Distribution of the Egyptian reptiles under current and future climates
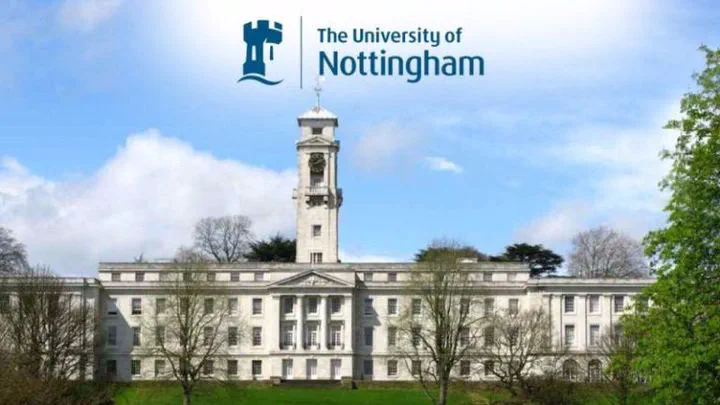
Climate change has caused range shifts and extinctions of many species in the past. In this study, the effects of climate change on Egyptian reptiles, as a representative of the Egyptian fauna, was investigated for the first time using species distribution models, relatively new tools now used in a variety of fields from conservation planning to the assessment of species’ responses to climate change. In this study, the Maxent algorithm was used to model the current and future distributions of 75 terrestrial reptile species from Egypt. The modelled distribution for current conditions for each species was projected into the future for three time slices (2020, 2050 and 2080) using two emission scenarios (A2a and B2a) from four global circulation models (CCCma, CSIRO, HadCM3 and NIES99) and under two assumptions of dispersal ability (unlimited dispersal and no dispersal). This produced a total of 48 projections for each species. Current and future species richness patterns were determined from the results using the average response across the different global circulation models to represent a consensus view. For each species, possible changes in range were calculated and used to assess future threat status. A national Red Data listing for the Egyptian reptiles was determined to show which species require more conservation measures. Zonation software was used for conservation prioritization to show which areas require more protection under current and future climates, and to assess the effectiveness of Egypt’s Protected Areas network to conserve reptiles.
Climate change is predicted to vary in its effects spatially, with some areas characterized by increased species richness while others show declines. Future range changes are predicted to vary among species and among different future projections, from the loss of the entire range (Tarentola mindiae and Hemidactylus robustus) to large gains in range (Hemidactylus flaviviridis). No species was predicted to lose its entire currently suitable range under all scenarios. Tarentola mindiae and Hemidactylus robustus were predicted to become extinct from Egypt in the future in at least one future projection. Another eight species were predicted to lose more than 80% of their current distribution in the future. According to IUCN guidelines and criteria, under current conditions, three species were classified as nationally Endangered and 24 species as Vulnerable. Although Protected Areas have greater conservation value compared to unprotected areas, Egypt’s Protected-Areas network seems to be inadequate to conserve Egyptian reptiles. My results suggest the need to construct new Protected Areas in a variety of places across northern Egypt from between Mersa Matruh and Sallum to the Gebel El-Hallal area in northern Sinai. Some Protected Areas require stricter protection in the future to counter the threats derived from climate change.
Supervisor:Prof. Francis Gilbert, School of Life Sciences, Nottingham University, Nottingham, UK.
By: Ahmed El-Gabbas
Supervisor: Dr. Francis Gilbert
A thesis presented to the School of Biology, The University of Nottingham in partial fulllment of the requirements for the degree of Master by Research (Conservation Biology).
The University of Nottingham, School of Biology,
September, 2012 https://elgabbas.github.io/ II
Abstract
Climate change has caused range shifts and extinctions of many species in the past. In this study, the effects of climate change on Egyptian reptiles, as a representative of the Egyptian fauna, was investigated for the first time using species distribution models, relatively new tools now used in a variety of fields from conservation planning to the assessment of species’ responses to climate change. In this study, the Maxent algorithm was used to model the current and future distributions of 75 terrestrial reptile species from Egypt. The modelled distribution for current conditions for each species was projected into the future for three time slices (2020, 2050 and 2080) using two emission scenarios (A2a and B2a) from four global circulation models (CCCma, CSIRO, HadCM3 and NIES99) and under two assumptions of dispersal ability (unlimited dispersal and no dispersal). This produced a total of 48 projections for each species. Current and future species richness patterns were determined from the results using the average response across the different global circulation models to represent a consensus view. For each species, possible changes in range were calculated and used to assess future threat status. A national Red Data listing for the Egyptian reptiles was determined to show which species require more conservation measures. Zonation software was used for conservation prioritization to show which areas require more protection under current and future climates, and to assess the effectiveness of Egypt’s Protected Areas network to conserve reptiles. Climate change is predicted to vary in its effects spatially, with some areas characterized by increased species richness while others show declines. Future range changes are predicted to vary among species and among different future projections, from the loss of the entire range (Tarentola mindiae and Hemidactylus robustus) to large gains in range (Hemidactylus flaviviridis). No species was predicted to lose its entire currently suitable range under all scenarios. Tarentola mindiae and Hemidactylus robustus were predicted to become extinct from Egypt in the future in at least one future projection. Another eight species were predicted to lose more than 80% of their current distribution in the future. According to IUCN guidelines and III
criteria, under current conditions, three species were classified as nationally
Endangered and 24 species as Vulnerable.
Although Protected Areas have greater conservation value compared to
unprotected areas, Egypt’s Protected-Areas network seems to be inadequate
to conserve Egyptian reptiles. My results suggest the need to construct new
Protected Areas in a variety of places across northern Egypt from between
Mersa Matruh and Sallum to the Gebel El-Hallal area in northern Sinai. Some
Protected Areas require stricter protection in the future to counter the threats
derived from climate change.
Keywords: Climate Change; Conservation prioritization; Egyptian herpetofauna; Egyptian reptiles; Maxent; Species Distribution Modelling; Species extinctions; Species range change; Species richness; Species Turnover; Zonation.
IV
Acknowledgement
First of all, I sincerely thank Allah Almighty for giving me strength and ability to
complete this study.
I would like to express my deep gratitude to my supervisor, Dr. Francis
Gilbert, for his great support, advice and mentorship throughout this study. I
am particularly grateful to him and Prof. Samy Zalat (Suez Canal University,
Egypt) for their enthusiasm, encouragement and guidance through the past
several years since working under their supervision at the BioMAP project.
This thesis would not have been completed without their support.
Many thanks are given for: Dr. Tim Newbold (UNEP-WCMC and
Microsoft Research Fellow) for introducing GIS and Species Distribution
Modelling for me, giving me a chance to join his PhD fieldwork in Egypt and for
sharing his field records; Dr. Sherif Baha El Din for providing his valuable
database records of the Egyptian herpetofauna; Dr. Moustafa Fouda (former
director of the Nature Conservation Sector, Egyptian Environmental Affairs
Agency) for his support throughout my work with BioMAP project and Nature
Conservation Sector; Mindy Baha El Din for providing valuable information on
the current status of the Egyptian tortoise; Abdelwahab Afefe and Mahmoud
Boghdady (Nature Conservation Sector) for providing me of the coordinates of
the latest declared Protected Areas (Mount Kamel Meteor and El-Wahat El-
Bahreya); BioMAP former staff and Nature Conservation Sector team; and all
the scientists and volunteers who contributed data to the databases used.
This research study is sponsored by Chevening Scholarships. I am
indebted to British Council in Egypt and the UK Foreign and Commonwealth
Office for their generous support throughout my stay in Britain.
Finally, I would like to thank my parents and sisters for their continuous
support, encouragement and patience.
V
“Dedicated to the soul of Prof. Mohamed El Kassas, the outstanding Egyptian naturalist and doyen of wildlife conservation in Egypt, who passed away earlier this year. ”
VI
Table of Contents
Abstract …………………………………………………………………………………………. II Acknowledgement ………………………………………………………………………….. IV List of Tables…………………………………………………………………………………… VIII List of Figures ………………………………………………………………………………… IX List of Acronyms …………………………………………………………………………….. XII
Introduction …………………………………………………………………………………… 1 Issues about the data required for distribution models ……………….. 3 The choice of model algorithms ……………………………………………….. 6 Assessing model performance …………………………………………………. 7 Maximum entropy modelling (Maxent) ………………………………………. 13 Climate change and biodiversity ………………………………………………. 18 Relevance to Egypt ………………………………………………………………….. 21 Aim of study …………………………………………………………………………… 24
Methods ………………………………………………………………………………………… 25 Study area – Egypt …………………………………………………………………. 25 Study species – Egyptian reptiles …………………………………………….. 25 Environmental predictor variables …………………………………………….. 27 Species distribution modelling ………………………………………………….. 39 Comparisons across species …………………………………………………… 42 Area prioritization for conservation ……………………………………………. 46
Results ………………………………………………………………………………………….. 50 Model performance …………………………………………………………………. 50 Most influential environmental variables ……………………………………. 50 Species richness …………………………………………………………………….. 51 Species Gains and Losses ………………………………………………………. 55 Species Turnover ……………………………………………………………………. 58 Range Changes ……………………………………………………………………… 59 Reptile species classifications ………………………………………………….. 65 Reptile records in Protected Areas …………………………………………… 66 Area prioritization for conservation ……………………………………………. 67 VII
Discussion …………………………………………………………………………………….. 106 Model performance ………………………………………………………………….. 106 Variables contributing to the models …………………………………………. 107 Species richness and turnover ………………………………………………….. 109 Range Changes & important species for conservation ………………… 112 Area prioritization for conservation & Protected Areas coverage ….. 121 The limitations of projecting into the future ………………………………… 126 Conclusion ……………………………………………………………………………… 127
References ……………………………………………………………………………………… 131
Appendices …………………………………………………………………………………….. 148
VIII
List of Tables
Table 1: The confusion matrix ……………………………………………………………. 9 Table 2: Some alternative criteria commonly used to convert probability distributions to binary forms (thresholded) …………………………………. 10 Table 3: Some measures of model accuracy …………………………………………. 11 Table 4: Estimates of relative contributions of the environmental variables to an example model …………………………………………………………………… 16 Table 5: A list of species used in this study (with the number of records for each species, their classification according to IUCN (global and national status), world status, and status in Egypt) …………………………………………… 33 Table 6: A list of 19 bioclimatic variables available at worldclim website …….. 36 Table 7: List of variables used to calculate VIF values …………………………….. 37 Table 8: Different scores of different parameters used to calculate relative species weight ………………………………………………………………………………. 48 Table 9: Mean and standard deviation of AUC values for each species ……… 71 Table 10: Species classification according to future species range change; assuming unlimited dispersal …………………………………………………. 88 Table 11: Species classification according to future species range change; assuming no-dispersal ………………………………………………………….. 90 Table 12: Number of species at each species range change category (assuming unlimited dispersal) ………………………………………………………………. 92 Table 13: Number of species at each species range change category (assuming no- dispersal) …………………………………………………………………………… 92 Table 14: Number of reptile species currently recorded or predicted and the number of predicted future species loss (UD and ND) or gain (UD) in each Protected Areas ………………………………………………………………….. 100
IX
List of Figures
