Multi-year presence of humpback whales in the Atlantic sector of the Southern Ocean but not during El Niño
Apr 15, 2021·,,,,,,·
36 min read
Elena Schall
Karolin Thomisch
Olaf Boebel
Gabriele Gerlach
Sari Mangia Woods
Ahmed El-Gabbas
Ilse Van Opzeeland
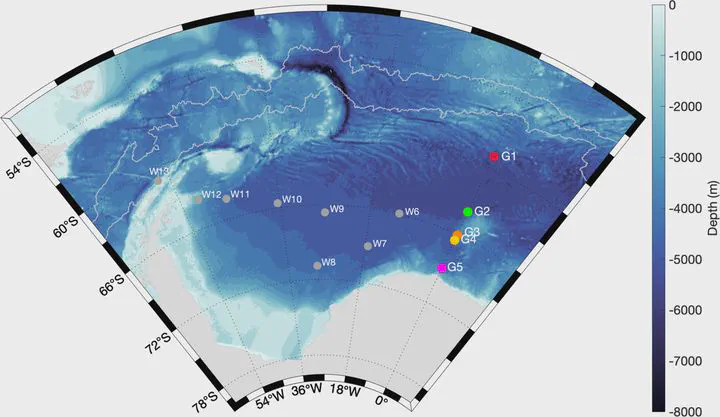
Abstract
Humpback whales are thought to undertake annual migrations between their low latitude breeding grounds and high latitude feeding grounds. However, under specific conditions, humpback whales sometimes change their migratory destination or skip migration overall. Here we document the surprising persistent presence of humpback whales in the Atlantic sector of the Southern Ocean during five years (2011, 2012, 2013, 2017, and 2018) using passive acoustic data. However, in the El Niño years 2015 and 2016, humpback whales were virtually absent. Our data show that humpback whales are systematically present in the Atlantic sector of the Southern Ocean and suggest that these whales are particularly sensitive to climate oscillations which have profound effects on winds, sea ice extent, primary production, and especially krill productivity.
Type
Publication
Nature Communications Biology 4, Article number: 790
Multi-year presence of humpback whales in the Atlantic sector of the Southern Ocean but not during El Niño
Elena Schall, Karolin Thomisch, Olaf Boebel, Gabriele Gerlach, Sari Mangia Woods, Ahmed El-Gabbas & Ilse Van Opzeeland
Communications Biology volume 4, Article number: 790 (2021) Cite this article
Abstract
Humpback whales are thought to undertake annual migrations between their low latitude breeding grounds and high latitude feeding grounds. However, under specific conditions, humpback whales sometimes change their migratory destination or skip migration overall. Here we document the surprising persistent presence of humpback whales in the Atlantic sector of the Southern Ocean during five years (2011, 2012, 2013, 2017, and 2018) using passive acoustic data. However, in the El Niño years 2015 and 2016, humpback whales were virtually absent. Our data show that humpback whales are systematically present in the Atlantic sector of the Southern Ocean and suggest that these whales are particularly sensitive to climate oscillations which have profound effects on winds, sea ice extent, primary production, and especially krill productivity.
Introduction
Humpback whales (Megaptera novaeangliae) inhabit all major oceans but these iconic large predators were thought to extend their range to polar and subpolar ecosystems only to feed during the summer months1. To reach these high latitude productive feeding areas, humpback whales undertake one of the longest mammalian migrations2. In the Atlantic sector of the Southern Ocean (ASSO), the investigation of humpback whale distribution by ship-based sighting surveys is only feasible during the austral summer and still limited due to necessary logistic effort. Therefore, systematic data on their (year-round) presence, abundance, and spatial distribution are missing for the ASSO. Insights on distribution are however vital for understanding their present and future role as large predators in structuring the Southern Ocean ecosystem3,4. A long-term autonomous passive acoustic monitoring (PAM) network was installed in 2010 to record humpback whales in their natural Antarctic environment year-round. Humpback whales are excellent candidates for PAM studies due to their year-round vocal activity of all sex and age classes5,6,7. To improve the understanding of the ecological conditions under which humpback whales use the area as a feeding ground, we investigated the inter-annual changes in humpback whale acoustic presence in relation to three environmental parameters that are key to the Southern Ocean: (1) The Southern Annular Mode (SAM) which is the dominant pattern of natural climate variability in polar and subpolar regions of the Southern Hemisphere. (2) The El Niño Southern Oscillation (ENSO) causes periodic fluctuation of sea surface temperature and air pressure originating from the tropical Pacific. Both climate oscillations have large effects on the Southern Ocean productivity8,9,10,11. (3) Local sea ice concentration (SIC) directly affects whale access to open water areas which is necessary for breathing. Indirect effects of local sea ice concentration entail its impact on the distribution of primary productivity, which in turn drives the distribution of Antarctic krill (Euphausia superba), the humpback whale’s key prey species7,8,12,13. The large-scale mooring network in the ASSO that we have been maintaining for more than ten years14 allowed us to relate the long-term trend in humpback whale acoustic presence to long-term trends in SIC and climate oscillations.
Results and discussion
Perennial humpback whale acoustic presence
We analyzed passive acoustic data of five recording positions (G1–G5) on the Greenwich Meridian from a mooring network throughout the ASSO from December 2010 to September 2018 (Fig. 1).
Fig. 1: The Atlantic sector of the Southern Ocean and recording positions on the Greenwich Meridian.
Bathymetric map of the Atlantic sector of the Southern Ocean (ASSO) including the geographic positions of the HAFOS (Hybrid Antarctic Float Observation System) mooring network in the ASSO (coastline and bathymetry data were obtained from ref. 61, 62). The five mooring positions, G1–G5, visualized with colored dots (i.e., red, green, orange, yellow, and magenta), represent the recording locations of the receivers (moored between 2010 and 2018) which were analyzed during this study. Positions G1–G5 form part of the HAFOS long-term mooring network (gray dots14). The other recording positions (W6–13) were only active during 2013 and were, therefore, not included here (but see ref. 25 for details). Light gray lines represent the minimum and maximum of the annual wintertime (21 June–21 September) maximum sea ice extent during the study period (2011–2018)63. Please note, that the lines shown do not delineate the sea ice extent of the specific years with the maximum and minimum wintertime maximum sea ice extent, but - calculated independently for each longitude—the multi-year composite of the maximum and minimum of the wintertime maximum sea ice extent during this period.
