyptian PAs forced to have highest priority scores. The vertical green line represents the area covered by PAs; while the vertical orange line represents the proposed areas for PAs expansion to 17% of Egypt. For equivalent curves using core-area zonation, see Fig. 5. Results for elastic net are shown in Fig. S2.11.
Figure S11: Performance curves for elastic-net models using core-area zonation (A) and additive benefit function (B). For more details, see Fig. 5 and main text.
Figure S12: Potential areas for Egyptian PAs expansion (to 17% of Egypt) using core-area zonation (above) and additive-benefit function (below) for each surrogate. All maps use bias-free predictions from Maxent. Egyptian PAs (shown in blue) were always forced to have the highest ranking. Grey areas outside PAs represent potential areas for Egyptian PAs expansion. The ranking of these maps are added together to summarise the overall pattern of important sites outside PAs (see Fig. 6, and similarly Fig. S2.13 for elastic net).
Figure S13: The overall pattern of top priority sites using core-area zonation (left) or additive-benefit function (right), both using bias-free predictions from elastic net. Each map shows the summed rankings of the top 17% sites from the four surrogates used. Top priority sites within current protected areas (blue borders) are not shown to highlight the overall pattern of potential areas for PAs expansion (the darker the colour, the higher the cumulative rank of the site using the four surrogates). For equivalent maps using Maxent, see Fig. 6.
Figure S14: Boxplots for the predictive consistency of cross-validated models with (left) and without (right) correction for sampling bias. Predictions from Maxent are shown in white boxes, while elastic-net is shown in grey boxes. The median predictive consistency is about 0.8, with relatively higher median consistency for elastic net (environment-only models). Predictive consistency was one method of weighting species in Zonation, see the main text for details.
No. Protectorate Name Declaration Date Area Km² Governorate 1 Ras Mohamed National Park 1983 850 South Sinai 2 Zaranik Protectorate 1985 230 North Sinai 3 Ahrash Protectorate 1985 8 North Sinai 4 El-Omayed Protectorate 1986 700 Matrouh 5 Elba National Park 1986 35600 Red Sea 6 Saluga and Ghazal Protectorate 1986 0.5 Aswan 7 St. Katherine National Park 1988 4250 South Sinai 8 Ashtum El-Gamil Protectorate 1988 180 Port Said 9 Lake Qarun Protectorate 1989 250 El Fayoum 10 Wadi El-Rayan Protectorate 1989 1225 El Fayoum 11 Wadi Allaqi Protectorate 1989 30000 Aswan 12 Wadi El-Assuti Protectorate 1989 35 Assuit 13 El Hassana Dome Protectorate 1989 1 Giza 14 Petrified Forest Protectorate 1989 7 Cairo 15 Sannur Cave Protectorate 1992 12 Beni Suef 16 Nabq Protectorate 1992 600 South Sinai 17 Abu Galum Protectorate 1992 500 South Sinai 18 Taba Protectorate 1998 3595 South Sinai 19 Lake Burullus Protectorate 1998 460 Kafr El Sheikh 20 Nile Islands Protectorates 1998 160 All Governorates on the Nile 21 Wadi Degla Protectorate 1999 60 Cairo 22 Siwa 2002 7800 Matrouh 23 White Desert 2002 3010 Matrouh 24 Wadi El-Gemal/Hamata 2003 7450 Red Sea 25 Red Sea Northern Islands 2006 1991 Red Sea 26 El-Gilf El-Kebir 2007 48523 New Valley 27 El-Dababya 2007 1 Qena 28 El-Salum Gulf 2010 383 Matrouh 29 El-Wahat El-Bahreya 2010 109 6th October 30 Mount Kamel Meteor Protectorate 2012 1 New Valley
Figure S15: Current Egyptian protected areas.
Figure S16: Field evaluation sites visited during protected areas identification mission between December 1996 and April 1998. (Sherif Baha El Din, pers. comm.).