Figure 1: Area under curve (AUC) ………………………………………………………… 12 Figure 2: The results of the jackknife test of variable importance ………………… 17 Figure 3: Response curves: how each environmental variable affects the Maxent prediction …………………………………………………………………………… 17 Figure 4: A map showing the outline of Egypt’s political boundaries overlain with the main cities and geographical locations used in this study……………… 30 Figure 5: A map showing the outline of Egypt’s political boundaries overlain with the Protected Areas …………………………………………………………………… 31 Figure 6: The distribution of Egyptian reptiles before and after 1950 ……………. 32 Figure 7: The distribution of all Egyptian reptile records and the number of records per grid square at a scale of a ¼ of a degree …………………………….. 32 Figure 8: The distribution of the weather stations used to interpolate the bioclimatic variables…………………………………………………………………………….. 36 Figure 9: Two examples of bio-layers variables excluded before calculating VIF statistics because they do not provide enough information to the model ………………………………………………………………………………………… 36 Figure 10: Calculation of future species gain or lost areas ………………………….. 43 Figure 11: Box-and-whiskers plot for mean AUC values across 75 studied species ………………………………………………………………………………………… 73 Figure 12: Frequency distribution of species mean AUC values showing the number of species at different ranges of mean AUC values …………………….. 73 Figure 13: The correlation between species mean AUC value and its predicted area of suitable habitats ……………………………………………………………….. 73 Figure 14: The correlation between species mean AUC value and its current extent of occurrence………………………………………………………………………. 74 Figure 15: The correlation between species mean AUC value and the number of its unique recorded points used to run the models ………………………….. 74 X
Figure 16: Relative contribution of environmental variables to the final model, represented by the mean value of average permutation importance across species………………………………………………………………………………. 75 Figure 17: The number of species at which each environmental variable was considered as the most influential environmental variable …………….. 75 Figure 18: Mean predicted reptile species richness using the summation of species predicted probability distributions (current and future – assuming unlimited dispersal) …………………………………………………………………………… 76 Figure 19: Future potential species richness change as a result of anthropogenic climate change (using species probability distributions – assuming unlimited dispersal) ………………………………………………………………. 77 Figure 20: Mean predicted reptile species richness using the summation of species thresholded predicted distributions (current and future - assuming unlimited dispersal) ………………………………………………………………. 78 Figure 21: Future potential species richness change as a result of anthropogenic climate change (using predicted species thresholded distributions – assuming unlimited dispersal) ………………………………………………… 79 Figure 22: Mean predicted reptile species richness using the summation of species thresholded predicted distributions (current and future - assuming no- dispersal) ………………………………………………………………………….. 80 Figure 23: Future potential species richness change (decline) as a result of anthropogenic climate change (using predicted species thresholded distributions – assuming no-dispersal) ……………………………………… 81 Figure 24: Potential future species loss as a result of anthropogenic climate change assuming both dispersal assumptions………………………………………. 82 Figure 25: Relative potential future species loss assuming both dispersal assumptions ……………………………………………………………………….. 83 Figure 26: Potential future species gain as a result of anthropogenic climate change assuming unlimited dispersal………………………………………………….. 84 Figure 27: Relative potential future species gain assuming unlimited dispersal .. 85 Figure 28: Future species turnover assuming unlimited dispersal …………………. 86 Figure 29: Future species turnover assuming no-dispersal …………………………. 87 XI
Figure 30: Number of species at each future species range change classification across global circulation models and the mean of different global circulation models (loss – unlimited dispersal) ……………………………. 93 Figure 31: Number of species at each future species range change classification across global circulation models and the mean of different global circulation models (gain – unlimited dispersal) …………………………… 94 Figure 32: Number of species at each future species range change classification across global circulation models and the mean of different global circulation models (loss – no-dispersal) ……………………………………. 95 Figure 33: Percentage of each future specie range change classification assuming unlimited dispersal and no-dispersal ………………………………………… 96 Figure 34: The overall pattern of future mean species range change under unlimited and no-dispersal assumptions ………………………………………………… 97 Figure 35: Average future range change across different taxonomic groups assuming either unlimited dispersal or no-dispersal assumptions ………………… 98 Figure 36: The correlation between number of recorded and predicted species per Protected Area ……………………………………………………………………. 99 Figure 37: The correlation between the area of the Protected Area (in 100 km 2 ) and either of the number of recorded or predicted species per Protected Area ………………………………………………………………………………………… 99 Figure 38: Current and future conservation prioritization ranked value (using Zonation algorithm - Additive benefit function) ……………………………. 101 Figure 39: Future change in conservation prioritization value (using Zonation algorithm - Additive benefit function) ………………………………………… 102 Figure 40: Current and future conservation prioritization ranked value (using Zonation algorithm – Core-Area function)………………………………….. 103 Figure 41: Future change in conservation prioritization value (using Zonation algorithm – Core-Area function) ………………………………………………. 104 Figure 42: Mean prioritization value (± 95% confidence limits - using Additive benefit function) across Protected Areas and non-Protected areas at current and future ………………………………………………………………………………… 105 XII
Figure 43: Mean prioritization value (± 95% confidence limits - using Core-Area function) across Protected Areas and non-Protected areas at current and future ………………………………………………………………………………… 105 Figure 44: The number of recorded amphibian and non-marine reptile species per a grid of half degree, comparing the results of (Baha El Din 2006a) to the results of this study ………………………………………………………………. 109 Figure 45: Accumulated species richness map of three taxonomic groups (butterflies, mammals and reptiles), showing areas of high species richness ……. 110 Figure 46: Maps showing actual distribution and suitable distribution areas for some Egyptian reptile species ……………………………………………………….. 128 Figure 47: Average MESS (Multivariate Environmental Similarity Surfaces) maps of different global circulation models showing areas of future novel climates ………………………………………………………………………………………… 130
XIII
List of Acronyms
AOO Area of Occupancy AUC Area Under Curve BioMAP Biodiversity Monitoring and Assessing Project EOO Extent of Occurrence GCM Global Circulation Models GIS Geographical Information Systems GPS Global Positioning System IUCN International Union for Conservation of Nature VIF Variance Inflation Factor
Environmental variabes: NDVI Normalized Difference Vegetation Index Bio1 Annual Mean Temperature Bio2 Mean Diurnal Range (Mean of monthly (max temp - min temp)) Bio3 Isothermality (Bi2/Bio7) (* 100) Bio4 Temperature Seasonality (standard deviation *100) Bio5 Max Temperature of Warmest Month Bio6 Min Temperature of Coldest Month Bio7 Temperature Annual Range (Bio5-Bio6) Bio8 Mean Temperature of Wettest Quarter Bio9 Mean Temperature of Driest Quarter Bio10 Mean Temperature of Warmest Quarter Bio11 Mean Temperature of Coldest Quarter Bio12 Annual Precipitation Bio13 Precipitation of Wettest Month Bio14 Precipitation of Driest Month Bio15 Precipitation Seasonality (Coefficient of Variation) Bio16 Precipitation of Wettest Quarter Bio17 Precipitation of Driest Quarter Bio18 Precipitation of Warmest Quarter Bio19 Precipitation of Coldest Quarter
- 1 - Introduction
Detailed information on the ecological and geographical distributions of species is essential for conservation planning and forecasting (Elith et al. 2006) especially for species facing conservation problems (Pineda & Lobo 2009). Species distribution modelling is one of many methods used to quantify patterns of species distributions and to extrapolate distributions across space and time (Elith & Leathwick 2007; Franklin 2009), usually based on a statistical model (Franklin 2009). This is done, basically, through a class of methods that combine known species observations (occurrence or abundance at known locations) with layers of environmental variables (and/or spatial characteristics of those locations) that are thought to have an influence on habitat suitability (and so species distribution) to make a model of the environmental conditions that meet the ecological requirements of a species, thus identifying where these suitable conditions are distributed in space (Phillips et al. 2006; Pearson 2007; Elith & Leathwick 2009; Franklin 2009; Warren & Seifert 2011). The output of most models aims to provide detailed predicted distribution maps (Elith et al. 2006). The earliest attempts at modelling species distributions using correlations between the observed distribution and climate were by Johnston (1924), who predicted the invasive spread of cactus in Australia, and Hintikka (1963), who assessed the climatic determinants of the distribution of some European species based on the minimum and maximum temperatures (Pearson & Dawson 2003; Guisan & Thuiller 2005). Recent years have seen an explosion of interest in species distribution modelling, with the publishing of hundreds of studies and governmental and non-governmental reports that use them (Franklin 2009). Species distribution models have been used in the literature under many different names including bioclimatic models, climate envelopes, species niche models, ecological niche models, niche-theory models, habitat models, resource selection functions, range maps and spatial models (Elith & Leathwick 2009; Franklin 2009). They can also be referred to as habitat suitability models, since they are said to describe the suitability of the habitat for a particular species (Hirzel & Le Lay 2008; Franklin 2009).
- 2 - They represent an approximation of the ecological niche by estimating the fundamental (physiological or potential) niche, realized (ecological or actual) niche or, when based only on climate variables, the “climatic niche” (Phillips et al. 2006; Franklin 2009). According to Hutchinson (1957), the fundamental niche of a species is the set of all conditions that allow long-term survival and reproduction, also called an “n-dimensional hypervolume” because of the multiple axes of the defining conditions. The realized niche, on the other hand, is the portion of the fundamental niche that a species actually occupies and from which the species is not excluded due to biotic interactions (Pulliam 2000; Phillips et al. 2006; Pearson 2007). The realized niche of a species is a subset of the fundamental niche, usually but not always due to human disturbance, biotic interactions (competition and predation), or the existence of geographical barriers that have prevented the dispersal and colonization of the species (Phillips et al. 2006; Franklin 2009). According to Hutchinson’s definition of the realized niche, and assuming that the records do not come from a sink habitat (where populations cannot be maintained without immigrants from elsewhere (Franklin 2009)), the occurrence records where a species has been recorded (and subsequently the environmental characteristics of these locations) represent a sample of the realized niche (Phillips et al. 2006). Using that sample together with one of the various possible algorithms, we can estimate the realized niche and the empirical correlation between distribution and environmental variables (Phillips et al. 2006; Franklin 2009). In reality, the predicted distribution does not fully reflect the requirements and realized niche of the species because of various factors not incorporated into the model (such as biotic interactions, geographic barriers and species history). Because of this, distribution models should be interpreted with caution (Pearson & Dawson 2003; Pearson et al. 2007) . Recently, species distribution models have developed into innovative and valuable techniques used to generate biogeographical information applicable to various fields including conservation biology, ecological restoration, biodiversity assessment, reserve planning and design, evolution, resource management and habitat management and restoration (Phillips et al. 2006; Pearson 2007; Franklin 2009). In poorly surveyed areas, they can be used to direct additional surveys or discover new species (Raxworthy et al.