At the four oceanic recording locations (G1–G4), humpback whales were acoustically present during summer and autumn of the years 2011, 2012, 2013, 2017, and 2018 (i.e., times at which data were available for each recording position), coinciding with periods of low SIC (Fig. 2a, Supplementary Note 2, Supplementary Note 3). The high proportion of hours with humpback whale acoustic presence during autumn at G1–G4 coincided with the known timing of onset of singing behavior in Southern Hemisphere humpback whale males in lower latitude waters15,16. During this period, two or more individuals often were vocalizing at the same time in our recordings and acoustic activity was registered at all four locations in parallel, indicating the presence of multiple animals. Close to the coastal recording location (G5) where high sea ice concentrations were common during most months, humpback whales were acoustically absent or appeared only at low rates (e.g., during the years 2011–2013; Supplementary Note 2, Supplementary Note 3). At the same position (G5) and one of the oceanic locations (G3), humpback whales were acoustically present also during winter months, when SIC reached almost 100% (Fig. 2a, Supplementary Note 2, Supplementary Note 3). Although humpback whale winter acoustic presence was limited compared to the summer months, the occurrence of calls in winter was persistent between years occurring at multiple sites (Supplementary Note 3).
Fig. 2: Perennial humpback whale acoustic presence in relation to sea ice concentration, SAM and ONI.
a Boxplot of proportion of hours per day with humpback whale acoustic presence for each month from the four oceanic recording locations (G1–G4) on the Greenwich Meridian from December 2010 until September 2018 (red bars; n = 4614 days of recordings). Red dots represent outliers (on a discrete scale as proportion of hours per day). Gray-shaded areas represent months without recording data. The blue solid line and the right y-axis depict the daily averaged sea ice concentration within a 50 km radius around recording locations. b Climatic variations from 2011 until 2018 indicated by 3-month running means of the Southern Annular Mode index (SAM) as a dominant pattern of natural climate variability in polar and subpolar regions of the Southern Hemisphere and the Oceanic Niño Index (ONI) representing the periodic fluctuation of sea surface temperature and air pressure originating from the tropical Pacific. Time span of strong El Niño phase in 2015/16 is indicated by the yellow rectangle. Vertical gray lines indicate the onset of summer (S) and winter (W) and vertical dotted lines indicate the onset of spring and autumn (based on equinoxes and solstices). Horizontal dashed line represents zero-orientation line.
Humpback whale acoustic absence during El Niño
In contrast to previous and following years, only very low numbers of humpback whale vocalizations were detected in 2015 and 2016 at all locations (Fig. 2a, Supplementary Note 2, Supplementary Note 3), whereas vocalizations of other species, e.g., the Antarctic minke whale (Balaenoptera bonaerensis), were detected during this time (see ref. 17), excluding a technical artifact. Our findings are supported by the absence of opportunistic visual observations of humpback whales in the ASSO during the summer seasons 2014/2015 and 2015/2016 and only a few sightings during systematic ship-based and aerial surveys during January 201518,19,20,21. During the same years, 2015 and 2016, when humpback whales were virtually absent, SAM and ENSO (represented by the Oceanic Niño Index, ONI) both simultaneously were in strong positive phases and one of the strongest El Niño phases since the beginning of measurements was registered22 (Fig. 2b).
Modeling the effect of SIC, SAM, and ONI on the acoustic presence of humpback whales at the study location revealed that mainly SIC and ONI explain the observed pattern of humpback whale acoustic presence in the ASSO. The smoothed effects of month and SIC were highly significant because these variables explain seasonality in humpback whale presence on the feeding ground (Table 1, Fig. 3;7,12) in 5 out of 7 years. The model showed that ONI in the positive phase predicts a significantly lower probability of humpback whale acoustic presence than ONI in neutral or negative phases (Table 1, Fig. 3). The smoothed effect of the SAM index was not statistically significant (Table 1, Fig. 3). The model prediction for the SAM index showed lower predicted values at negative and high positive index values, although with higher uncertainties (Fig. 3). This appears reasonable when looking at the original time-series. The negative phases of SAM were usually registered during winter when acoustic presences are naturally low, and extreme positive phases were only registered during summer 2015 and 2016 (Fig. 2). Uncertainties at extreme index values (also for ONI) are high because these values are rare in the analyzed time-series, which potentially also explains the resulting non-significant effect of SAM. To quantify the relationship between humpback whale presence and climate indices with higher certainty, much longer time-series than presented here would be required.
Table 1 Results of best-fit model.
Fig. 3: Humpback whale acoustic presence modeled as a function of SIC, SAM, and ONI.
Model predictions of the best-fit model for the acoustic presence of humpback whales at stations G3/G4, including the smooth terms SIC, month, and SAM as well as ONI as a categorical predictor (see “Methods” for further explanation of categories). Gray-shaded areas in line plots depict 95%-confidence intervals (n = 2629 days of recordings).
Ecological pathways from environmental variabilities to humpback whales
Local sea ice concentration is one of the most important environmental factors explaining the spatio-temporal distribution of baleen whales in the Southern Ocean12,17,23,24. Similarly, sea ice dynamics play an important role in the intra-annual timing of humpback whale presence in the ASSO, showing that they move out of the area when SIC increases and that humpback whales are rarely present at SIC > 80% (see also refs. 7,25). Additionally, our data indicate that large-scale climate variabilities drive the major inter-annual variability in the acoustic presence of humpback whales on a Southern Ocean feeding ground. The most likely pathway by which climate variabilities such as ENSO and SAM could affect humpback whale presence in the Southern Ocean is through their influence on Antarctic krill (Euphausia superba)11, since the availability and distribution of this primary prey species most likely is the main driver behind the spatio-temporal distribution of humpback whales in the ASSO.