Figure S17: The frequency with which each cell was located at the top or lowest priority sites across all prioritization analyses performed. For each of the 2,560 Zonation analysis, the top 17% (above) or lowest 30% (below) priority sites were determined and the number of times each cell was chosen is reported, either with forcing PAs to have highest scores (left) or not (right). Only cells chosen in more than 500 zonation solutions are shown. Appendix S1: Data sources List of literature resources used for extracting Egyptian records. ACR (2015) African Chiroptera Report. 2015. AfricanBats, Pretoria, i - xix, 1 - 7001 pp. Alfieri A. (1942) Quatre lépidoptères nouveaux pour la faune du Sinai. Bulletin de la Société Fouad 1er Entomologie, 26: 163-166. Al-Hussaini A.H. (1959) The vertebrate fauna of the Bahariya Oasis (excluding fishes and mammals). Ain Shams Science Bull., 4:103-107. Ali R.A. (2011) Molecular Phylogenetic Relationship Between and Within the Fruit Bat (Rousettus aegyptiacus) and the Lesser Tailed Bat (Rhinopoma hardwickei) Deduced From RAPD-PCR Analysis. Journal of American Science, 7(10):678-687. Andersen K. (1906) On some new or little-known bats of the genus Rhinolophus in the collection of the Museo Civico, Genoa. Annali del Musei Civico di Storia Naturale di Genova, (3a): 173-195. Anderson J. (1902) Zoology of Egypt: Mammalia (compiled by W.E. de Winton). London: Hugh Rees Ltd. Andres A. & Seitz A. (1925) Die Lepidopteren-Fauna Aegyptens. Nachtrag zum ersten Teil. Senckenbergiana, 7(1/2): 54-61. Andres A. (1912) Verzeichnis der bis jetzt in Aegypten beobachten Schmetterlinge. Bulletin de la Société entomologiques d’Egypte, 5: 53-114. Awadallah A.M., Azab A.K. & El Nahal A.K.M. (1970) Studies on the pomegranate butterfly, Virachola livia (Klug). Bulletin de la Société entomologiques d’Egypte, 54: 545-567. Badir M. A. & EL-Nagar M. H. (1994) Some ecological studies on the fauna of Saint Kathrine area in South Sinai with special reference to the Agamid lizard Agama stellio. J. Egypt Ger. Soc. Zool., 13 (A), 67:84. Baha El Din S. (2006) A guide to the reptiles and amphibians of Egypt. The American University in Cairo Press, Cairo. Baha El Din S.M. (1999) A new species of Tropiocolotes (Reptilia: Gekkonidae) from Egypt. Zoology in the Middle East, 19: 17-26. Baha El Din S.M. (2001) A synopsis of African and south Arabian geckos of the genus Tropiocolotes (Reptilia: Gekkonidae), with a description of a new species from Egypt. Zoology in the Middle East, 22: 45-56. Baha El Din S.M. (2003) A new species of Hemidactylus (Squamata: Gekkonidae) from Egypt. African Journal of Herpetology, 52: 39-47. Baha El Din S.M. (2005) An overview of Egyptian species of Hemidactylus (Gekkonidae), with the description of a new species from the high mountains of South Sinai. Zoology in the Middle East, 34: 11-26. Baha El Din S.M. (2011) Distribution and recent range extension of Natrix tessellata in Egypt. Mertensiella, 18: 401-403. Baharav D. & Meiboom U. (1981) The status of the Nubian ibex Capra ibex nubiana in the Sinai desert. Biological Conservation, 20(2): 91-97. Bajer A., Harris P.D., Behnke J.M., et al. (2006) Local variation of haemoparasites and arthropod vectors, and intestinal protozoans in spiny mice (Acomys dimidiatus) from four montane wadis in the St Katherine Protectorate, Sinai, Egypt. Journal of Zoology, 270: 9-24. Barnard C.J., Sayed E., et al. (2003) Local variation in helminth burdens of Egyptian spiny mice (Acomys cahirinus dimidiatus) from ecologically similar sites: relationships with hormone concentrations and social behaviour. Journal of Helminthology, 77(3):197-207. Basuony M.I. & Saleh M.A. (2005) Biodiversity of the southern sector of the Egyptian Eastern Desert. Egyptian Journal of Zoology, 44: 99-123. Basuony M.I. (2003) Ecological distribution and zoogeographical relationships of mammalian assemblages in two wetland areas in northern Egypt. Egyptian Journal of Zoology, 40: 575-599. Basuony M.I. (2004) New distributional records of the shrews Crocidura nana Dobson, 1890 and Suncus murinus (Linnaeus, 1766) from Egypt. Al-Azhar Bulletin of Science, 15(2): 11-17. Basuony M.I. (2013) Food and feeding ecology of the Egyptian Mongoose, Herpestes ichneumon (Linnaeus, 1758) in Egypt. Journal of Applied Sciences Research, 9(11): 5811-5816. Basuony M.I., Gilbert F. & Zalat S. (2010). Mammals of Egypt: atlas, red data listing & conservation. BioMAP & CultNat, EEAA & Bibliotheca Alexandrina, Cairo. 