- 3 - 2003). They have proven themselves to be an important component in predicting the potential anthropogenic effects on biodiversity (Guisan & Thuiller 2005) and assessing the invasive potential of non-native species (Elith et al. 2006). Another growing application is to predict the distributions of harmful pests and disease hosts and vectors that affect humans, plants, and animals, providing information on the potential distribution or outbreaks of these pathogens and thus supporting public health and epidemiology (Franklin
- (examples include: Kelly & Meentemeyer 2002; Levine et al. 2004; Saathoff et al. 2005; Peterson 2006; Zeilhofer et al. 2007; Meentemeyer et al. 2011). Here I first review the way in which species distribution models use data and the choice of algorithm, and then look at ways of validating such models. I then consider in more detail the algorithm that I chose to use, called MaxEnt, before reviewing the ways in which distribution models have been used to predict future changes in biodiversity under the assumptions of models of climate change. I end with a section about the relevance of this work to the Egyptian fauna together with the very few previous studies of the impacts of climate change on Egypt’s biodiversity.
Issues about the data required for distribution models Species distribution models require two sorts of data input: biological data describing the current known species distribution and predictor variables describing the environmental conditions thought to affect species distribution (Pearson 2007; Phillips & Dudik 2008). These two data input components are usually in the format suitable for visualizing on a Geographic Information System (GIS). The biological data usually come in the form of geo-databases containing the georeferenced records of the occurrences of species, while the predictor variables usually come in the form of GIS raster grids. The most common predictor variables are those related to climate (e.g. temperature, precipitation), topography (e.g. elevation, aspect, slope), soil type and land cover type (Phillips et al. 2006). Some studies also use variables describing the distribution of interacting species to improve model accuracy (Newbold 2010). Predictor variables come into two formats: continuous (any value within
- 4 - a certain range; e.g. altitude, temperature or precipitation) or categorical (only a limited number of discrete values; e.g. habitat, vegetation type, land cover and soil) (Phillips et al. 2006; Phillips & Dudik 2008). In the context of species distribution models, there are two types of biological data, either presence-only or presence/absence data. Presence-only data represent only where the species has actually been collected or recorded: they do not provide information on where it was not recorded. In contrast, presence-absence data, in addition to presence records, indicate areas where the species was not recorded during surveys. Presence-only data constitute the majority of existing records (Phillips et al. 2006; Elith & Leathwick 2007), and are somewhat easier to collect. The main sources of presence-only data are those extracted from museum collections, herbaria, personal collections, planned surveys, casual observations, published articles and reports, and online databases (e.g. the Global Biodiversity Information Facility, see: http://www.gbif.org/). In recent years, data from many museum and herbaria collections have become available online over the internet (Graham et al. 2004; Newbold 2010). Most of these occurrence records were recorded without systematic planning, and collection methods are rarely known (Elith et al. 2006). Most museum databases, for example, give information on where the specimens were collected, but usually not on the location of surveys that failed to record a particular species (Stockwell & Peters 1999). These data, when used with caution, can give invaluable information on patterns of distribution (Newbold 2010) and be effective in calibrating distribution models for many species and regions (Elith et al. 2006). Presence-absence records, on the other hand, are rarely available and are often difficult to obtain accurately, especially in poorly surveyed areas where modelling may hold greatest value (Hirzel et al. 2002; Anderson et al. 2003; Phillips et al. 2006; Pearson 2007). Absence records may be unreliable or of questionable value in many situations (Phillips et al. 2006; Pearson 2007): failure to detect a particular species may simply be due to inadequate collecting effort (Anderson 2003; Graham et al. 2004), or when the target species can be easily missed during the surveys (rare and highly mobile species) (Pearson et al. 2007; Rödder et al. 2011). These cases are often called ‘false absences’, and if used as input, the distribution model will
- 5 - interpret the predictor values of these locations as unsuitable environmental conditions, even though this is not true (Pearson 2007). This can cause serious bias in the model analyses, so absence records should be used with caution (Hirzel et al. 2002; Pearson 2007). There are some other potential sources of bias and error associated with biological data that should be carefully considered when collating records (Pearson 2007). For example, there can be error through incorrect identification of species (Graham et al. 2004; Pearson 2007; Newbold 2010). Incorrect identification of specimens from museums and collections can be detected by re-examining the original specimens (Newbold 2010). There is also a certain degree of uncertainty and error with spatial referencing of samples. Historic data, for example, often have location descriptions that represent only a coarse estimate of the true location, in contrast to more recent records from the last 20 years many of which have been georeferenced with GPS technology with location precision down to 1 m (Reside et al. 2011). Other errors include transcribing mistakes while transferring data from field sheets to electronic databases (some of which are correctable by data cleaning) and transliterating site names from local language into Latin script (especially from particular languages such as Arabic, which has no official method of transliteration into English: every writer performs a new transliteration as if from scratch (Gilbert & Zalat 2008)). Georeferencing the records can introduce further errors; the textual description of the location may be very far from precise, describing a very broad or vague area (e.g. Egypt or Western desert) or include an ill-defined offset (e.g. 15 km NE of Wadi Feiran). In such cases there are additional sources of uncertainty: errors with measuring the extent of the area name, the direction and the measure of distance (Wieczorek et al. 2004; Graham et al. 2008; Newbold 2010). In certain situations, the collector may not have provided enough details of the recording sites, and often there can be several sites with the same name in the study area. For example, there are two widely separated areas in Egypt named Gebel El-Ahmar (in South Sinai and Giza governorate): mistakenly locating a record in the wrong location has the potential to cause great error in the model, especially if dealing with endemic species or species with restricted
- 6 - ranges. In such cases, the safest way is to ignore the record, unless more details can be found that establish which site is correct. Presence-only records are usually highly non-random in space and time, especially those derived from casual observations or Natural History museums and herbaria (Franklin 2009). This introduces another source of bias to the model, of which there are four types: spatial, environmental, temporal and taxonomic (Soberón et al. 2000; Newbold 2010). Records are often collected closer to cities, roads, rivers, and coasts (Soberón et al. 2000; Reddy & Dávalos 2003; Kadmon et al. 2004; Newbold 2010) than a truly random sample. They tend also to be close to the homes of the active recorders (Dennis & Thomas 2000; Newbold 2010) or in areas that are of high interest, such as Protected Areas or biodiversity hotspots (Newbold 2010). The records may also show spatial correlation (if collected from several nearby localities in a restricted area) (Phillips et al. 2006). Collectors also are more likely to focus on larger, easy-to-identify or charismatic organisms, causing substantial bias in sampling towards flowering plants and vertebrates (Newbold 2010).
The choice of model algorithms A wide variety of modelling algorithms has been used recently (Guisan & Thuiller 2005; Pearson 2007). Some methods are based on statistical regression methods (e.g. generalized linear models, generalized additive models, multivariate adaptive regression splines), whilst others depend on machine-learning methods (decision trees, artificial neural networks, genetic algorithms, maximum entropy and support vector machines) (Pearson 2007; Franklin 2009). Some of these algorithms have been developed in user- friendly free software (Pearson 2007) (e.g. Maxent). Algorithms that include the possibility of interactions between predictors are considered more suitable (Elith et al. 2006; Pearson 2007). Some studies have founded that different modelling algorithms can potentially produce different predictions (Pearson 2007; Pearson et al. 2007; Wisz et al. 2008), and that the best performing model is not always the same for all species (Luoto et al. 2005; Grenouillet et al. 2011), so it is important to select the most appropriate method for the species being studied (Pearson et al. 2007).
- 7 - Distribution-model algorithms vary in their data requirements. Many require both presence and absence records (e.g. GLM), while others can be carried out without absence records (e.g. BIOCLIM, DOMAIN, Maxent) (Pearson 2007). For presence-only models, instead of using real absence records (especially in poorly surveyed areas where absent records are usually not available), the model is applied by sampling pseudo-absences from the background study area (Pearson 2007; Pearson et al. 2007). Model algorithms also vary in their output formats; some are continuous (yielding a probability of occurrence between 0 and 1), while others give a binary prediction (suitable / unsuitable or presence / absence) (Pearson 2007). In general, predictions calibrated with fewer records are unlikely to be as good as those based on large numbers (Wisz et al. 2008; Franklin 2009) because when the sample size is small, outliers give more weight to the analysis than if a greater number of records compensates for their effect. Larger numbers of records can describe complex relationships and requirements (Wisz et al. 2008). There is no minimum fixed number of records required to run an acceptable model (Franklin 2009); some studies have found that 50-100 records are enough to produce an acceptable model, while some methods, (e.g. Maxent) seem to give reasonable prediction performance when calibrated with many fewer records (Wisz et al. 2008; Franklin 2009) (see below).
Assessing model performance Assessing model performance is a vital step in model development (Pearson 2007). It helps to determine the suitability of the model for particular applications, and to compare different models (Pearson 2007). Nevertheless, model performance is just one aspect of model evaluation, and other criteria should be considered, such as ecological realism, spatial pattern of error, and model credibility (acceptability to the user community) (Franklin 2009). It is preferable to use new independent data in model validation (Pearson 2007; Pearson et al. 2007; Franklin 2009). Sometimes this is done by collecting new independent data from different areas (e.g. Newbold et al. 2010), spatial resolution, or time period, or by using data from other surveys
- 8 -
(Pearson 2007). Using the same data for running and evaluating the model
results in overestimating the predictive performance (Franklin 2009). However,
it is often not feasible or cost-effective to collect new independent data for
validation (Franklin 2009). In this situation it is very common to divide the data
into two sets prior to the running the model, a training (calibration) set and a
testing (evaluation) set. The partitions can be either at random or spatially
(Pearson 2007; Franklin 2009). The training set is used to build the model,
while the testing set is used for model validation (Franklin 2009). The relative
sizes of the two sets is somewhat arbitrary and depend on the number of
record locations, although using 75% for training and 25% for testing has
become common (Pearson 2007).
An alternative to a one-time split is to split the data into partitions many
times; this is included in cross-validation, subsampling, and bootstrapping
methods. In cross-validation, the occurrence records are randomly split into a
number of equal-sized sets (>2) and the model is calibrated by leaving out
each set in turn, which is then used for evaluation (Pearson 2007; Phillips &
AT&T Research 2011). The validation statistic can be calculated as the mean
of the replicated models. A big advantage of cross-validation over one-time
splitting of the data is that it uses all of the data for validation (Phillips & AT&T
Research 2011). For rare species where the species has just a few
occurrence records (<20), an extreme form of cross-validation is
recommended, using ‘jackknifing’ (Pearson 2007), where the model is
repeatedly run omitting each record in turn, with the omitted record used for
testing (Baldwin 2009). Jackknifing can be used with larger sample sizes, but
creates an over-optimistic estimate of predictive power (Baldwin 2009).
Another alternative is to use repeated subsamples, in which the data are
repeatedly split randomly into training and testing subsets without replacement
(Phillips & AT&T Research 2011). In bootstrapping, the training data are
randomly selected by sampling with replacement for multiple times (the same
record is used in testing more than once) (Pearson 2007; Phillips & AT&T
Research 2011).