Both SAM and ENSO are factors influencing the spatial distribution and biomass of Antarctic krill by affecting winds, cloud cover, currents, sea surface temperature, and sea ice extent8,9,10,26,27,28,29. During the positive phase of SAM, the westerly wind belt surrounding the Antarctic continent contracts toward the continental shelf and climatic conditions north of the wind belt change to warmer, windier, and cloudier weather. During positive values of SAM, the oceanic feeding areas of humpback whales in the ASSO show signs of reduced sea ice extent, reduced primary production, and, in turn, also reduced krill densities8,9. Especially north of the Antarctic Polar Front, sea surface temperature increases and chlorophyll concentration decreases during positive SAM30,31. ENSO has the strongest effects on the Pacific sector of the Southern Ocean, including the Western Antarctic Peninsula8,32. The Western Antarctic Peninsula is a key habitat for Antarctic krill as a spawning and nursery ground, from which krill is transported with the Antarctic Circumpolar Current (ACC) ‘conveyor belt’ into north-eastern areas of the ASSO8. During or after the positive phase of ENSO, El Niño caused warming and the reduction of sea ice at the Western Antarctic Peninsula. Due to these climate conditions, less krill might be recruited from the Antarctic Peninsula toward the oceanic regions of the ASSO, although this process is probably delayed by multiple months considering the estimated transport speed of the ACC8,10,11,33. Also, during years of El Niño, a manifestation of negative sea surface temperature anomalies in the southwest Atlantic, termed the Antarctic dipole, is common which probably affects productivity in this area10,11,30. We, therefore, hypothesize that during the years 2015 and 2016, positive phases of both SAM and ENSO led to reduced densities of krill on the oceanic feeding grounds of humpback whales in the ASSO while potentially creating alternative prey resources in other areas.
Among baleen whale species, the humpback whale is probably the most flexible when it comes to ecological requirements, being able to adapt to wide-ranging changes in the environment with alternative migration and feeding strategies34,35. This adaptivity is probably also the reason for the continued presence of at least some humpback whales in the ASSO during austral winter. Especially female and juvenile humpback whales tend to prolong their stay on the feeding grounds or even skip migration entirely in order to fuel growth, pregnancy, or lactation with additional winter feeding 32,36. During 2015 and 2016, the main part of the South Atlantic humpback whales (probably individuals from breeding stocks from South America and Africa37) might have adapted their migration routes to exploit areas of high productivity elsewhere than in the ASSO34. For example, an unusual assemblage of humpback whale ‘super groups’ was documented in 2015 and 2016 in the southern Benguela upwelling system off South Africa38,39. Furthermore, in 2016, exceptional recordings of humpback whale song were made close to the west coast of South Africa39,40 which indicates not only the displacement of the feeding area but also multifaceted habitat use (i.e., also including reproductive activities) along this displacement. Humpback whales acoustically and physically absent from the ASSO during 2015 and 2016 might have been exploiting alternative habitats and prey resources along the west coast of South Africa34,38 or other yet undiscovered high productivity areas in the South Atlantic or adjacent waters. To date, the current knowledge on spatio-temporal trends in productivity hotspots in the Southern Hemisphere is nevertheless too sparse to explain trends in migratory predator distribution with certainty. In this context, the maintenance and implementation of further long-term observation systems such as the HAFOS mooring network from which the analyzed recordings originate (see Fig. 1 and ref. 14) are essential to detect and understand changes in this ecosystem and its functions.
Future climate change could cause the shift of ENSO and SAM toward higher frequencies of positive phases9,41, which in turn might change the overall occupancy of certain feeding areas or prey resources by humpback whales on a hemisphere-wide spatial scale. Our results clearly show that acoustic detection of whales can shed light on biophysical interactions within the fascinating Southern Ocean ecosystem. Interannual trends in the distribution or health status (e.g., ref. 42) of humpback whales and other baleen whales from the South Atlantic, but also other areas, warrant further investigation to provide information to whale stock and fishery management. Evaluating the sensitivity of keystone species to climate variabilities is essential to our understanding of the effects of climate-induced changes on the Southern Ocean ecosystem.
Methods
Passive acoustic data
Humpback whale acoustic behavior throughout the ASSO was investigated by analyzing a multi-year passive acoustic dataset (2010–2018) from five recording positions along the Greenwich Meridian (Table 2, Fig. 1). Passive acoustic recordings were obtained using SonoVaults (Develogic GmbH, Hamburg) operated on a continuous recording scheme and with a sampling rate of 5,333 or 6,857 Hz (Table 2).
Table 2 Overview of passive acoustic data.
Automatic detection and classification of humpback whale vocalizations
All available passive acoustic data were processed by the “Low Frequency Detection and Classification System” (LFDCS) developed by ref. 43 and a custom-made acoustic-context filter to detect humpback whale acoustic presence at an hourly basis (humpback whales that did not produce any sounds remained undetected). LFDCS was set up with a customized call library based on the most common vocalization types of humpback whales and other acoustically abundant Antarctic marine mammal species (i.e., Antarctic minke whale, killer whale (Orcinus orca), Weddell seal (Leptonychotes weddellii), crabeater seal (Lobodon carcinophaga), leopard seal (Hydrurga leptonyx), and Ross seal (Ommatophoca rossii)) 6,44,45,46,47,48. Parameter settings and thresholds of LFDCS and the acoustic-context filter were tuned employing multiple test datasets to optimize the automatic detection of humpback whale vocalizations to the requirements of this study. Detailed information on set up and test runs of the automatic detection process is provided in the Supplementary material (Supplementary Note 1)49.
Manual post-processing of detection results
In order to limit the temporal effort of manual post-processing, only even hours (i.e., hours starting at 00:00, 02:00, 04:00, 06:00, 08:00, 10:00, 12:00, 14:00, 16:00, 18:00, 20:00, and 22:00) were included in the further analysis. Four human analysts revised even hours with presumed humpback whale acoustic presence visually and aurally for the presence of humpback whale vocalizations by creating spectrograms in Raven Pro 1.5 (Hann Window, 1025–1790 window size, 80% overlap, 2048 DFT size50). Spectrograms were screened for humpback whale vocalizations by viewing windows of 60 s duration, spanning 0 to 1.80 kHz. Hours with confirmed humpback whale acoustic presence could contain both humpback whale social calls and humpback whale song.
Environmental data
The SIC data used for this study were extracted from a combination of satellite sensor data from the Nimbus-7 Scanning Multichannel Microwave Radiometer (SMMR), the Defense Meteorological Satellite Program (DMSP) -F8, -F11, and -F13 Special Sensor Microwave/Imrs (SSM/Is), and the DMSP-F17 Special Sensor Microwave Imager/Sounder (SSMIS), with a grid size of 25 km51. The data were used to calculate the daily SIC of the area within a 50 km radius around each recording location, with the Daily Antarctic Sea Ice Concentration packages in MATLAB52. The radius of 50 km was chosen because the acoustic range of humpback whales in the ASSO was estimated at 2–78 km7.