286 pp. Bedir M.A., El-Naggar M.H., Kayed A.N. & Orabi G.M. (1999) Ecological studies on two lizard species inhabiting Wadi El-Arbein at Saint Katherine Area, South Sinai with special reference to biotic elements. J. Egypt. Ger. Soc. Zool., 28(A): 485-503. Benda P., Abi-Said M., Bartonička T., Bilgin R., Faizolahi K., Lučan R.K., Nicolaou H., Reiter A., Shohdi W.M., Uhrin M., Horáček I. (2011) Rousettus aegyptiacus (Pteropodidae) in the Palaearctic: list of records and revision of the distribution range. Vespertilio, 15: 3–36. Benda P., Al-Juaid M.M., Reiter A., Nasher A.K. (2011) Noteworthy records of bats from Yemen with description of a new species from Socotra. Hystrix, 22(1):23–56. Benda P., Andreas M., Kock D., Lučan R., Munclinger P., Nová P., Obuch J., Ochman K., Reiter A., Uhrin M., Weinfurtová D. (2006) Bats (Mammalia: Chiroptera) of the Eastern Mediterranean. Part 4. Bat fauna of Syria: distribution, systematics, ecology. Acta Societatis Zoologica Bohemicae, 70:1–329. Benda P., Andriollo T., Ruedi M. (2014) Systematic position and taxonomy of Pipistrellus deserti (Chiroptera: Vespertilionidae). Mammalia, 79(4):419-438. Benda P., Červený J., Konečný A., Reiter A., Ševčík M., Uhrin M., Vallo P. (2010) Some new records of bats from Morocco (Chiroptera). Lynx, 41:151-166. Benda P., Dietz C., Andreas M., Hotový J., Lučan R., Maltby A., Meakin K., Truscott J., Vallo P. (2008) Bats (Mammalia: Chiroptera) of the Eastern Mediterranean and Middle East. Part 6. Bats of Sinai (Egypt) with some taxonomic, ecological and echolocation data on that fauna. Acta Societatis Zoologicae Bohemicae, 72(1-2):1-103. Benda P., Faizolâhi K., Andreas M., Obuch J., Reiter A., Ševčík M., Uhrin M., Vallo P., Ashrafi S. (2012) Bats (Mammalia: Chiroptera) of the Eastern Mediterranean and Middle East. Part 10. Bat fauna of Iran. Acta Societatis Zoologicae Bohemicae, 76:163–582. Benda P., Gvoždík V. (2010) Taxonomy of the genus Otonycteris (Chiroptera: Vespertilionidae: Plecotini) as inferred from morphological and mtDNA data. Acta Chiropterologica, 12(1):83-102. Benda P., Hanák V., Andreas M., Reiter A., Uhrin M. (2004) Two new species of bats (Chiroptera) for the fauna of Libya: Rhinopoma hardwickii and Pipistrellus rueppellii. Myotis, 41–42: 109-124. Benda P., Hanák V., Červený J. (2011) Bats (Mammalia: Chiroptera) of the Eastern Mediterranean and Middle East. Part 9. Bats from Transcaucasia and West Turkestan in collection of the National Museum, Prague. Acta Societatis Zoologicae Bohemicae, 75:159–222. Benda P., Kiefer A., Hanák V., Veith M. (2004) Systematic status of African populations of long-eared bats, genus Plecotus (Mammalia: Chiroptera). Folia Zoologica, 53(1):1–48. Benda P., Lučan R. K., Shohdi W. M., Porteš M., Horáček I. (2014) Microbats of the Western Oases of Egypt, Libyan Desert. Vespertilio, 17:45–58. Benda P., Lučan R.K., Obuch J., Reiter A., Andreas M., Bačkor P., Bohnenstengel T., Eid E.K., Ševčík M., Vallo P., et al. (2010) Bats (Mammalia: Chiroptera) of the Eastern Mediterranean and Middle East. Part 8. Bats of Jordan: fauna, ecology, echolocation, ectoparasites. Acta Societas Zoologicae Bohemicae, 74:185–353. Benda P., Reiter A., Al-Jumaily M., Nasher A.-K., Hulva P. (2009) A new species of mouse-tailed bat (Chiroptera: Rhinopomatidae: Rhinopoma) from Yemen. Journal of the National Museum (Prague), Natural History Series, 177 (6):53–68. Benda P., Ruedi M. (2004) New data on the distribution of bats (Chiroptera) in Morocco. Lynx, 35:13-44. Benda P., Spitzenberger F., Hanák V., Andreas M., Reiter A., Ševčík M., Šmíd J., Uhrin M. (2014) Bats (Mammalia: Chiroptera) of the Eastern Mediterranean and Middle East. Part 11. On the bat fauna of Libya II. Acta Societatis Zoologicae Bohemicae, 78:1–162. Benda P., Vallo P. (2012) New look on the geographical variation in Rhinolophus clivosus with description of a new horseshoe bat species from Cyrenaica, Libya. Vespertilio, 16: 69-96. Benda P., Vallo P., Hulva P., Horáček I. (2012) The Egyptian fruit bat Rousettus aegyptiacus (Chiroptera: Pteropodidae) in the Palaearctic: geographical variation and taxonomic status. Biologia, 67(6):1230–1244. Benda P., Vallo P., Reiter A. (2011) Taxonomic revision of the genus Asellia (Chiroptera: Hipposideridae) with a description of a new species from Southern Arabia. Acta Chiropterol, 13(2):245–270. Benyamini D. (1984) The butterflies of the Sinai Peninsula (Lep. Rhopalocera). Nota Lepidoptera, 7(4): 309-321. Benyamini D. (1999) The biology and conservation of Iolana alfierii Wiltshire, 1948: the Burning Bush Blue (Lepidoptera: Lycaenidae). Linneana Belgica, 17(4): 119-134. Bergmans W. (1994) Taxonomy and biogeography of African fruit bats (Mammalia, Megachiroptera). 