Several test statistics have been used to measure model performance, often derived from the presence/absence confusion matrix (Table 1) (Pearson et al. 2007). The confusion matrix requires binary model predictions (i.e. - 9 - presence/absence), not continuous prediction (Pearson 2007; Franklin 2009). The confusion matrix uses the frequencies of each of the four possibilities: true positive, where the model predicts a species as present and the data confirms it; false positive, where the model predicts a species as present and the data shows absence; false negative, where the model predicts a species as absent and the data shows present; and true negative, where the model predicts a species as absent and the data also show absence (Pearson 2007).
Table 1: The confusion matrix
Observed Present Absent Predicted Present True Positive (TP) False Positive (FP - Commission) Absent False Negative (FN - Omission) True Negative (TN)
It is common in distribution modelling to convert from continuous output (probability of occurrence) to categorical (presence/absence or suitable/non- suitable), conventionally done by using a threshold value (Franklin 2009). Subsequently, so-called ’threshold-dependent’ measures can be used to evaluate models, or alternatively continuous probabilistic predictions can also be evaluated, so-called ’threshold-independent’ measures (Franklin 2009). The confusion matrix, and statistics used to test model performance derived from it, requires binary predictions (presence/absence). Thus it is often necessary to convert the probabilities obtained from continuous models to binary predictions by using this threshold (Pearson 2007). Various alternative methods have been employed to select the best value for the threshold (see Table 2 for some examples). The simplest is to use a fixed arbitrary value, above which the species is deemed present; by convention this threshold is set to 0.5 (Pearson 2007; Franklin 2009). However, this method is the worst and lacks any ecological justification or reasoning (Liu et al. 2005; Pearson 2007). Liu et al. (2005) evaluated the use of different threshold criteria, reporting that five threshold criteria performed equally well and better than the others: observed prevalence, average predicted probability, the sum of sensitivity and specificity, sensitivity specificity quality approach, and the point on the ROC plot nearest the upper left corner.
- 10 - For presence-only models, some studies favored using “lowest predicted threshold”, which equals the lowest predicted value of any of the presence records used to run the model (Pearson et al. 2007); this sometimes called minimum training presence. It is a conservative threshold that can be interpreted ecologically (Pearson et al. 2007), but because it predicts that all training records are correctly predicted, this may yield over-prediction (Jarnevich & Reynolds 2011). A more conservative threshold can be used, such as the value above which 90% of the training records are correctly classified (10 percentile training presence value) (Jarnevich & Reynolds 2011); this rejects 10% of the training records with lowest predicted probability as unsuitable, considered appropriate since they may represent recording errors, migrants or ephemeral populations (Morueta-Holme et al. 2010).
Table 2: Some alternative criteria commonly used to convert probability distributions to binary forms (thresholded).
Fixed threshold: e.g. 0.5
Sensitivity= Specificity
Maximum Sensitivity plus Specificity
Maximum Kappa
Maximum percent correct classification (PCC)
Predicted prevalence = observed prevalence
observed prevalence
mean or median predicted probability
minimizing the distance between the ROC curve and the upper left corner of
the ROC plot
Weighting omission and commission errors by their costs relative to a given
application of the model
Maximum Sensitivity (Specificity) that also achieves user-defined minimum
Specificity (Sensitivity)
Of the various ways of measuring model accuracy (Table 3), one simple and easy way is to calculate the percent correct classification, by dividing the sum of the diagonals of the confusion matrix (true positives and true negatives) by the number of the observations (Pearson 2007; Franklin 2009). Although its concept is easy and logic, there are two problems with it: first, it does not distinguish between false negatives and false positives; second, it is possible to get high accuracy using a poor model when a species’ prevalence (proportion or frequency) is relatively high or low (Pearson 2007; Franklin 2009). To overcome this problem, a similar measure of accuracy has been used, called Kappa, which is considered as a measure of categorical agreement that describes the difference between the observed agreement and chance agreement with the proportion of correct predictions expected by
- 11 - chance taken into account (Pearson 2007; Franklin 2009). Kappa, along with AUC (see below), is more suitable to evaluate models in poorly surveyed regions, where absence data are not available (Pearson et al. 2007). The ‘true skill statistic’ is another method of measuring model accuracy, suggested as an alternative to kappa if a threshold-dependent measure is needed (Franklin 2009): it is reported to be independent of the species prevalence and so considered more appropriate than kappa (Baldwin 2009).
Table 3: Some measures of model accuracy
Measure Calculation Sensitivity (true positive fraction)
( + )
False negative rate (1 – Sensitivity) Specificity
False positive rate (1 – Specificity) Model accuracy (% correct) ( + )
Positive predictive power
+
Odds ratio ( ) ( )
Kappa [( + ) − (( + )( + ) + ( + )( + )) ⁄ )] [ − (( + )( + ) + ( + )( + ) ⁄ )]
True Skill Statistic (TSS) sensitivity + specificity - 1
When model output is continuous and a particular threshold measure is used to convert it to categorical (binary) output, the statistics derived from the confusion matrix will be sensitive to the threshold method used (Pearson 2007). It would be useful to have a single measure of model performance that is independent of the threshold choice (Phillips et al. 2006); such measures could then be used to compare modelling methods, species, candidate predictors, etc. (Franklin 2009). One of the most frequently used such statistics in species distribution modelling is the AUC - the Area Under the ROC (receiver operating characteristic) Curve (Elith et al. 2006; Elith & Leathwick 2007). The AUC is derived from the receiver operating characteristic (ROC) curve (Baldwin 2009). The ROC curve is defined by plotting the false-positive error rate (1 - specificity) on the x-axis versus the true positive rate (sensitivity)
- 12 - on the y-axis across a range of possible threshold values (Fig. 1) (Pearson 2007; Franklin 2009). The AUC value can be interpreted as the probability that when a presence site and absence site are randomly chosen, the former will have a higher predicted value (Elith et al. 2006; Elith & Leathwick 2007; Franklin 2009). An AUC value of 0.8 means that there is a probability of 80% that a randomly selected presence record has a greater predicted value than a randomly selected absence (Pearson 2007; Wisz et al. 2008). The AUC value is calculating by measuring the area under the ROC curve, and ranges from 0 to 1. An AUC score of 1 indicates a perfect model i.e. perfect discrimination between presences and absences; a model with AUC of 0.5 indicates a discriminating ability no better than random; an AUC value <0.5 indicates the model performs worse than random, i.e. the model may fit the modelling data but it predicts badly (Elith et al. 2006; Elith & Leathwick 2007; Pearson 2007; Franklin 2009). A model of AUC score of 0.5 to 0.7 has been used as indication of poor performance, 0.7 to 0.9 is moderate, and >0.9 is high (Franklin 2009). The AUC is not influenced by species prevalence, and therefore is reliable for model comparisons (Phillips et al. 2006; Franklin 2009); such comparisons are valid only between models and sample sizes of the same species and study area (Wisz et al. 2008).
Fig. 1: Area under curve (AUC)
- 13 - In cases where only presence data are available, almost all the measures discussed above can be applied by testing the ability of the model to discriminate accurately between presence and background (also called pseudo-absence) data, although they should be interpreted differently (Franklin 2009). In such cases, AUC, for example, could be interpreted as the probability of the model scoring a random presence site higher than a random pseudo-absence or background site (Franklin 2009). All methods defined from the confusion matrix (including kappa and AUC), are flawed and are unsuitable to be used when only presence data are available (Boyce et al. 2002; Pearson et al. 2007). AUC, for example, is not an absolute measure and is sensitive to the method used to determine the absence data during the evaluation (Wisz et al. 2008) and may often give poor model performance because of low classification success (Boyce et al. 2002). Its value tend to be higher for species with narrower ranges relative to the study area, which does not necessarily mean that the model is better (Phillips & AT&T Research 2011). In statistical modelling the usual method of assessing the model fit is the Akaike Information Criterion, or AIC. This strikes a balance between fitting the data as well as possible (which can always be improved by using more predictor variables), and parismony (which is taken to mean the fewest number of predictors). It is used to arrive at the best set of predictors from a panel of possibilities, refining an individual model, but comparing models with different response variables cannot be done. Thus here the use of AIC is not very useful.
Maximum entropy modelling (Maxent) Maxent is a general-purpose machine-learning method for making inferences or predictions from incomplete information (Phillips et al. 2006; Baldwin 2009). It estimates the most uniform distribution (maximum entropy) subject to a set of constraints that represent our incomplete information about the target distribution (Phillips et al. 2006). It is being applied in a variety of fields including finance and astronomy (Franklin 2009). Since 2004 it has been applied extensively to model species distributions because it shows high predictive accuracy and enjoys several additional attractive properties (Phillips
- 14 - & Dudik 2008; Franklin 2009). It has been used in a variety of applications including predicting species richness (Graham & Hijmans 2006; Pineda & Lobo 2009), invasive species (Ficetola et al. 2007; Ward 2007), estimating species extent of occurrence (Sergio et al. 2007), and the quality of protection of rare species (DeMatteo & Loiselle 2008; Thorn et al. 2009). Maxent can produce three output formats (raw, cumulative and logistic), but the logistic format improves model calibration and so is highly recommended over the two other formats: it provides an estimate of probability of presence as predicted by the environmental variables, and thus large differences in the values correspond to large differences in habitat suitability (Phillips & Dudik 2008; Baldwin 2009). The logistic format provides output values ranged from 0 to 1 and is easier to interpret when projected to Geographic Information System (GIS) (Baldwin 2009). All the three types of the output formats are monotonically related but are scaled differently and have different interpretations (Phillips & AT&T Research 2011); all pixels will be ranked in the same order, resulting in identical performance when a rank- based statistic is used (e.g. AUC), but will vary in predictive performance when using a measure that depends on the actual output value (e.g. Pearson’s correlation) (Phillips & Dudik 2008; Baldwin 2009). Several studies have shown that Maxent performs well in comparison with alternative algorithms and shows higher predictive accuracy (Elith et al. 2006; Hernandez et al. 2006; Pearson et al. 2007; Wisz et al. 2008; Franklin 2009; Pineda & Lobo 2009). According to Phillips et al. (2006), the main advantages of Maxent are that it requires only presence records along with predictor variables for the whole study area; it is able to use both continuous and categorical environmental data (Baldwin 2009); it can fit interactions between different predictor variables; it is based on a concise mathematical definition; over-fitting can be avoided by using standard methods (called regularization); prediction output is continuous; and it can also be applied to presence/absence data by using conditional models. Maxent has been shown to be very robust to small numbers of training records and outperforms other algorithms when using a relatively small number of occurrence records (Wisz et al. 2008; Franklin 2009). It has been reported as producing accurate predictions using as few as 20-30 records (Feeley & Silman 2011); in some
- 15 - cases as few as five records can calibrate a model, but such models should be interpreted with great caution and be used to point out areas where future surveys should focus (Baldwin 2009). It is also robust against moderate location errors accompanying species records, outperforming other algorithms (Graham et al. 2008). In some studies that compare the accuracy of different algorithms, Maxent was shown to outperform GARP, especially with small sample sizes (Franklin 2009). In Elith et al. (2006) comprehensive study that compares the accuracy of a large number of presence-only algorithms, Maxent was among the top performers and showed high predictive accuracy (Franklin 2009). It also outperformed GLM and GAM when applied to presence/background data, and was much better than BIOCLIM (Franklin 2009). Maxent has some drawbacks, however. It is not as mature a statistical method as GLM and GAM, and hence special-purpose software is required because it is not available in standard statistical packages (Phillips et al. 2006). Maxent is disposed to overfitting (matching the input data too closely), yielding predicted distributions with high values clumped around the occurrence points. This has a detrimental effect on model performance (Phillips & Dudik 2008; Baldwin 2009). In order to surmount this issue, a number of ‘regularization’ parameters have been added to recent versions of Maxent (Phillips & Dudik 2008; Baldwin 2009). There are various different settings that can be adjusted by the modeller, but recent studies have shown that the default settings work perfectly well for a wide range of species, environmental conditions, number of available records and level of sample bias, achieving a performance just as good as the models that result from adjusted settings (Phillips & Dudik 2008; Baldwin 2009). It is important to know how each variable influences the model predictions, and which has the greatest influence (Baldwin 2009). Maxent provides two measures of variable importance. First, it estimates the contribution of each variable using either: percent contribution and permutation importance (Table 4). Percent contribution shows how each variable contribute to the model, based on the particular path selected by the algorithm to get the optimal solution (different algorithms can get the same solution using different paths, resulting in having different percent contribution values) (Phillips &
- 16 - AT&T Research 2011). Permutation importance depends only on the final model, not the path used to get it. This is calculated by randomly permuting variable values among presence and background points and measuring the resulting decline in training AUC, high AUC decline indicates high dependency of the model of that variable (Phillips & AT&T Research 2011). Permutation importance is more favourable to estimate variables importance as it does not depend on the path used by the algorithm (Songer et al. 2012); although both values should be interpreted with caution because of collinearity - when using strongly correlated environmental variables, a greater importance can easily be attributed to one of two or more highly correlated variables (Baldwin 2009; Phillips & AT&T Research 2011). The second approach is the jackknife test (Fig. 2), done by repeatedly running the model excluding each variable in turn, as well as models using each variable in isolation and all variables together (Baldwin 2009; Phillips & AT&T Research 2011). This provides information on the relative strength of each variable, how accurately each is able to explain the distribution and how much unique information is provided by it (Yost et al. 2008; Baldwin 2009). Maxent also provides response curves describing how each variable influences the probability of occurrence, keeping all other variables constant at their average value (Baldwin 2009). These response curves are also highly affected by strong collinearity among predictors (Baldwin 2009) (Fig. 3).