The two most common climate indices for the Southern Hemisphere, the Southern Annular Mode (SAM)53 and the Oceanic Niño Index (ONI, representing ENSO variabilities)22 were used in this study. SAM data were downloaded from the Climate Data Guide54 as monthly averages. ONI data were downloaded from the Climate Prediction Centre22 as three-month running means.
Statistics and reproducibility
To assess the impact of the three climate variables, SIC, ONI, and SAM on the acoustic presence of humpback whales in the ASSO, generalized additive mixed models (GAMMs) were applied in R 49,55. To model the effects of the three climate variables on the presence of humpback whales in the ASSO, the data from G3 and G4 were combined into a single time series (i.e., averaged daily SIC and daily averaged proportion of hours with humpback whale acoustic presence) because these recording positions were less than 50 km apart and provided the most complete time series (see Table 2 and Supplementary Note 2). SAM and ONI were also converted into categorical variables with negative, neutral, and positive phases to investigate responses both to small-scale and large-scale changes of climate variables (index value < −0.5 = negative; index value between −0.5 and 0.5 = neutral; index value > 0.5 = positive; see56 for details on ONI categories; the same standard was applied for SAM to create a neutral buffer between positive and negative phases). Binomial GAMMs were applied to model the daily acoustic presence/absence of humpback whales at G3/4 as a function of month, SIC, ONI (either continuous or categorical variable), and SAM (either continuous or categorical variable), including a model to account for temporal autocorrelation (functions gamm of the package mgcv57 and corARMA of the package nlme58 for an auto-regressive moving average (ARMA) model for the residuals). The optimal setup of starting values and orders for the implemented correlation structure was estimated in two ways: (1) with the function auto.arima (package forecast59), (2) by allowing the corARMA function to estimate its parameters directly from our data (used in the final model). The variables month and SIC were modeled with a cyclic smoothing term to account for the natural seasonal fluctuations. Model selection was performed using the Akaike Information Criterion (AIC), adjusted r-squared values, and the analyses of residuals.
Data availability
The hourly humpback whale acoustic presence data that support the findings of this study are available on Dryad (https://doi.org/10.5061/dryad.ncjsxkss0) with the identifier https://doi.org/10.5061/dryad.ncjsxkss060.
References
- Clapham, P. J. in Encyclopedia of marine mammals 489–492 (Elsevier, 2018).
- Stevick, P. T. et al. A quarter of a world away: female humpback whale moves 10 000 km between breeding areas. Biol. Lett. 7, 299–302 (2010).
- Nicol, S. et al. Southern Ocean iron fertilization by baleen whales and Antarctic krill. Fish. Fish. 11, 203–209 (2010).
- Smetacek, V. & Nicol, S. Polar ocean ecosystems in a changing world. Nature 437, 362–368 (2005).
- Dunlop, R. A. Potential motivational information encoded within humpback whale non-song vocal sounds. J. Acoustical Soc. Am. 141, 2204–2213 (2017).
- Stimpert, A. K., Au, W. W. L., Parks, S. E., Hurst, T. & Wiley, D. N. Common humpback whale (Megaptera novaeangliae) sound types for passive acoustic monitoring. J. Acoustical Soc. Am. 129, 476–482 (2011).
- Van Opzeeland, I., Van Parijs, S., Kindermann, L., Burkhardt, E. & Boebel, O. Calling in the cold: pervasive acoustic presence of humpback whales (Megaptera novaeangliae) in Antarctic coastal waters. PLoS ONE 8, 1–7 (2013).
- Siegel, V. Biology and Ecology of Antarctic Krill. (Springer, 2016).
- Atkinson, A. et al. Krill (Euphausia superba) distribution contracts southward during rapid regional warming. Nat. Clim. Change 9, 142–147 (2019).
- Loeb, V. J., Hofmann, E. E., Klinck, J. M., Holm-Hansen, O. & White, W. B. ENSO and variability of the Antarctic Peninsula pelagic marine ecosystem. Antarctic Sci. https://doi.org/10.1017/s0954102008001636 (2009).
- Loeb, V. J. & Santora, J. A. Climate variability and spatiotemporal dynamics of five Southern Ocean krill species. Prog. Oceanogr. 134, 93–122 (2015).
- Bombosch, A. et al. Predictive habitat modelling of humpback (Megaptera novaeangliae) and Antarctic minke (Balaenoptera bonaerensis) whales in the Southern Ocean as a planning tool for seismic surveys. Deep-Sea Res. Part I: Oceanographic Res. Pap. 91, 101–114 (2014).
- Brierley, A. S. et al. Antarctic krill under sea ice: elevated abundance in a narrow band just south of ice edge. Science 295, 1890–1892 (2002).
- Rettig, S. et al. in 1st International Conference and Exhibition on Underwater Acoustics. (eds Papadakis, J. & Bjorno, L.) 1669–1674 (2013).
- Garland, E. C. et al. Humpback whale song on the Southern Ocean feeding grounds: Implications for cultural transmission. PLoS ONE 8, e79422 (2013).
- Stimpert, A. K., Peavey, L. E., Friedlaender, A. S. & Nowacek, D. P. Humpback whale song and foraging behavior on an Antarctic feeding ground. PLoS ONE 7, e51214 (2012).
- Filun, D. et al. Frozen verses: Antarctic minke whales (Balaenoptera bonaerensis) call predominantly during austral winter. R. Soc. Open Sci. 7, 192112 (2020).
- Boebel, O. The Expedition PS89 of the Research Vessel POLARSTERN to the Weddell Sea in 2014/2015. (Alfred Wegener Institute, Helmholtz Centre for Polar and Marine Research, Bremerhaven, 2015).
- Burkhardt, E. Whale sightings during Polarstern cruise PS96 (ANT-XXXI/2), https://doi.org/10.1594/PANGAEA.923113 (2020).
- Herr, H., Viquerat, S. & Siebert, U. Aerial cetacean survey Southern Ocean 2014/2015, https://doi.org/10.1594/PANGAEA.894938 (2018).