4. The genus Rousettus Gray, 1821. Beaufortia, 44:79-126. Bonhote J.L. (1909) On a small collection of mammals from Egypt. Proceedings of the Zoological Society of London, 79(4)788-802. Boyd A.W. (1917) Six month’s collecting between Ismailia and El Arish. Bulletin de la Société entomologiques d’Egypte, 5: 98-119. Churcher C.S. (1991) The Egyptian fruit bat Rousettus aegyptiacus in Dakhleh Oasis, Western Desert of Egypt. Mammalia, 551:139-143. DeBlase A. (1972) Rhinolophus euryale and R. mehelyi (Chiroptera: Rhinolophidae) in Egypt and Southwest Asia. Israel Journal of Zoology, 21(1):1-12. Debski B. (1919) Quelques observations sur Teracolus fausta, Melitaea didyma et Azanus ubaldus. Bulletin de la Société entomologique de France, 1919: 33-43. Demaison L. (1895) Note sur les Lépidoptères d’Egypte. Bulletin de la Société entomologique de France, 1895: 51-62. Dietz C. (2005) Bats Final Report - Operation Wallacea. Sinai 2005. 23 pp. Dornburg A., Colosi J.G., Maser C., Reesel A.T., Watkins-Colwell G.J. (2011) A survey of the Yale Peabody Museum collection of Egyptian mammals collected during construction of the Aswan high dam, with an emphasis on material from the 1962–1965 Yale University prehistoric expedition to Nubia. Bulletin of the Peabody Museum of Natural History, 52(2):255-272. El-Gabbas A. & Gilbert F. (2016) The Desert Beauty Calopieris eulimene: a butterfly new to Egypt (Insecta: Lepidoptera). Zoology in the Middle East, 62(3): 279–281. Farid M. (1977) On some fishes, Amphibia, and reptilia from Siwa Oasis. Proc. Zool. Soc. A.R.E, 6: 234-231. Fathy W. (2006) Studies of the genus Vulpes in Egypt. PhD Thesis, Faculty of Education, Ain Shams University, Cairo. Ferguson W.W. (1981) The systematic position of Canis aureus lupaster (Carnivora: Canidae) and the occurrence of Canis lupus in North Africa, Egypt and Sinai. Mammalia, 45(4): 459–466. Ferguson W.W. (1981) The systematic position of Gazella dorcas (Artiodactyla: Bovidae) in Israel and Sinai. Mammalia, 45(4): 453-458. Flower S.S. (1932) Notes on the recent mammals of Egypt, with a list of the species recorded from that kingdom. Proceedings of the Zoological Society of London 1932, 369-450. Foui E. (2011) Operation Wallacea: Sinai bats report. Available at: https://opwall.com/wp-content/uploads/OpWall_Sinai-bat-report_2011.pdf Fowler H.W. (1914) Fishes and Reptiles from Assuan, Egypt. Copeia, 8: 1-2. Freeman P.W. (1981) A multivariate study of the family Molossidae (Mammalia, Chiroptera): morphology, ecology, evolution. Fieldiana Zoology, New Series No. 7, Field Museum of Natural History, Chicago, 173 pp. Gabriel A.G. & Steven-Corbet A. (1949) Results of the Armstrong College expedition to Siwa Oasis (Libyan desert), 1395, under the leadership of Prof. J Omer-Cooper. Bulletin de la Societe Fouad 1er d’Entomologie, 33: 373-9. Gaisler J., Madkour G. & Pelikan J. (1972) On the bats of Egypt. Acta Scientiarum Naturalium Academiae Scientarium Bohemoslovaceae Brno, 6(8):1-40. Ghazali O.F. & Baha El Din S. (2005) On the occurrence of Psammophis punctulatus Bibron & Dumeril 1854 in Egypt. Herpetological Bulletin, 94: 4-5. Ghobashi A.A., Abu Eglah M.H., Tantawy H.M. & Ibrahim A.A. (1990) Herpetofaunal survey of Al-Arish area (North Sinai) with special reference to their habitat and seasonal distribution. Proc. Zool. Soc. A.R.E, 21: 273-290. Gilbert, F. & Zalat, S. (2007). Butterflies of Egypt: atlas, red data listing & conservation. Egyptian Environmental Affairs Agency. Goodman S. M. & Helmy I. (1986) The sand cat Felis margarita Loche, 1858 in Egypt. Mammalia, 50:120-123. Graves P. (1915) A list of the butterflies of Egypt with some notes on those of the Sinai. Bulletin de la Société entomologiques d’Egypte, 4: 135-157. Graves P. (1918) Lampides ethoda, Wlk, and Lycaena itea, Wlk = Lampides thebana, Stdgr., and Azanus ubaldus, Cr. Entomologist, 51: 98-100. Graves P. (1918) Some new forms of Lycaenidae from Egypt. Entomologist, 51: 97-98. Harrison D.L. (1961) On Savi’s Pipistrelle, (Pipistrellus savii Bonaparte, 1837) in the Middle East, and a second record of Nycticeius schlieffeni Peters 1859 from Egypt. Senckenbergiana Biologica, 42 (1/2): 41-44. Harrison D.L. (1964) The Mammals of Arabia. Vol 1. Introduction, Insectivora, Chiroptera, Primates. pp 192. Benn, London, 1st edition. Harrison D.L., Bates P.J.J. (1991) The mammals of Arabia. 