Table 4: Estimates of relative contributions of the environmental variables to an example model
Variable Percent contribution Permutation importance bio19 31.2 9.6 bio6 19.8 18.9 bio2 12.1 1.8 bio9 11.2 6.3 bio13 8 5.2 bio7 3.6 0 bio18 3.2 1.4 bio16 2.6 3.3 bio4 2 25.3 bio11 1.3 0.4 bio5 1.3 16 bio8 1.1 1.8 bio1 1 0.2 bio10 0.8 8.1 bio3 0.7 1.5 bio12 0.1 0 bio17 0.1 0 bio15 0 0 bio14 0 0
- 17 -
Fig. 2: The results of the jackknife test of variable importance.
Fig. 3: Response curves: how each environmental variable affects the Maxent prediction.
- 18 - Climate change and biodiversity Over the last century, changes of Earth’s climate have been recorded, including warmer temperatures (0.6 °C increase over the 20 th century) accompanied with altered geographical and seasonal distribution of precipitation (Araújo & Rahbek 2006; Thuiller 2007; Alkemade et al. 2011). It is expected that climate will undergo a continuous increase in temperature of between 1 and 6 °C during the 21 st century (Thuiller 2007; Alkemade et al. 2011). There is a widespread agreement that climate change has high impact on the survival of ecological communities and individuals (Suarez & Tsutsui 2004; Alkemade et al. 2011); and that biodiversity is continually being transformed in response to it (Hannah et al. 2005). Even though recorded effects of climate change on biodiversity seem to be generally slower than other factors, it is predicted that its effects will become increasingly prominent over the next 50 years and beyond (Thuiller 2007). The combination of habitat fragmentation and climate change is the most threatening aspect of climate change impacts on biodiversity and species conservation (Hannah et al. 2005). As climate changes, species (or populations) will be able to survive in novel environments if the new environmental conditions are within the species’ fundamental niches, or if species are able to adapt to these conditions if they are outside of its fundamental niche (Martínez-Meyer 2005). In other words, the ability of a species to respond to climate change will be dependent on its ability to track climate changes, colonize new areas and modify its physiology and seasonal behavior to adapt to novel environmental conditions (Thuiller 2007). Responses of different species to climate change can be categorized into three non-exclusive axes: time (e.g. phenology), space (e.g. range), and ‘self’ (e.g. physiology) (Bellard et al. 2012). Climate change has been implicated in the last 40 years as the main cause for species’ distribution shifts and the extinction (Thomas et al. 2004), with a particularly strong impact on butterflies, birds and species at high altitude (Hannah 2011). Some climate change model forecasts claim that 15-37% of the current species are committed to extinction by 2050 (without taking in consideration biological factors; e.g. competition and evolutionary history) (Thuiller 2007). Expected
- 19 - species range shifts are generally polewards or towards higher altitudes, with each temperature increase of 1 °C predicted to cause a move of ecological zone by 160 km polewards or 0.16 km higher altitude (Thuiller 2007). Over the past 50 years, the range of terrestrial plants and animals in the northern hemisphere has been shifted on average by 6.1 km northward (or 6.1 m upwards) each decade, causing remarkable increases in species diversity in some places in Europe over the last century (Thuiller 2007). Many European birds and butterflies, for example, have shifted their distributions northwards by about 20 km and 150 km respectively from 1970 to 1990 (Alkemade et al. 2011). Range shifts of flowering plants and pollinating insect species due to climate change will cause possible mismatches between plant and pollinator populations; coupled with increased extinction risks to either of them, this will result in changes to insect-pollinator networks (Bellard et al. 2012), potentially causing dramatic changes to ecosystem services. Other interspecific relationships are also susceptible to such modifications (competition, predation, parasitism and mutualism) which will modify community structure and ecosystem functions (Bellard et al. 2012). Range shifts of introduced pest and pathogenic species due to climate change may have even more dramatic impacts on ecosystems, conservation and human life (Hannah 2011). Climate change has also resulted in changes to the timing of seasonal events of some species e.g. earlier egg-laying for birds (Crick et al. 1997; Hughes 2000; Suarez & Tsutsui 2004), bird migration, reproduction, planting and harvesting, fruiting and flowering times (Ibanez et al. 2006; Parmesan 2006). Several extinction incidents recorded in the recent past have been claimed as the results of climate change. The first documented extinction linked to climate change occurred in 1987 when the formerly common Golden Toad (Bufo periglenes) from Costa Rica disappeared (Hannah 2011). As a result of increasing temperature, it is expected that species turnover will increase and the proportion of the range of species that will remain suitable under climate change will decrease in all biomes (Alkemade et al. 2011). Species distribution models have been widely used to estimate the potential impacts of climate change on species distributions and ecosystems (Coetzee et al. 2009; Franklin 2009) and predict potential future extinction risks. Once a model has been calibrated for current climate conditions, it can
- 20 - be projected to predict the potential distributions at different time periods (in the future or the past) by using information on predicted climates, or to different study areas in order to assess the potential locations where invasions are more likely to establish (Franklin 2009). This helps to manage species facing possible future threats by identifying biological corridors for dispersal, determining sites for re-introduction and areas requires more protection measures (Thuiller 2007). Several studies have used species distribution models to show the effect of climate change on the whole fauna and flora; such predictions are challenging tasks because this involves sources of uncertainty: in the data, the resolution, which algorithm is used (different algorithms may produce very different future projections even if they give similar present-day models (Martínez-Meyer 2005)), and the global circulation model (GCM) used to create predicted climate scenarios (Carvalho et al. 2010; Guisan & Rahbek 2011). Validation of the projected results is problematic, because predictions are made for events that have not yet occurred (Araújo & Pearson 2005). Validation can be done by projecting recent past distributions of some species to current conditions, using new records as independent data for evaluation, or by projecting current distributions back to the distant past (e.g. Pleistocene climates) and using fossil records as independent data for evaluation (Araújo & Pearson 2005; Thuiller 2007). In most cases, the availability of such data is very limited to certain geographical areas or taxonomic groups. For these reasons, results from such projection studies should be treated with great caution. It is assumed by most distribution models (projected in time or space) that the climate conditions in current and projected models are analogous. When this assumption is violated, by extrapolating to conditions beyond those of the training data (non-analogous climates), inaccuracies can result, with either over-prediction or under-prediction (Fitzpatrick et al. 2008; Richmond et al. 2010). A possible solution is to limit predictions in areas with novel climate conditions by applying so-called ‘clamping’ (Richmond et al. 2010). Clamping has become available in recent versions of Maxent, allowing the identification of the degree of uncertainty in projected models and showing areas where the model is less reliable because of extrapolation (Rödder et al. 2009; Holcombe et al. 2010). Using clamping in Maxent results in a constant response outside
- 21 -
the maximum and minimum limits of the training data, by constraining the
climate to remain at the limits of the training data (Phillips et al. 2006; Elith et
al. 2010; Elith et al. 2011).
Forecast results using different model algorithms can vary quite a lot: fundamentally these models depend on correlations between environment and distribution, and thus are dependent on the data and the mathematical functions used to predict species distributions (Araújo & New 2007). In addition, studies have shown that results derived from different species distribution modelling methods are not equally reliable for all species and that the best performing model is not always the same for all species (Luoto et al. 2005; Grenouillet et al. 2011). To reduce the effect of inter-model variation, some researchers recommend the simultaneous use of different prediction methods (and climate change models and emission scenarios) within a consensus modelling framework (ensemble or consensus modelling), which they claim produces more robust inference and prediction (Araújo & New 2007; Coetzee et al. 2009; Grenouillet et al. 2011; Yen et al. 2011). The term consensus refers to an agreement of different model outputs (Marmion et al. 2009). Using ensemble modelling helps to reduce the predictive uncertainty of a single model and potentially to improve the robustness of the projections (Araújo & New 2007; Grenouillet et al. 2011). It may also help to reduce the effect of model uncertainty and improve predictions for rare species (Guisan & Rahbek 2011). Ensemble modelling has been recently used in broad-scale conservation studies, especially to check the impacts of climate change on different species (Marmion et al. 2009). BIOMOD (Thuiller et al. 2009) is one of the most-used platforms for ensemble modelling. It is a free Cran-R (R Development Core Team 2012) package that enables users to perform species distribution models using several modelling techniques (including Maxent in most recent versions) and testing methods, including projecting the models into predicted future environmental conditions (Thuiller et al. 2009).