- Herr, H., Viquerat, S. & Siebert, U. Ship based cetacean survey Southern Ocean 2014/2015, https://doi.org/10.1594/PANGAEA.894873 (2018).
- National Oceanic and Atmospheric Administration & Department of Commerce. Climate Prediction Centre (CPC) Oceanic Nino Index (2019).
- Thomisch, K. et al. Spatio-temporal patterns in acoustic presence and distribution of Antarctic blue whales Balaenoptera musculus intermedia in the Weddell Sea. Endanger. Species Res. 30, 239–253 (2016).
- Širović, A. et al. Seasonality of blue and fin whale calls and the influence of sea ice in the Western Antarctic Peninsula. Deep Sea Res. Part II: Topical Stud. Oceanogr. 51, 2327–2344 (2004).
- Schall, E. et al. Large-scale spatial variabilities in the humpback whale acoustic presence in the Atlantic sector of the Southern Ocean. R. Soc. Open Sci. 7, 201347 (2020).
- Loeb, V., Hofmann, E. E., Klinck, J. M. & Holm-Hansen, O. Hydrographic control of the marine ecosystem in the South Shetland-Elephant Island and Bransfield Strait region. Deep Sea Res. Part II: Topical Stud. Oceanogr. 57, 519–542 (2010).
- Sallée, J.-B., Speer, K. & Rintoul, S. Zonally asymmetric response of the Southern Ocean mixed-layer depth to the Southern Annular Mode. Nat. Geosci. 3, 273–279 (2010).
- Kim, Y. S. & Orsi, A. H. On the variability of Antarctic Circumpolar Current fronts inferred from 1992–2011 altimetry. J. Phys. Oceanogr. 44, 3054–3071 (2014).
- Yuan, X. ENSO-related impacts on Antarctic sea ice: a synthesis of phenomenon and mechanisms. Antarct. Sci. 16, 415 (2004).
- Meredith, M. P., Murphy, E. J., Hawker, E. J., King, J. C. & Wallace, M. I. On the interannual variability of ocean temperatures around South Georgia, Southern Ocean: Forcing by El Niño/Southern Oscillation and the southern annular mode. Deep Sea Res. Part II: Topical Stud. Oceanogr. 55, 2007–2022 (2008).
- Lovenduski, N. S. & Gruber, N. Impact of the Southern Annular Mode on Southern Ocean circulation and biology. Geophys. Res. Lett. 32, 1–4 (2005).
- Craig, A. S., Herman, L. M., Gabriele, C. M. & Pack, A. A. Migratory timing of humpback whales (Megaptera novaeangliae) in the central north Pacific varies with age, sex and reproductive status. Behaviour 140, 981–1001 (2003).
- Hofmann, E. E., Klinck, J. M., Locarnini, R. A., Fach, B. & Murphy, E. Krill transport in the Scotia Sea and environs. Antarct. Sci. 10, 406–415 (1998).
- Barendse, J. et al. Migration redefined? Seasonality, movements and group composition of humpback whales Megaptera novaeangliae off the west coast of South Africa. Afr. J. Mar. Sci. 32, 1–22 (2010).
- Witteveen, B. H., Foy, R. J., Wynne, K. M. & Tremblay, Y. Investigation of foraging habits and prey selection by humpback whales (Megaptera novaeangliae) using acoustic tags and concurrent fish surveys. Mar. Mammal. Sci. 24, 516–534 (2008).
- Brown, M. R., Corkeron, P. J., Hale, P. T., Schultz, K. W. & Bryden, M. M. Evidence for a sex-segregated migration in the humpback whale (Megaptera novaeangliae). Proc. R. Soc. Lond. B 259, 229–234 (1995).
- International Whaling Commission. Report on the workshop on the comprehensive assessment of Southern Hemisphere humpback whales. J. Cetacea. Res. Manag. Spec. Issue 3, 1–50 (2011).
- Findlay, K. P. et al. Humpback whale “super-groups” – A novel low-latitude feeding behaviour of Southern Hemisphere humpback whales (Megaptera novaeangliae) in the Benguela Upwelling System. PLOS ONE 12, e0172002 (2017).
- Gridley, T., Silva, M., Wilkinson, C., Seakamela, S. & Elwen, S. H. Song recorded near a super-group of humpback whales on a mid-latitude feeding ground off South Africa. J. Acoustical Soc. Am. 143, EL298–EL304 (2018).
- Ross-Marsh, E., Elwen, S., Prinsloo, A., James, B. & Gridley, T. Singing in South Africa: monitoring the occurrence of humpback whale (Megaptera novaeangliae) song near the Western Cape. Bioacoustics, 1–17, https://doi.org/10.1080/09524622.2019.1710254 (2020).
- Cai, W. et al. Increasing frequency of extreme El Niño events due to greenhouse warming. Nat. Clim. Change 4, 111–116 (2014).
- Bengtson Nash, S. M. et al. Signals from the south; humpback whales carry messages of Antarctic sea‐ice ecosystem variability. Glob. Change Biol. 24, 1500–1510 (2018).
- Baumgartner, M. F. & Mussoline, S. E. A generalized baleen whale call detection and classification system. J. Acoustical Soc. Am. 129, 2889–2902 (2011).
- Klinck, H. et al. Long-range underwater vocalizations of the crabeater seal (Lobodon carcinophaga). J. Acoustical Soc. Am. 128, 474–479 (2010).
- Risch, D. et al. Mysterious bio-duck sound attributed to the Antarctic minke whale (Balaenoptera bonaerensis). Biol. Lett. 10, 20140175 (2014).
- Schall, E. & Van Opzeeland, I. Calls produced by Ecotype C killer whales (Orcinus orca) off the Eckstrom iceshelf, Antarctica. Aquat. Mamm. 43, 117–126 (2017).
- Van Opzeeland, I. et al. Acoustic ecology of Antarctic pinnipeds. Mar. Ecol. Prog. Ser. 414, 267–291 (2010).
- Dunlop, R. A., Cato, D. H. & Noad, M. J. Non-song acoustic communication in migrating humpback whales (Megaptera novaeangliae). Mar. Mammal. Sci. 24, 613–629 (2008).
- Schall, E. & El-Gabbas, A. Humpback-whale-acoustic-detection-and-environmental-modelling, https://github.com/elenaschall/Humpback-whale-acoustic-detection-and-environmental-modelling (GitHub, GitHub, 2021).