2nd edition. Harrison Zoological Museum Publication. Harrison D.L., Makin D. (1988) Significant new records of Vespertilionid Bats (Chiroptera: Vespertilionidae) from Israel. Mammalia, 52(4):593–596. Hart H.C. (1891) Some account of the flora and fauna of Sinai, Petra, and Wadi ‘Arabah. Committee of the Palestine Exploration Fund, London. Hassan M.M. & Fadl H.H. (2000) Contribution to the insect fauna of Gabal Elba and the Red Sea coast. Bull. Ent. Soc. Egypt, 78: 145-175. Hayman R.W. (1948) The Armstrong College Zoological Expedition to Siwa Oasis (Libyan Desert) 1935: Mammalia. Proceedings of the Egyptian Academy of Sciences, 4:38–42. Hill J.E. & Harrison D.L. (1987) The baculum in the Vespertilioninae (Chiroptera: Vespertilionidae) with a systematic review, a synopsis of Pipistrellus and Eptesicus, and the descriptions of a new genus and subgenus. Bulletin of the British Museum (Natural History) Zoology, 52:225-305. Hoogstraal H. & Traub R. (1963) The fleas (Siphonaptera) of Egypt. Host-parasite relationships of Insectivora and Chiroptera. Journal of the Egyptian Public Health Association, 38(3):111-130. Hoogstraal H. (1962) A brief review of the contemporary land mammals of Egypt (including Sinai). 1. Insectivora and Chiroptera. Journal of the Egyptian Public Health Association, 37(4):143-162. Hoogstraal H. (1963) A brief review of the contemporary land mammals of Egypt (including Sinai). 2. Lagomorpha and Rodentia. Journal of the Egyptian Public Health Association, 38(1): 1-35. Hoogstraal H. (1964) A brief review of the contemporary land mammals of Egypt (including Sinai). 3. Carnivora, Hyracoidea, Perissodactyla and Artiodactyla. Journal of the Egyptian Public Health Association, 39(1): 205-239. Ibrahim A.A. (2000) A radiotelemetric study of the body temperature of Varanus griseus (Sauria - Varanidae) in Zaranik Protected Area, North Sinai, Egypt. Egyptian Journal of Biology, 2: 57-66. Ibrahim A.A. (2000) Geographic distribution: Hemidactyllls flaviviridis. Herpetological Review 31(3): 185. Ibrahim A.A. (2000) Geographic distribution: Tarentola annularis annularis. Herpetological Review 31(3): 185. Ibrahim A.A. (2001) A Note on the Diet of the White-spotted gecko, Tarentola a. annularis. Gecko, 2: 6. Ibrahim A.A. (2001) Geographic distribution: Bufo regularis. Herpetological Review 32(2): 112. Ibrahim A.A. (2001) Geographic distribution: Coluber florulentus florulentus. Herpetological Review, 32(1): 59. Ibrahim A.A. (2001) Geographic distribution: Malpolon monspessulanus insignitus. Herpetological review: 32(2): 123. Ibrahim A.A. (2001) Geographic distribution: Ptychadena mascareniensis. Herpetological Review 32(2): 115. Ibrahim A.A. (2001) Geographic distribution: Ptyodactylus h. hasselquistii. Herpetological Review 32(2): 120. Ibrahim A.A. (2002) Activity area, movement patterns, and habitat use of the desert monitor, Varanus griseus, in the Zaranik Protected Area, North Sinai, Egypt. African Journal of Herpetology, 51(1): 35-45. Ibrahim A.A. (2002) Activity patterns and microhabitat use of the Starred agama Laudakia stellio (Linnaeus, 1758) in AL-Arish city, North Sinai, Egypt. Egypt. J. zool., 39, 173-196. Ibrahim A.A. (2002) Geographic Distribution: Psammophis sibilans sibilans. Herpetological Review, 33: 69. Ibrahim A.A. (2003) Diet and reproduction of the Indian Leaf-toed gecko, Hemidactylus flaviviridis (Sauria: Gekkonidae), in Ismailia governorate, with consideration to its distribution in the Suez Canal area, Egypt. J. Union Arab Biol. Cairo, 19(A): 125-151. Ibrahim A.A. (2004) Behavioural ecology of the White-spotted Gecko, Tarentola annularis (Reptilia: Gekkonidae), in Ismailia City, Egypt. Zoology in the Middle East, 31: 23-38. Ibrahim A.A. (2007) Ecology of the Mediterranean Geko, Hemidactylus turcicus (Linnaeus, 1785) (Reptilia: Gekkonidae), in North Sinai, Egypt. Zoology in the Middle East, 41: 41-54. Ibrahim A.A. (2008) Amphibians and reptiles of the Suez Canal University campuses, Egypt. Herpetological Bulletin, 105: 1-9. Ibrahim A.A. (2012) New records of the Diced snake (Natrix tessellata), in the Suez Canal zone and Sinai. Amphibian and Reptile Conservation 6(2):2-4(e42). Ibrahim A.A. (2013) The Herpetology of the Suez Canal Zone, Egypt. Vertebrate Zoology, 63(1): 87-110. Ibrahim A.A., Saleh M.A., Dixon J.R. & Mahmoud I.M. (2000) On the ecology of the fringe-toed lizard, Acanthodactylus scutellatus (Sauria: Lacertidae) in North Sinai, Egypt. J. Egypt. Ger. Soc. Zool., 32 (A): 335-355. James M., Gilbert F. & Zalat S. (2003) Thyme and isolation for the Sinai Baton Blue butterfly (Pseudophilotes sinaicus). Oecologia, 134: 445-453. Kamal A., Soliman M. & EL-Assy Y. (1966) New record of the Amphibians and reptiles of some districts of the western Egyptian desert. Bulletin de l’instiude du deser d’egypte, 16: 145-157. Kamal A., Soliman M. & EL-Assy Y. (1966) New record of the Amphibians and reptiles of two other districts of the western Egyptian desert. Bulletin de l’instiude du deser d’egypte, 16: 159-167. Kark S., Warburg I. & Werner Y.L. (1997) Polymorphism in the snake Psammophis schokari on both sides of the desert edge in Israel and Sinai. Journal of Arid Environments, 37: 513-527. Kiefer A. (2007) Phylogeny of Western Palaearctic long-eared bats (Mammalia, Chiroptera, Plecotus): a molecular perspective. PhD thesis, Johannes Gutenberg-Universität in Mainz. Kneucker A. (1903) Zoologische Ausbeute einer botanischer Studienreise durch die Sinai-Halbinsel im März und April 1902. Verhandlungen der zoologischer-botanischer Gesellschaft im Wien, 53: 575-587. Kock D. (1969) Die Fledermaus-Fauna des Sudan. Abhandlungen der Senckenbergischen Naturforschenden Gesellschaft, Band 521:1:238. Kock D., Al-Jumaily M. & Nasher A.K. (2002) Horseshoe bats, genus Rhinolophus Lacépède, 1799 (Mammalia: Chiroptera: Rhinolophidae), of Yemen. Fauna of Arabia, 19:507–515. Koopman K.F. (1975) Bats of the Sudan. Bulletin of the American Museum of Natural History, 154(4). Lachman E., Carmely H. & Werner Y.L. (2006) Subspeciation befogged by the ‘‘Seligmann effect’’: the case of Laudakia stellio (Reptilia: Sauria: Agamidae) in southern Sinai, Egypt. Journal of Natural History, 40(19–20): 1259–1284 Larsen T.B. (1990) The butterflies of Egypt. Apollo Books, Svenborg, Denmark. Lewandowski S. & LewandowskiKrenz K. (2014) Beitrag zur Lepidopterenfauna von Ägypten (Lepidoptera), Teil 1: Familien Hesperiidae, Pieridae, Lycaenidae, Nymphalidae, Sphingidae, Lasiocampidae; sowie Erebidae: Unterfamilien Lymantriinae und Arctiinae. Nachrichten des Entomologischen Vereins Apollo, 34: 175-184. Lučan R.K., Šálek M. (2013) Observation of successful mobbing of an insectivorous bat, Taphozous nudiventris (Emballonuridae), on an avian predator, Tyto alba (Tytonidae). Mammalia, 77(2):235-236. Macy R.W., Heyneman D., Kuntz R.E. (1961) Records of trematodes of the families Lecithodendriidae, Dicrocoeliidae, and Heterophyidae from Chiroptera collected in Egypt and Yemen, S. W. Arabia. Proc. Helminthol. Soc. Washington, 28:13-17. Madkour G. (1977) Further observations on bats (Chiroptera) of Egypt. Agricultural Research Review, 55(1):173–184. Madkour G. (1977) Rousettus aegyptiacus (Megachiroptera) as a fruit eating bat in A.R. EGYPT. Agricultural research review, 55(1):167-172. Madkour G. (1978) Significance of the distal part of the humerus in the identification of Egyptian bats. Zoologischer Anzeiger, 201(5/6):387-390. Meakin K., de Kort S.R., Gilbert H., Gilbert F., et al. (2005) Monitoring birds, reptiles and butterflies in the St Katherine Protectorate, Egypt. Egyptian Journal of Biology, 7: 66-95. Milto K.D. (2017) New records of reptiles on the Red Sea Coast, Egypt, with notes on zoogeography. Russian Journal of Herpetology, 24(1):11-21. Nader I.A. & Kock D. (1983) Notes on some bats from the Near East (Mammalia: Chiroptera). Zeitschrift für Säugetierkunde, 48:1–9. Nader I.A. (1982) New distributional records of bats from the Kingdom of Saudi Arabia (Mammalia: Chiroptera). Journal of Zoology, 198(1):69-82. Nakamura I. & Benyamini D. (1973) Euchloe falloui Allard (Lepidoptera; Pieridae) from Egypt and the Sinai. Entomologist, 106: 267-8. Nakamura I. (1975) Descriptions of two new species of butterflies (Lepidoptera, Lycaenidae) from the South Sinai. Journal of Entomology (B), 44(3): 283-295. Nakamura I. (1977) On the identity of some of the Lycaenidae species (Lep.) described by Francis Walker (1870) from Egypt and the Sinai. Entomologist’s Gazette, 28: 9-14. Osborn D.J. & Helmy I. (1980) The contemporary land mammals of Egypt (including Sinai). Fieldiana Zoology, New Series, No 5: 1-579. Osborn D.J. (1988) New bat records from the Red Sea Mountains of Egypt. Mammalia, 52(4):596-598. Owen R.D., Qumsiyeh M.B. (1987) The subspecies problem in the Trident leaf-nosed bat, Asellia tridens: homomorphism in widely separated populations. Zeitschrift Für Saeugetierkunde, 