Relevance to Egypt Predicted impacts of climate change on Egypt include the raising of the sea level (which will affect people living in Nile Delta and coastal areas in Egypt
22 - and also will make high percentage of Egypt flora and fauna vulnerable to extinction), changes in the distribution of vector-borne infectious diseases, and reduction in the productivity of major crops (Parry et al. 2007; Tolba & Saab 2009). It also predicted that Egypt will be vulnerable to water stress and increase of water requirements, uncertainty of Nile flow, and increase in irrigation demand under climate change (Parry et al. 2007). The water issue is a serious problem facing Egypt in the future because the country is mostly arid and depends on the Nile as the main source of drinking and irrigation water.
Tourism, one of the main sources of Egypt National Income, is also predicted to be affected in the future by climate change (Tolba & Saab 2009). The number of tourists coming to Egypt’s coasts each year is expected to decline because of the potential impact of climate change on coral reefs. Worldwide, almost all coral reefs have been affected by climate-change- induced coral bleaching at one time or another (Hannah 2011). Corals depend on a certain kind of algae (zooxanthellae), using the nutrients they produce while providing the algae with physical support, protection and maintenance in adequate level of sunlight for photosynthesis (Hannah 2011). When exposed to high water temperatures (more than 1 or 2° C rise in sea surface temperature above normal summer maximal temperatures for a period longer than 3–5 weeks), corals expel the algae, resulting in loss of coral color (Hannah et al. 2005; Hannah 2011). Beside its conservation implications, declines in the number of tourists will have great impact on Egypt National Income and a relatively high proportion of Egyptians working in tourism will suffer.
Climate change will potentially affect biodiversity and species composition of Egypt ecosystems (Tolba & Saab 2009), although not enough studies or data are available on this (see below). It is predicted that Egypt will suffer from global changes in the distribution of flowering plants and pollinating insects due to climate change, which are predicted to cause dramatic declines in the ecosystem services they provide. We do have an estimate of the potential costs of such declines: the annual cost of losing Egyptian pollinators to the Egyptian National Income would be approximately L.E. 13.5 billion ($2.4 billion, 3.3% of the 2003 GDP) (Brading et al. 2009).23 - Only a handful of studies have been conducted with the use of species distribution modelling techniques to predict the potential distribution of Egyptian fauna and the potential impacts of climate chance on them: there are no such published studies on Egyptian flora. This may be because that the models are relatively new and the availability of biodiversity data records of Egyptian fauna and flora are not well organized and sparse. Gilbert & Zalat (2008); Basuony et al. (2010), the main two publication of the BioMAP project (see: http://biomapegypt.org), discussed the distribution of each Egyptian butterfly and mammal species respectively. In these two books, data on each species were collated from available sources and an actual and predicted distribution map for each species were provided (using Maxent); each species was also assessed according to the IUCN guidelines and criteria. In another study, El Alqamy et al. (2010) used Maxent to predict the potential distribution of the Nubian Ibex (Capra nubiana) in South Sinai, and showed that the presence of water was the environmental factor most influential in their distribution in South Sinai. Three studies have discussed the effect of climate change on Egyptian species. Hoyle & James (2005) used an occupancy model (a type of population viability analysis) to assess the potential impacts of global warming on the world’s smallest butterfly, the Sinai Baton Blue (Pseudophilotes sinaicus). Just two recent studies have used species distribution models to assess the effect of climate change on Egypt biodiversity. Soultan (2011) used Maxent to test the potential impact of climate change on the distribution of Egyptian antelopes (Barbary sheep Ammotragus lervia, Nubian ibex Capra nubiana, Dorcas gazelle Gazella dorcas, and Slender-horned gazelle Gazella leptoceros) using the A2 and B2 emission scenarios of a Global Circulation Models. Leach (2011) discussed the effect of climate change on Egyptian butterflies and mammals (using Maxent algorithm and A2 and B2 emission scenarios of a different Global Circulation Models), and considered the effectiveness of the Protected Area network in Egypt in conserving Egypt biodiversity under current and future climates (using Zonation software). No studies have been published on the use of species distribution models to predict the potential distribution of Egyptian reptiles - the topic of this study.
24 - Aim of study
The main aims of this study are to: prepare a distribution map of the records for each reptile species; predict their potential distribution under the current climate; predict their future potential distribution under climate change using four global circulation models, two emission scenarios, three time slices and two assumptions about dispersal ability; estimate the pattern of species richness under current and future climates; estimate areas predicted to have highest future gains / losses / turnover of species; analyse the responses to climate change and assess future threat status for each species; assess current status according to IUCN guidelines and criteria; assess the priority areas for conservation of Egyptian reptiles; perform a gap analysis to show the effectiveness of Egypt’s Protected Areas network to conserve reptiles.
- 25 - Methods
Study area – Egypt Egypt is located at the north eastern part of the continent of Africa; it is situated between latitudes 22° to 32° N and longitudes 24° to 37° E. It occupies an area of a little more than 1,000 kilometers square (1 million km 2 ), constituting about 3 per cent of Africa (Zahran & Willis 2009 - See Fig. 4 for the political boundaries of Egypt showing the main locations discussed in this study). Geographically, it can be divided into four units: the Nile Valley and Delta, Western Desert, Eastern Desert, and Sinai. Most of Egypt’s human population lives around the Nile and Delta because of the restricted availability of water and food elsewhere, which leaves a large proportion of Egypt’s land as desolate desert. Relatively small settlements are sparsely distributed in Western Desert oases, the Red Sea coast, and Sinai. Egypt is one of if not the most arid country in the world; average annual rainfall across Egypt is just 10 mm with a maximum of 200 mm at the Mediterranean coast (Zahran & Willis 2009). There are 30 Protected Areas in Egypt, compromising about 15% of the total area of Egypt (Fig. 5).
Study species – Egyptian reptiles According to (Baha El Din 2006a), the most recent comprehensive publication on the Egyptian herpetofauna, the contemporary Egyptian reptiles include at least 109 species: 61 lizards, 39 snakes, 1 crocodile, 7 turtles and a tortoise; in addition there is a recently separated species, Acanthodactylus aegyptius (Baha El Din 2007). Data on species distribution were compiled from various different sources. The main source was records extracted from the BioMAP project database [Biodiversity Monitoring and Assessment Project 2004-2008; see: http://www.biomapegypt.org/]; one of BioMAP’s main aims was to develop a comprehensive database of existing Egyptian biodiversity records derived mainly from international museum records, recent literature, and personal collections. For Egyptian reptiles, the collections were mainly from two well-known Egyptian herpetologists, Dr. Sherif Baha El Din and Dr.
- 26 - Mostafa Saleh. In addition to BioMAP database records, I included some further records from the sparse literature (a full list of the published literature used is given in Appendix 1). I also involved my personal sightings from fieldwork surveys done in Sinai, Gebel Elba, Nile Delta, and Eastern desert (2005-2011). All these records were gathered into one preliminary database. Records were then assessed based on various criteria (mainly taxonomic and geographic). Species taxonomy was revised according to latest taxonomic findings (Baha El Din 2006a), and records with invalid unrecognizable taxonomy were deleted. Some records came from outside Egypt and some non-Egyptian species had erroneously been included in the database. Records for marine or Nile species (Nile crocodile and Sea Turtles) were also excluded from the database because the lack of GIS predictor layers for aquatic environments meant that these species could not be involved in species distribution modelling. For recent records coming with global positioning systems (GPS) receiver coordinates, sighting coordinates were double-checked to avoid possible uncertainties over the coordinate system used (Newbold 2009), errors accompanied with transferring information from GPS devices or field sheets to electronic databases or with unintentional swapping between latitude and longitude. All other records were then georeferenced to the nearest 5 decimal places (when possible) using topographic maps, gazetteers, and Google Earth®. Records with vague location names (e.g. records with just Egypt, Eastern Desert, Western Desert, Sinai, South Sinai, etc.) could not be georeferenced precisely and so were deleted from the database. Some locations were not able to be georeferenced, i.e. very small locations or locations with local names that could not be identified using available topographic maps and gazetteers (e.g. small wadis or villages). These records were deleted from the database. All valid georeferenced records were then mapped using ArcMap 10 (ESRI, USA) and a current distribution map were prepared for each species (not shown). Current species distributions were then checked and compared to those from (Baha El Din 2006a). Records from odd locations (outside the distribution range) were assigned as inaccurate and deleted from the database (especially for old museum records).
- 27 - Only species recorded from 8 unique pixels or more (at the resolution of 2.5 arc minutes; see below) were processed further with species distribution models to avoid high over-fitting as a result of using a very small number of species occurrences with Maxent (Baldwin 2009). Twenty-eight terrestrial reptile species were excluded from this study on this basis (see Appendix 2). More records for these species are required in order to be able to assess their potential response to climate change, particularly urgent since many are either endemics/near-endemics or with restricted ranges. This results in having a final database of 12,188 records representing 75 species (49 lizards, 25 snakes, and 1 tortoise - the species list and number of valid records for each are given in Table 5). The collection dates of the records were found to range from 1821 to 2011, with an apparent increase in the recording effort from 1950 onwards (Fig. 6). The coverage of the records is good, covering most of Egypt’s landscape and habitats (Fig. 7a). Bias in recording effort (represented by the number of valid records) was checked across a 0.25º grid, showing high bias towards the main cities and populated areas (Fig. 7b). Unsurprisingly, the highest collection effort was found around the greater Cairo district, followed by South Sinai (the St Katherine area), the Alexandria area, some areas around Fayoum and Wadi El-Natrun and small patches near El-Arish and Mersa Matruh.