- Bioacoustics, Research & Program. Raven Pro: Interactive Sound Analysis Software (Version 1.5) http://ravensoundsoftware.com/ (The Cornell Lab of Ornithology, Ithaca, NY, 2014).
- Cavalieri, D., Parkinson, C., Gloersen, P. & Zwally, H. Sea ice concentrations from Nimbus-7 SMMR and DMSP SSM/I-SSMIS passive microwave data, version 1. Boulder, Colorado USA, NASA National Snow and Ice Data Center Distributed Active Archive Center 10, https://doi.org/10.5067/8GQ8LZQVL0VL (1996).
- Greene, C. A. Daily Antarctic sea ice concentration (2020).
- Marshall, G. J. Trends in the southern annular mode from observations and reanalyses. J. Clim. 16, 4134–4143 (2003).
- Marshall, G. & National Center for Atmospheric Research Staff (Eds). The climate data guide: Marshall Southern Annular Mode (SAM) index (Station-based), (2019).
- R Core Team. R: A language and environment for statistical computing, https://www.R-project.org/ (R Foundation for Statistical Computing, Vienna, Austria, 2018)
- National Oceanic and Atmospheric Administration (NOAA) & Climate Prediction Centre (CPC). Oceanic Nino Index (2019).
- Wood, S. N. Generalized additive models: an introduction with R (CRC press, 2017).
- Pinheiro, J., Bates, D., DebRoy, S. & Sarkar, D. _nlme: Linear and nonlinear mixed effects models. R package version 3.1-145 (R CoreTeam, 2020).
- Hyndman, R. et al. forecast: Forecasting functions for time series and linear models_. R. package version 8.11, http://pkg.robjhyndman.com/forecast (2020)..
- Schall, E. et al. Humpback whale acoustic presence in the Atlantic sector of the Southern Ocean, https://doi.org/10.5061/dryad.ncjsxkss0 (2021).
- Wessel, P. & Smith, W. H. A global, self‐consistent, hierarchical, high‐resolution shoreline database. J. Geophys. Res.: Solid Earth 101, 8741–8743 (1996).
- Amante, C. & Eakins, B. W. ETOPO1 arc-minute global relief model: procedures, data sources and analysis, https://doi.org/10.7289/V5C8276M (2009).
- Spreen, G., Kaleschke, L. & Heygster, G. Sea ice remote sensing using AMSR-E 89-GHz channels. J. Geophys Res. Oceans 113, C02S03 (2008). Acknowledgements Thanks to Develogic GmbH, Hamburg, to the logistics department of the Alfred Wegener Institute, Bremerhaven, the mooring team of the AWI’s physical oceanography department, to Reederei F. Laeisz GmbH, Rostock, and the crews of RV Polarstern expeditions ANT-XXVII/2, ANT-XXIX/2, PS89, PS103 for their contribution to the development, setup or maintenance of the passive acoustic recording array. We thank Maria Mallet and Katharina Hiemer for assistance with the manual post-processing of acoustic data and the whole team of the Ocean Acoustics Lab for the productive discussions on this study. We also want to thank Mark Baumgartner and Genevieve Davis for the assistance in setting up LFDCS and Bettina Meyer for discussions on Antarctic krill ecology. Funding Open Access funding enabled and organized by Projekt DEAL. Author information Affiliations Alfred Wegener Institute for Polar and Marine Research, Bremerhaven, Germany Elena Schall, Karolin Thomisch, Olaf Boebel, Sari Mangia Woods, Ahmed El-Gabbas & Ilse Van Opzeeland Helmholtz Institute for Functional Marine Biodiversity, Carl von Ossietzky University Oldenburg, Oldenburg, Germany Gabriele Gerlach & Ilse Van Opzeeland Carl von Ossietzky University Oldenburg, Oldenburg, Germany Gabriele Gerlach Marine Evolution and Conservation, Groningen Institute of Evolutionary Life Sciences, University of Groningen, Groningen, The Netherlands Sari Mangia Woods Contributions E.S. analyzed the data and wrote the manuscript. K.T. participated in some data collection and helped draft the manuscript. O.B. coordinated the study and collected the majority of the data. G.B. guided the analysis and helped draft the manuscript. S.M.W. helped with data analysis. A.E.-G. supervised the statistical analysis. I.V.O. coordinated the study, collected part of the data, and helped draft the manuscript. All the authors reviewed and contributed to the final document edits. All the authors gave the final approval for publication. Multi-year presence of humpback whales in the Atlantic sector of the Southern Ocean but not during El Niño
- Supplementary Information - Authors Elena Schall, Karolin Thomisch, Olaf Boebel, Gabriele Gerlach, Sari Mangia Woods, Ahmed El- Gabbas, Ilse Van Opzeeland Supplementary Note 1 Material and Methods Automatic detection and classification of humpback whale vocalizations All available passive acoustic data were processed by the ‘Low frequency detection and classification system’ (LFDCS) developed by Baumgartner and Mussoline 1 in order to automatically detect and classify humpback whale vocalizations. A call library for humpback whale call types from the Atlantic sector of the Southern Ocean was constructed using data from two recording periods with confirmed humpback whale acoustic presence: May 2011 and June 2013 from recorders deployed at 59°S 0°E, 64°S 0°E, and 61°S 55°W. In total seven common humpback whale call types were included in the call library, comprising between 153 and 332 selected exemplars (Table 1). To avoid the miss-classification of vocalizations from other marine mammal species (inhabiting the wider Weddell Sea area and occupying a similar frequency range as humpback whales) as a humpback whale vocalization, at least one common call type per species was determined to be included in the call library. In total seven additional call types from other vocal marine mammal species were included in the call library with between 160 and 321 selected exemplars per call type (Table 1; 2-5). The humpback whale call type 18, a low frequency downsweep (LF DS), has acoustic characteristics that were very similar to those of the common low frequency downsweeps of other baleen whale species 6-8. For this reason, the automatic detections of this particular call type cannot be considered as a reliable sign for humpback whale acoustic presence and corresponding detections were therefore only considered in combination with other humpback whale call type detections. Table 1. LFDCS call library of all tonal sounds which serve as choices for the classification algorithm. Call type numbers were assigned arbitrarily (Humpback whale call type numbers were chosen to match the call type number given in catalogue for manual analysis). Call type names were assigned, based on the visual and aural appearance of the call types during analysis (e.g., ‘LF’ = low frequency; ‘DS’ = downsweep). Species Call Type Name N exemplars Humpback whale 1 Moan 200 3 Roof 192 4 J 270 5 L 191 6 MoanUp 166 18 LF DS 153 19 LF Moan 332 Minke whale 30 Bioduck call 213 Killer