6:329-337. Qumsiyeh M.B. (1985) The bats of Egypt. Special Publication of the Texas Technological University, 23: 1-102. Qumsiyeh M.B. (1996) Mammals of the Holy Land. Texas Tech University Press, Lubbock. Robbins L.W., Sarich V.M. (1988) Evolutionary relationships in the family Emballonuridae (Chiroptera). Journal of Mammalogy, 69(1):1-13. Rothschild C. (1901) Lepidoptera from Egypt and the Soudan. Novitates Zoologicae, V. 8. Saber S.S. (1994) Checklist of the Saurian fauna of Southern Sinai, Egypt. Al-Azhar Bull. of Science, 5(1): 183-201. Saber S.S. (1994) Herpetological survey of Suez province, Egypt. Bull. Biol. Soc. Egypt, 42. Saber S.S. (1994) Ophidian community of Sinai, Egypt. Al-Azhar Bull. of Science, 5(1): 203-217. Saleh M.A. & Basuony M.I. (1997) Ecological distribution of land mammals in South Sinai, Egypt. Al-Azhar Bulletin of Science, 8(2): 653-670. Saleh M.A. & Basuony M.I. (1998) A contribution to the mammalogy of the Sinai Peninsula. Mammalia, 62(4): 557-575. Saleh M.A. & Basuony M.I. (2005) The Zoril, Ictonyx striatus erythreae De Winton, 1898 in Egypt. Egyptian Journal of Biology, 7: 103-107. Saleh M.A. & Basuony M.I. (2014) Mammals of the Genus Canis Linnaeus, 1758 (Canidae, Carnivora) in Egypt. Egyptian Journal of Zoology, 62: 49-92. Saleh M.A. & Basuony M.I. (2014) New mammalian records from Egypt. Egyptian Journal of Zoology, 62: 111-130. Saleh M.A. (1987) The decline of gazelles in Egypt. Biological Conservation, 39: 83-95. Saleh M.A., Basuony M.I., Galhoum A. & Tolba M. (2003) Biodiversity and zoogeography of the Qattara Depression, Western Desert, Egypt. Egyptian Journal of Zoology, 40: 357-387. Saleh M.A., Helmy I. & Giegengack R. (2001) The cheetah, Acinonyx jubatus (Schreber, 1776) in Egypt (Felidae, Acinonychinae). Mammalia 65: 177-194. Sanborn C.C. & Hoogstraal H. (1955) The identification of Egyptian bats. Journal of the Egyptian Public Health Association, 30(4):103-119. Saoud M.F. & Ramadan M.M. (1977): On a new trematode, Prohemistomum azimi n. sp. (Trematoda: Cyathocotylidae) from the Egyptian slit-faced bat. Zeitschrift für Parasitenkunde, 53(3):281–285. Sayed M.T., Rostom Z.M.F. & Kaschef A.H. (1964) Contributions to the insect fauna of some oases of the Egyptian Western Desert. Bulletin de la Société entomologiques d’Egypte, 48: 259-267. Schmidt K.P. & Marx H. (1957) Results of the NAMRU-3 southeastern Egypt expedition, 1954. 2. Reptiles and amphibians. The Zoological Society of Egypt Bulletin, 13:16–28. Schwann H. (1905) A list of the mammals collected by the Hon. N. C. Rothschild, the Hon. F. R. Henley, and Mr. A. F. R. Wollaston in Egypt and the Soudan in January, February, and March 1904. Novitates Zoologicae, 12:1–5. Semida F.M. (1985) Survey and ecological studies of non-true pollinators of southern Sinai. MSc Thesis, Suez Canal University, Ismailia. Setzer H.W. (1952) Notes on mammals from the Nile Delta region of Egypt. Proceedings of the United States National Museum, 102(3305):343–369. Setzer H.W. (1955) Two new Jerboas from Egypt. Proceedings of The Biological Society of Washington, 68: 183–184. Setzer H.W. (1957) The hedgehogs and shrews (Insectivora) of Egypt. Journal of the Egyptian Public Health Association, 32(1): 1-17. Setzer H.W. (1958) The gerbils of Egypt. The Journal of the Egyptian Public Health Association, 23(6): 205-227. Setzer H.W. (1958) The Jerboas of Egypt. Journal of the Egyptian Public Health Association, 33(3): 87-94. Setzer H.W. (1958) The Mustelids of Egypt. The Journal of the Egyptian Public Health Association, 23(6): 199–204. Setzer H.W. (1959) The spiny mice (Acomys) of Egypt. The Journal of the Egyptian Public Health Association, 34(3): 93-101. Setzer H.W. (1961) The canids (Mammalia) of Egypt. Journal of the Egyptian Public Health Association, 36(3): 113-118. Setzer H.W. (1961) The jirds (Mammalia: Rodentia) of Egypt. Journal of the Egyptian Public Health Association, 36(3): 81-92. Setzer H.W. (1963) Notes on some Egyptian rodents. Journal of the Egyptian Public Health Association, 38(2): 51-60. Shahin A.A. & Ata A.T. (2004) C-banding Karyotype and Relationship of the Dipodids Allactaga and Jaculus (Mammalia: Rodentia) in Egypt. Folia biologica (Kraków), 52 (1-2): 25-31. Shehab A., Mamkhair I., Amr Z. (2006) First record of the Lesser Horseshoe bat, Rhinolophus hipposideros (Bechstein, 1800) (Rhinolophidae, Chiroptera) from Syria. Hystrix, the Italian Journal of Mammalogy, 17(2):161-166. Shifman S., Shacham B. & Werner Y.L. (1999) Tropioclotes