Environmental predictor variables
Climate data for the near past (1950-2000) was downloaded from WorldClim
Global Climate Data v1.4 (release 3 - see: http://www.worldclim.org) (Hijmans
et al. 2005). These data were considered to indicate the current climate
conditions. The Worldclim website provides a set of global environmental
layers (at different resolutions) in the form of 19 climate variables (bio-layers:
see Table 6) derived from precipitation and temperature records. Bio-layers
were clipped to the boundaries of Egypt using Clip tool in ArcMap 10 (ESRI,
USA). The resolution of 2.5 arc-minutes (~5 km square) was chosen to run the
distribution models because it is appropriate to the level of uncertainty that
accompanies the museum records (which form the majority of the records),
and because the climate data for Egypt were interpolated using relatively few
weather stations largely concentrated in the Nile Valley and Delta (Newbold
- 28 - 2009 - Fig. 8), creating more uncertainty. A special study may be needed to validate the reliability of bio-layers derived from relatively few weather stations in species distribution modelling, but this will need independent field data (Martínez-Meyer 2005). A further benefit of running the models at a resolution of 2.5 arc-minutes instead of the more detailed 30 arc-seconds is not only that this reduces run times and storage space, but it also is thought to minimise the effect of ignoring species interactions on modelled distributions, which can not be managed in correlative models: Pearson & Dawson (2003) argued that the impact of biological interactions on distribution models is minimized by constructing models at macro-scales, where the influence of environmental variables on species distributions dominate. Elevation data were obtained from the SRTM Digital Elevation Database v4.1 [available at: http://www.cgiar-csi.org/data/elevation], which is available in tiles covering the globe. The tiles that cover Egypt were downloaded at 90-m resolution, merged together using the Mosaic tool, clipped to the boundaries of Egypt, and rescaled to be of the same resolution of other layers (2.5 arc minutes) using ArcMap 10 (ESRI, USA). An Egyptian geological habitat map was one of the products of the BioMAP project (A. A. Hassan, unpublished data), and was used here. In this map, the habitat of Egypt is classified into 11 classes (sea, littoral coastal land, cultivated land, sand dune, wadi, metamorphic rock, igneous rock, gravels, serir sand sheets, sabkhas and sedimentary rocks) based on existing maps, remote sensing and extensive ground-truthing (Newbold et al. 2009a). The layer was available in vector format, and was converted to ascii raster format at the same resolution of other variables (2.5 arc minutes). The normalized-difference vegetation index (NDVI) is a remote-sensing product indicating the greenness of vegetation; it is used to monitor the vegetation condition of the landscape, and to discriminate between vegetation and non-vegetation areas. It has therefore often been used as a correlate of faunal species occurrence and diversity in species distribution modelling (Leyequien et al. 2007; Peterson et al. 2011), including studies of reptiles (Rashid 2007; Costa et al. 2010; Taheri 2010; de Pous et al. 2011; Wooten & Gibbs 2012). In this study, NDVI data for seven years (from Jan 2004 to Dec
- were downloaded from the SPOT Vegetation website (see:
29 - http://free.vgt.vito.be/). They are provided as 10-day synthetic maps at a resolution of 1 km, and a total of 252 maps were obtained representing data from 2004 to 2010. The maximum and minimum value of each pixel was calculated across the full set of maps. Two layers were derived from and used as predictors in the models: maximum NDVI value (indicating how much vegetation there is per pixel), and the difference between maximum and minimum NDVI value (indicating the degree of fluctuation in vegetation per pixel). The layers were clipped to the boundaries of Egypt, and re-scaled to 2.5 arc-minutes resolution. In order to reduce the negative effect of having high correlations between predictor variables on model performance and the over-fitting resulting from using many variables, the Variance Inflation Factor (VIF) statistic was calculated among the continuous variables as a measure of multicollinearity. The VIF statistic has been widely used (with other statistical alternatives) as a measure of collinearity between continuous variables in order to prune predictors before use (Farren et al. 2010; Fernández-Moya et al. 2010; Bombi & D’Amen 2011; Bombi et al. 2012).
Four bio-layers were excluded prior to VIF calculations because they show only minor variability in their values across Egypt (see Fig. 9) and hence do not provide valuable information to the models. The excluded layers were: Bio7 (temperature annual range), Bio14 (precipitation of driest month), Bio17 (precipitation of driest quarter), and Bio18 (precipitation of warmest quarter). In variable Bio14, for example, almost all pixels were shown to have 0 value except just 5 pixels that have a value of 1 (as shown in Fig. 9). This explicitly reflects how arid Egypt is. Using these variables will not provide useful information to the models and may just add extra noise, so they were excluded before calculating the VIF statistic.30 -
1 The Mediterranean Sea 25 Assiut 49 Ras El-Hekma
2 The Suez Gulf 26 Hurghada 50 Port-Said
3 The Aqaba Gulf 27 Ras Mohamed 51 Rafah
4 The Red Sea 28 Sharm El-Sheikh 52 Mersa Matruh
5 The Nile Delta 29 El-Minia 53 Rosetta
6 Lake Nasser 30 El-Tur 54 Damietta
7 Lake Brullus 31 Ras Gharib 55 Sallum
8 Lake Bardawil 32 Dahab 56 Sidi Barrani
9 Lake Manzala 33 Saint-Katherine 57 Kharga oasis
10 Lake Idku 34 Nuweiba 58 Dakhla oasis
11 Lake Mariut 35 Abu Zneima 59 Farafra oasis
12 Lake Qarun 36 Beni Suef 60 Bahariya oasis
13 Halayeb 37 Ras Zaafarana 61 Siwa oasis
14 Abu Ramad 38 Fayoum 62 Gebel Elba area
15 Al-Shalatein 39 Taba 63 El-Gilf El-Kebir
16 Berenice 40 Ain Sukhna 64 Gebel Abraq area
17 Aswan 41 Suez 65 Gebel El-Gallala El-Qibliya
18 Edfu 42 The greater Cairo 66 Gebel El-Gallala El-Bahariya
19 Mersa Alam 43 Wadi El-Natrun 67 Qattara Depression
20 Luxor 44 Ismailia 68 Gebel Yillaq
21 El-Quseir 45 El-Alamein 69 El-Hassana
22 Qena 46 El-Dabaa 70 Gebel El-Hallal
23 Sohag 47 El-Arish 71 Gebel El-Maghara
24 Safaga 48 Alexandria 72 Tiran & Sanafir islands
Fig. 4: A map showing the outline of Egypt’s political boundaries overlain with the main cities and geographical locations used in this study.
- 31 -
No. Protectorate Name Declaration Date Area Km² Governorate 1 Ras Mohamed National Park 1983 850 South Sinai 2 Zaranik Protectorate 1985 230 North Sinai 3 Ahrash Protectorate 1985 8 North Sinai 4 El-Omayed Protectorate 1986 700 Matrouh 5 Elba National Park 1986 35600 Red Sea 6 Saluga and Ghazal Protectorate 1986 0.5 Aswan 7 St. Katherine National Park 1988 4250 South Sinai 8 Ashtum El-Gamil Protectorate 1988 180 Port Said 9 Lake Qarun Protectorate 1989 250 El Fayoum 10 Wadi El-Rayan Protectorate 1989 1225 El Fayoum 11 Wadi Allaqi Protectorate 1989 30000 Aswan 12 Wadi El-Assuti Protectorate 1989 35 Assuit 13 El Hassana Dome Protectorate 1989 1 Giza 14 Petrified Forest Protectorate 1989 7 Cairo 15 Sannur Cave Protectorate 1992 12 Beni Suef 16 Nabq Protectorate 1992 600 South Sinai 17 Abu Galum Protectorate 1992 500 South Sinai 18 Taba Protectorate 1998 3595 South Sinai 19 Lake Burullus Protectorate 1998 460 Kafr El Sheikh 20 Nile Islands Protectorates * 1998 160 All Governorates on the Nile 21 Wadi Degla Protectorate 1999 60 Cairo 22 Siwa 2002 7800 Matrouh 23 White Desert 2002 3010 Matrouh 24 Wadi El-Gemal/Hamata 2003 7450 Red Sea 25 Red Sea Northern Islands * 2006 1991 Red Sea 26 El-Gilf El-Kebir 2007 48523 New Valley 27 El-Dababya 2007 1 Qena 28 El-Salum Gulf 2010 383 Matrouh 29 El-Wahat El-Bahreya 2010 109 6th October 30 Mount Kamel Meteor Protectorate 2012 1 New Valley
Fig. 5: A map showing the outline of Egypt’s political boundaries overlain with the Protected Areas. Protected Areas with * symbols are not shown in the map.
- 32 -
Fig. 6: The distribution of Egyptian reptiles before 1950 (left) and after 1950 (right).
(a) (b) Fig. 7: The distribution of all Egyptian reptile records (a) and the number of records per grid square at a scale of a ¼ of a degree (b).
- 33 - Table 5: A list of species used in this study (with the number of records for each species, their classification according to IUCN guidelines and criteria (global and national status), and distribution status worldwide and in Egypt (see later)).
N Species # records Global IUCN Egypt National IUCN World status Egypt Status 1 Cyrtopodion scabrum 37 LC LC Narrow Widespread 2 Hemidactylus flaviviridis 31 NA VU (D2) Narrow Narrow 3 Hemidactylus robustus 40 NA VU (D2) Narrow Narrow 4 Hemidactylus turcicus 217 LC LC Widespread Widespread 5 Pristurus flavipunctatus 80 NA VU (D2) Narrow Narrow 6 Ptyodactylus guttatus 115 NA LC Narrow Narrow 7 Ptyodactylus hasselquistii 225 NA LC Narrow Widespread 8 Ptyodactylus siphonorhina 180 NA LC Restricted Widespread 9 Stenodactylus mauritanicus 35 NA VU (D2) Restricted localized 10 Stenodactylus petrii 60 NA LC Narrow Widespread 11 Stenodactylus sthenodactylus 268 NA LC Narrow Widespread 12 Tarentola annularis 324 NA LC Narrow Widespread 13 Tarentola mauritanica 342 LC LC Widespread Narrow 14 Tarentola mindiae 43 LC VU (D2) Near- Endemic Narrow 15 Tropiocolotes bisharicus 20 NA VU (D2) Endemic Narrow 16 Tropiocolotes nattereri 34 NA LC Narrow Narrow 17 Tropiocolotes steudneri 197 NA LC Narrow Widespread 18 Tropiocolotes tripolitanus 24 LC LC Narrow Narrow 19 Agama spinosa 113 LC LC Narrow Narrow 20 Laudakia stellio 458 NA LC Narrow Narrow 21 Pseudotrapelus sinaitus 117 NA LC Narrow Widespread 22 Trapelus mutabilis 299 NA LC Narrow Widespread 23 Trapelus pallidus 173 NA LC Narrow Widespread 24 Trapelus savignii 86 VU (A2abcd) VU (D2) Near- Endemic Narrow
34 - N Species # records Global IUCN Egypt National IUCN World status Egypt Status 25 Uromastyx aegyptia 82 NA LC Narrow Widespread 26 Uromastyx ocellata 56 LC EN (B2 a,b i) Narrow Narrow 27 Uromastyx ornata 16 NA VU (D2) Restricted localized 28 Chamaeleo africanus 72 NA EN (B2 a,b i,iv) Narrow Narrow 29 Chamaeleo chamaeleon 262 NA LC Widespread Narrow 30 Acanthodactylus aegyptius 167 NA LC Near- Endemic Widespread 31 Acanthodactylus boskianus 1414 NA LC Narrow Widespread 32 Acanthodactylus longipes 50 NA VU (D2) Narrow Widespread 33 Acanthodactylus pardalis 191 VU - (A2c; B1ab (i,ii,iii)) VU (D2) Restricted Narrow 34 Acanthodactylus scutellatus 406 NA LC Narrow Widespread 35 Mesalina bahaeldini 98 LC VU (D2) Endemic localized 36 Mesalina guttulata 216 NA LC Narrow Widespread 37 Mesalina olivieri 172 NA LC Narrow Widespread 38 Mesalina pasteuri 19 NA VU (D2) Narrow localized 39 Mesalina rubropunctata 129 NA LC Narrow Widespread 40 Ophisops occidentalis 28 LC VU (D2) Restricted localized 41 Varanus griseus 141 NA LC Narrow Widespread 42 Varanus niloticus 24 NA VU (D2) narrow localized 43 Chalcides cf. humilis 37 NA VU (D2) Narrow Widespread 44 Chalcides ocellatus 596 NA LC Widespread Widespread 45 Eumeces schneiderii 188 NA LC Narrow Narrow 46 Scincus scincus 376 NA LC Narrow Widespread 47 Sphenops sepsoides 367 LC LC Restricted Widespread 48 Trachylepis quinquetaeniata 318 NA LC Narrow Widespread 49 Trachylepis vittata 17 LC VU (D2) Narrow Narrow 50 Leptotyphlops cairi 218 NA EN (B2 a,b i) Narrow Narrow