whale 31 Excited DS 268 Weddell seal 32 Long DS 173 Crabeater seal 33 Low Moan 160 Leopard Seal 34 Low trill 275 Leopard Seal 35 High trill 139 Ross Seal 36 Sirene call 321 Figure 1. Exemplary humpback whale vocalizations of the call library. Figure 2. Exemplary marine mammal vocalizations of the call library. Call type names were chosen, based on the visual and aural appearance of the call types during analysis (‘DS’ = downsweep). In order to tune the LFDCS detection and classification parameters to yield the best possible detector/classifier performance, a two-step evaluation analysis was applied to selected subsets of acoustic recordings. The first evaluation step was performed on a subset of the passive acoustic data summing up to 30 recording hours (30h-dataset). The 30h-dataset was selected in order to contain different quality humpback whale social calls and songs in different noise conditions (i.e., environmental, anthropogenic and electronic), silent periods, periods with only noise and periods with vocalizations from other marine mammals (i.e., Antarctic blue whale, fin whale, Antarctic minke whale, sperm whale, killer whale, leopard seal, Ross seal, crabeater seal and Weddell seal). This 30h-dataset was compiled from six different recording locations, three different years and all four different seasons. The 30h-dataset was manually screened in Raven Pro 1.5 (Hann Window, 1025 window size, 80% overlap, 2048 DFT size; Bioacoustics Research Program 2014) by marking the start-time of each clearly assignable humpback whale vocalization. Manually detected vocalizations were manually classified into the seven tonal humpback whale call types included in the LFDCS call library. Further, the 30h-dataset was repeatedly automatically processed in LFDCS using the above mentioned customized call library with detection and classification parameters changing between single LFDCS runs in order to determine the optimal parameter settings (23 adjustable parameters; see Baumgartner and Mussoline 1 for parameter descriptions). Parameter settings were optimized in a parameter optimization cycle, which cycled through 2400 LFDCS runs with randomly chosen parameter combinations. For each run, automatic humpback whale call detections were compared against manual humpback whale call detections with a start-time buffer of 1.8 s (validated via manual comparison of detection start-times in the LFDCS browse mode). The number of true positive, false positive and false negative detections was determined and used to calculate recall, precision and ultimately F1 score 9. The second evaluation step was designed to evaluate detection efficiency on an hourly basis. For this purpose, ten different parameter settings were chosen from the optimization cycle runs based on their step one performance results, i.e. the balance between recall, precision and F1 score (Figure 3). Step two of the evaluation procedure was performed on a subset of the passive acoustic data summing up to 150 recording hours (150h-dataset) compiled from the same six locations, three years and four seasons as the 30h-dataset. Likewise, the 150h-dataset was composed of recordings with similar variable acoustic conditions as the 30h-dataset (i.e., including different noise conditions and vocalizations of the different marine mammal species). The 150h-dataset was manually screened in Raven Pro 1.5 (Hann Window, 1025 window size, 80% overlap, 2048 DFT size; Bioacoustics Research Program 2014) by detecting humpback whale acoustic presence on an hourly basis. In LFDCS, the 150h-dataset was processed in ten runs, each with one of the parameter settings chosen from the first evaluation step. For each run, automatic humpback whale detections were compared against manual detections of humpback whale acoustic presence per hour. To minimize false positive hours due to confusion with other species’ vocalizations an additional acoustic-context filter was applied before evaluating the results. This acoustic- context filter was based on two conditions: (1) When the number of good quality detections (i.e., Mahalanobis distance (MD) ≤ 2 and signal-to-noise ratio (SNR) ≥ 14) of another species’ call type similar to a humpback whale call type (Table 2) within a respective hour exceeds an hourly call rate (CR) threshold (i.e., 4 calls per hour), and (2) when the number of humpback whale good quality detections (i.e., MD ≤ 2 and SNR ≥ 14; summing over all call types), within a respective hour is lower than an hourly CR threshold (i.e., 6 calls per hour). In case these two conditions were met, all detections of the humpback whale call type similar to the respective other species’ call type were deleted from the respective hour. Figure 3. Performance evaluation of 2400 LFDCS runs on the 30h-dataset during step one of the performance evaluation. Ten distinct parameter settings with differently balanced performances (marked with black stars) were chosen for the second evaluation step. Table 2. Potential sources of miss-identification of humpback whale calls with other species’ call types. Humpback whale call types in the first column were frequently mistaken (by LFDCS) for call types of other species as listed in the second column (‘CT’ = call type). Humpback whale call type Similar call type from other species CT1 leopard seal Low trill (CT34), Crabeater seal Low Moan (CT33) CT3 Ross seal Sirene call (CT36) CT5 killer whale Excited DS (CT31), Weddell seal Long DS (CT32) CT6 Leopard seal Low trill (CT34) CT18 Antarctic minke whale Bioduck call (CT30) Remaining humpback whale call detections were the basis for step two of the evaluation procedure, where hourly detection efficiency of LFDCS and the acoustic context filter was estimated applying different detection quality and hourly CR thresholds. In total seven MD (i.e., 1.5-4.5), seven SNR (i.e., 8-14dB) and 30 CR thresholds (i.e., 1-30) were tested, summing up to 1470 threshold combinations. For each threshold combination the probability of HW hourly presence (ProbPres) and the probability of false negative hours (ProbFN) were calculated: with the number of humpback whale true positive hours (’() +, -./01), the number of humpback whale false negative hours (’()23 -./01), the number of humpback whale positive predicted hours (’() 405678956 -./01), and the number of humpback whale negative predicted hours (’() %5:;97<5 405678956 -./01). The parameter setting and MD/SNR/CR threshold combination of the run with the highest respective ProbPres at a ProbFN lower than 20% was finally selected to process the full dataset (Table 3; see Baumgartner and Mussoline 1 for parameter