nattereri (Reptilia, Gekkonidae) - comments on validity, variation and distribution. Zoology in the Middle East, 17: 51-66 Sohail S, Sameeh A.M. & Kareem M.S. (2014) Population studies on two sympatric bat species: Taphozous Perforatus and Rhinopoma Hardwickii (Chiroptera: Microchiroptera) from Egypt. Egyptian Journal of Zoology, 64: 13–31. Soliman S. & Mohallal E. (2009) Patterns of reproduction in two sympatric gerbil species in arid Egypt. Integrative Zoology, 4(2):248-253. Soliman S. & Mohallal E. (2014) A survey of the mammalian fauna of Siwa Oasis, Egypt. Egyptian Journal of Zoology, 61:171–186. Soultan A., Attum O., Hamada A., et al. (2017) Recent observation for leopard Panthera pardus in Egypt. Mammalia, 81(1): 115–117. Tawfik A. (1994) Taxonomic analysis of two chamaeleon species (Chamaeleontidae, Reptilia): morphological and biochemical studies. J. Egypt. Ger. Soc. Zool., 15(A): 191-203. Tolba M., Basuony M.I. & Khedr A. (2004) Ecosystem structure and sensitivity modeling of Hamata area, Eastern Desert, Egypt using GIS. Al-Azhar Bulletin of Science, 15(1): 55-82. Tregenza L. (2004) The Red Sea Mountains of Egypt and Egyptian Years. AUC Press, Cairo. Van Cakenberghe V., De Vree F. (1998) Systematics of African Nycteris (Mammalia: Chiroptera) Part III. The Nyteris thebaica group. Bonner Zoologische Beitrage, 48(2):123-166. Wacher T., Baha El Din S., Mikhail G. & Baha el Din M. (2002) New observations of the ’extinct’ Barbary sheep Ammotragus lervia ornata in Egypt. Oryx, 36(3): 301-304 Wagner P. & Böhme W. (2006) A new species of the genus Trapelus Cuvier, 1816 (Squamata - Agamidae) from arid central Africa. Bonner Zool. Beitr. 55(2): 81-87. Wahrman J. & Gourevitz P. (1973) Extreme chromosome variability in a colonising rodent. Chromosomes Today, 4: 399-424. Wallach V. (1999) Geographic distribution: Ramphotyphlops braminus (Brahminy Blind Snake). Herpetological Review 30(4): 236. Wassif K. & Hoogstraal H. (1953) The Mammals of South Sinai, Egypt. Proceedings of the Egyptian Academy of Science, 9: 63-79. Wassif K. & Soliman S. (1980) Population studies on gerbils of the Western Desert of Egypt, with special reference to Gerbillus andersoni, de Winton. Proceedings of the 9th Vertebrate Pest Conference, pp.154–160. Wassif K. (1944) On the occurrence of Paraechinus dorsalis (Anderson and De Winton) in South Sinai, with a note on the osteology of the animal. Bull. Fac. Sci. Egypt. Univ. 25: 203-211. Wassif K. (1954) On a collection of mammals from northern Sinai. Bulletin de l’Institute du Désert de l’Egypte, 3(1): 107-118. Wassif K. (1954) On the occurrence of hedgehogs of the genus Paraechinus in the El Tahreer Province of Egypt. Bull. Zool. Soc. Egypt 11: 40-47. Wassif K. (1954) The Bushy-Tailed Gerbil, Gerbillus calurus Thomas, of South Sinai. Journal of Mammalogy, 35(2):243. Wassif K., Lutfy R.G. & Wassif S. (1969) Morphological, cytological and taxonomic studies of the rodent genera Gerbillus and Dipodillus from Egypt. Proc. Egypt. Acad. Sci., 22: 22:77. Wassif K., Madkour G. & Soliman S. (1984) Fauna and Flora of Egypt. 1. On a collection of bats from Egypt. Academy of Scientific Research and Technology, Natural History Museum of Egypt, 2: 1-36. Werner Y.L. & Ashkenazi S. (2010) Notes on some Egyptian Lacertidae, including a new subspecies of Mesalina, involving the Seligmann effect. Turk J Zool, 34: 123-133. Werner Y.L. (1968) Distribution of the Saharan Sphenops sepsoides (Reptilia: Scincidae) in Israel and Jordan. Herpetologica, 24(3): 238-242. Werner Y.L. (1983) Lizards and snakes from eastern Lower Egypt in the Hebrew University of Jerusalem and Tel Aviv University with range extensions. Herpetological Review, 14(1), 29-31. White M.L., Dauphiné N.S., Mohamed A.E. (2007) Recent surveys and comparisons of birds and reptiles in St. Katherine Protectorate, Egypt, 2007. Operation Wallacea/BioMAP Report. Williams C.B. & Bishara I. (1929) The seasonal abundance of four common butterflies in Egypt. Bulletin de la Société Royale entomologiques d’Egypte, 13: 85-92 Williams G.H. (1917) Notes on some Egyptian butterflies. Bulletin de la Société entomologiques d’Egypte, 5: 91-4. Wiltshire E.P. (1948) The Lepidoptera of the Kingdom of Egypt. Bulletin de la Societe Fouad 1er d’Entomologie, 32: 203-294. Yassen A.E., Hassan H.A., Kawashti L.S. (1994) Comparative study of the karyotypes of two Egyptian species of bats, Taphozous perforatus and Taphozous nudiventris (Chiroptera: Mammalia). Experientia, 50(11):111-1114.