descriptions). Resulting automatically detected hours with presumed humpback whale acoustic presence will be termed presumed humpback whale presence (pHWP) hours in the following. Table 3. Final LFDCS parameter settings and Mahalanobis Distance (MD)/Signal-to-Noise Ratio (SNR)/Call Rate (CR) threshold combination. For parameter descriptions see Baumgartner and Mussoline Comparative SNR measurements In order to evaluate the influence of the missed humpback whale detections for the resulting acoustic presence data of humpback whales in the wider Weddell Sea area, comparative SNR measurements were conducted. Both detected and missed humpback whale calls of the 150h-dataset from the LFDCS run using the final chosen parameter settings (chosen by the highest ProbPres and a ProbFN lower than 20%) were identified to measure their SNR. In all false negative hours, humpback whale calls were manually identified in Raven Pro 1.5 and their SNRs were measured by comparing the average power (dB re 1µPa) of the spectrum with the call’s duration and bandwidth with the average power of two spectra with the same dimensions, one before and one after the call, respectively. From all true positive hours, a number of hours was randomly chosen in order to match the number of false negative hours. Three detected calls were randomly chosen from each of these selected true positive hours and SNRs of these calls were measured applying the same method as described above. Results Detector/classifier performance evaluation In the 30h-dataset, 5274 humpback whale vocalizations were manually detected and classified into the seven humpback whale call types included in the LFDCS call library, with 357 manual detections of call type 1, 439 of call type 3, 2471 of call type 4, 1056 of call type 5, 508 of call type 6, 338 of call type 18, and 100 of call type 19. Different parameter settings in LFDCS yielded different performance results in terms of recall, precision and F1-score (Figure 3). The selected parameter setting resulted in a recall of 22%, a precision of 56%, and a F1-score of 0.32. In the present study, for the detection of humpback whale presence on an hourly basis, it was considered more important to aim for a higher precision of the automated detector at the cost of a lower recall (because it was not necessary to detect all vocalizations in order to capture hourly acoustic presence). The final parameter setting in combination with the acoustic-context filter and specific MD, SNR and CR thresholds resulted in a good detection performance in terms of hourly humpback whale presence (Figure 4). At an hourly CR threshold of at least 10 calls/hour the automatic detection process yielded a ProbPres of 75% and a ProbFN of only 18%. Because it is common practice to exclude vocalizations with a SNR below 10 dB from the analysis 10,11, the quality of missed vocalizations in these 18% of false negative hours was checked. In comparison to the sampled humpback whale vocalizations in the detected hours, the vocalizations in the false negative hours had SNRs which were mainly below 10 dB (Figure 5). Figure 4. Final detection performance of hourly humpback whale acoustic presence in the 150h-dataset. The probability of humpback whale acoustic presence in the recording hours is depicted in blue on the left y-axis and the probability of false negative hours is depicted in orange on the right y-axis. The x-axis represents the hourly call rate observed by the detector after applying the acoustic-context filter. Figure 5. Measured signal-to-noise ratios (SNR) of humpback whale (HW) vocalizations. Blue bars (left y-axis) represent SNRs of vocalizations in false negative (missed) hours and orange bars (right y-axis) represent SNRs of vocalizations in detected hours. Supplementary Note 2 Figure 6. Boxplots of daily proportions of hours with humpback whale acoustic presence from the five recording positions (G1-G5) on the Greenwich Meridian displayed per month from December 2010 until September 2018 (center line, median; box limits, upper and lower quartiles; whiskers, 1.5x interquartile range; points, outliers). Grey bars represent months without recording data, yellow, red, blue and green shades indicate summer, fall, winter and spring seasons, respectively. Single points indicate single daily observations of humpback whale acoustic presence. Supplementary Note 3 Figure 7. Heatmap showing the average number of days with humpback whale acoustic presence for all months for recording stations G1-5. Darker colors indicate higher presence, numbers in cells represent the average number of days with presence per month per recording station. Supplementary References 1 Baumgartner, M. F. & Mussoline, S. E. A generalized baleen whale call detection and classification system. J Acoust Soc Am 129, 2889-2902, doi:10.1121/1.3562166 (2011). 2 Schall, E. & Van Opzeeland, I. Calls produced by Ecotype C killer whales (Orcinus orca) off the Eckstrom iceshelf, Antarctica. Aquatic Mammals 43, 117-126, doi:10.1578/AM.43.2.2017.117 (2017). 3 Van Opzeeland, I. et al. Acoustic ecology of Antarctic pinnipeds. Marine Ecology Progress Series 414, 267-291, doi:10.3354/meps08683 (2010). 4 Risch, D. et al. Mysterious bio-duck sound attributed to the Antarctic minke whale (Balaenoptera bonaerensis). Biol Lett 10, 20140175, doi:10.1098/rsbl.2014.0175 (2014). 5 Klinck, H. et al. Long-range underwater vocalizations of the crabeater seal (Lobodon carcinophaga). The Journal of the Acoustical Society of America 128, 474-479, doi:10.1121/1.3442362 (2010). 6 Ou, H., Au, W. W. L., Van Parijs, S., Oleson, E. M. & Rankin, S. Discrimination of frequency- modulated Baleen whale downsweep calls with overlapping frequencies. Journal of the Acoustical Society of America 137, 3024-3032, doi:10.1121/1.4919304 (2015). 7 Baumgartner, M. F. et al. Low frequency vocalizations attributed to sei whales (Balaenoptera borealis). Journal of the Acoustical Society of America 124, 1339-1349 (2008). 8 Edds-Walton, P. L. Acoustic communication signals of mysticete whales. Bioacustics 8, 47-60 (1997). 9 Powers, D. M. Evaluation: from precision, recall and F-measure to ROC, informedness, markedness and correlation. Journal of Machine Learning Technologies (2011). 10 Dunlop, R. A., Cato, D. H. & Noad, M. J. Non-song acoustic communication in migrating humpback whales (Megaptera novaeangliae). Marine Mammal Science 24, 613-629, doi:10.1111/j.1748- 7692.2008.00208.x (2008). 11 Magnúsdóttir, E. E. & Lim, R. Subarctic singers: Humpback whale (Megaptera novaeangliae) song structure and progression from an Icelandic feeding ground during winter. PloS one 14, e0210057 (2019).