35 - N Species # records Global IUCN Egypt National IUCN World status Egypt Status 51 Leptotyphlops macrorhynchus 17 NA VU (D2) Narrow Narrow 52 Eryx colubrinus 51 NA VU (D2) Narrow Narrow 53 Eryx jaculus 44 NA LC Widespread Narrow 54 Eirenis coronella 22 NA VU (D2) Narrow localized 55 Lytorhynchus diadema 144 NA LC Narrow Widespread 56 Macroprotodon cucullatus 53 LC VU (D2) Widespread Narrow 57 Malpolon moilensis 51 NA LC Narrow Widespread 58 Malpolon monspessulanus 170 LC LC Widespread Narrow 59 Natrix tessellata 96 LC VU (D2) Widespread localized 60 Platyceps florulentus 127 LC LC Narrow localized 61 Platyceps rogersi 45 NA LC Narrow Widespread 62 Platyceps saharicus 34 NA LC Narrow Narrow 63 Psammophis aegyptius 183 NA LC Narrow Widespread 64 Psammophis schokari 371 NA LC Narrow Widespread 65 Psammophis sibilans 283 LC LC Narrow Narrow 66 Spalerosophis diadema 216 NA LC Widespread Widespread 67 Telescopus dhara 56 NA LC Narrow Narrow 68 Naja haje 60 NA LC Narrow Narrow 69 Naja nubiae 14 NA VU (D2) Restricted Narrow 70 Walterinnesia aegyptia 17 NA VU (D2) Restricted Narrow 71 Cerastes cerastes 233 NA LC Narrow Widespread 72 Cerastes vipera 551 NA LC Narrow Widespread 73 Echis coloratus 50 NA LC Narrow Widespread 74 Echis pyramidum 59 NA LC Narrow Widespread 75 Testudo kleinmanni 63 CE (A2 abcd + 3d) VU (D2) Near- Endemic Narrow
36 - Table 6: A list of 19 bioclimatic variables available from the worldclim website
Bio1 Annual mean temperature Bio2 Mean diurnal range (mean of monthly (max temp - min temp)) Bio3 Isothermality (Bio2/Bio7) (* 100) Bio4 Temperature seasonality (standard deviation *100) Bio5 Maximum temperature of the warmest month Bio6 Minimum temperature of coldest month Bio7 Temperature annual range (Bio5-Bio6) Bio8 Mean temperature of the wettest quarter Bio9 Mean temperature of the driest quarter Bio10 Mean temperature of the warmest quarter Bio11 Mean temperature of the coldest quarter Bio12 Annual precipitation Bio13 Precipitation of the wettest month Bio14 Precipitation of the driest month Bio15 Precipitation seasonality (coefficient of variation) Bio16 Precipitation of the wettest quarter Bio17 Precipitation of the driest quarter Bio18 Precipitation of the warmest quarter Bio19 Precipitation of the coldest quarter
Fig. 8: The distribution of the weather stations used to interpolate the bioclimatic variables: the number of weather stations in Egypt is clearly relatively few.
Fig. 9: Two examples of bio-layers variables excluded before calculating VIF statistics because they do not provide enough information to the model.
- 37 - All the rest of the continuous variables (altitude, NDVI maximum value, difference between NDVI maximum and minimum values, and 15 bio-layers – see: Table 7) were involved in the collinearity test. VIF calculations were done using R software v2.15 (‘car’ package - R Development Core Team 2012); the variable with the highest VIF score was removed first and new VIF values calculated between the remaining variables, a process repeated until all yielded VIF values below 10. The resulting variables (the grey shaded rows in Table 7) were then used in the Maxent modelling.
Table 7: List of variables used to calculate VIF values; rows shaded with grey show variables with VIF values less than 10 and so used to run the models.
Altitude Altitude NDVI_Max NDVI maximum value NDVI_Difference Absolute difference between the highest and lowest NDVI values Bio1 Annual mean temperature Bio2 Mean diurnal range (mean of monthly (max temp - min temp)) Bio3 Isothermality (Bio2/Bio7) (* 100) Bio4 Temperature seasonality (standard deviation *100) Bio5 Maximum temperature of the warmest month Bio6 Minimum temperature of the coldest month Bio8 Mean temperature of the wettest quarter Bio9 Mean temperature of the driest quarter Bio10 Mean temperature of the warmest quarter Bio11 Mean temperature of the coldest quarter Bio12 Annual precipitation Bio13 Precipitation of the wettest month Bio15 Precipitation seasonality (coefficient of Variation) Bio16 Precipitation of the wettest quarter Bio19 Precipitation of the coldest quarter
To estimate the potential impact of climate change on Egypt biodiversity (represented here by reptiles), current distribution models were projected into the future. This was done for three time slices (2020, 2050, and 2080). Future climate data (IPCC 4th assessment data - IPCC 2007) were downloaded from the International Centre for Tropical Agriculture website (see http://www.ccafs- climate.org/). In this study, four Global Circulation Models (GCMs) were used at each time slice to minimise the effect of model type and model calculation method on the final results, giving an average overall trend of the potential impacts of climate change (i.e. some elements of ensemble modelling). The four GCMs used were the Hadley Centre Coupled Model, version 3 (HadCM3), produced by the Hadley Centre for Climate Prediction and Research (UK), the second-generation coupled global climate model produced by the Canadian Centre for Climate Modelling and Analysis (CGCM2-
- 38 -
CCCma), a model produced by the Commonwealth Scientific and Industrial
Research Organization in Australia (CSIRO Mk2) and a model produced by
the Japanese Centre for Climate System Research (NIES99). These GCMs
have been frequently used in species distribution modelling studies that
involve climate change impacts on biodiversity (Araújo et al. 2006; Mika et al.
2008; Holt et al. 2009; Rödder 2009; Rödder & Weinsheimer 2009; Buisson et
al. 2010; Fouquet et al. 2010; Brito et al. 2011; Hu & Jiang 2011; Nori et al.
2011; Sanchez et al. 2011; Wilms et al. 2011). In this study, possible
evolutionary and/or phenological responses to climate change were not
considered, so I assume that species will try to track their suitable habitat
(depending on dispersal ability) rather than accommodate to the new
conditions. The fossil record shows little evidence of species evolutionary
response to past climate changes; during the Pleistocene glaciation, for
example, the fauna and flora were subjected to climate change 5-10 times
stronger than that of the 20
th
century, and the main response was to track the
changed climate (Maiorano et al. 2011).
For each GCM model and time slice, two emission scenarios (A2a and B2a) were used, derived from the Intergovernmental Panel on Climate Change (IPCC) Special Report: these are the most commonly used scenarios in climate-change assessments (Hannah 2011). They reflect two different assumptions about the levels of CO 2 emissions, linked to assumed demographic changes and socio-economic and technological developments (Marini et al. 2009). In the A2a scenario, it is assumed that CO 2 emission rate will be unrestrictedly doubled by 2050 (medium to high emission rates - “Business as usual” scenario) due to a highly heterogeneous future world characterized by high population growth rate, increased land-use changes, and not much technological improvement; while in the B2a scenario, it is assumed that CO 2 emission rates will remain unchanged or even decrease by 2050 (medium to low emission rates - “moderate” scenario) due to an environmentally conscious future world characterized by slower population growth rate and land-use changes, and more technological innovations (Sauer et al. 2011; Saupe et al. 2011; Taubmann et al. 2011). Sets of future climate layers equivalent to those used in the current-distribution models were prepared to be used in future projections. We have no idea how NDVI and - 39 - habitat variables will alter in the future, and so I assumed in the future models that these variables will not alter; a further predictor variable will also not alter, altitude.
Species distribution modelling
In this study, Maxent software v3.3.3k (Phillips et al. 2004; Phillips et al. 2006 -
see: http://www.cs.princeton.edu/~schapire/maxent/) was chosen to run the
models, for reasons explained above. Ten replicated runs with cross-validation
were made for each species (except species distributed in less than 10 unique
pixels ‘at the resolution of 2.5 arc minutes’: in these cases the number of
replicates was set automatically to the number of unique points). This was
done by randomly dividing the records into 10% sections, and then each run
used 90% of the data (training), leaving 10% for testing. This was repeated ten
times, each with a different 10% of the data for testing. This method gets the
best use of all the data for validation, especially if there are not many records
(Phillips & AT&T Research 2011). This also obtains a more stable model
performance to estimate the potential distribution, and minimises the effect of
possible errors and bias in species records. The habitat map (categorical) and
all non-collinear continuous variables (grey-shaded rows in Table 7) were
used to run the models. The mean (± SD) AUC values of the ten replicated
runs are given in Table 9.
Default Maxent settings have been shown to have the potential of
achieving a performance as good as if they were tuned on the evaluation data
itself (Phillips & Dudik 2008). Default settings were used to run the models,
with just one exception: the maximum number of iterations was set to 1000, to
be sure of allowing the algorithm enough time to converge (Phillips et al.
2006). The logistic output format was chosen, i.e. the probability of
occurrence, ranging from 0 to 1. From the cross-validation runs, ten raster
ASCII files were produced for each species, together with the overall mean
probability distribution. For each species, the most influential variable was
determined as the variable with the highest mean permutation importance
across model runs. Mean contribution value of each variable across all
- 40 - species was also calculated to indicate the most influential variable across all Egyptian reptile species. In order to convert the Maxent probabilities of occurrence into predicted presence/absence (or, more precisely, suitable/non-suitable habitat), the form required for further analyses, the 10 th -percentile training presence threshold was used (following: Pearson et al. 2007). This threshold is determined by assuming that 10% of the training points which have the lowest predicted values were not precisely located, either because they were erroneously georeferenced, are migrants, or are ephemeral populations (Morueta-Holme et al. 2010). A pixel is assigned to indicate presence (suitable) if it has a predicted value more than the lowest 10% of the predicted values of training records. For each species, ten thresholded distribution maps were created by Maxent from the ten probability maps. Maxent does not automatically provide an overall presence/absence map for each species, so this was created manually by assigning presence to a pixel if it has presence values in more than half of the model runs (>5 runs). This was done using the Raster Calculator tool in ArcMap 10 (ESRI, USA). The current predicted distributions (750 model runs for 75 species) were then projected into the future to assess the potential impacts of climate change on Egyptian reptiles. This was done for three future time slices (2020, 2050, and 2080), four GCM models (HadCM3, CCCma, CSIRO, and NIES99) and two emission scenarios (A2a and A2b); giving a total of 24 possible future projections. The average impact of different GCMs was calculated to see the overall effect of climate change on Egyptian reptiles, regardless of which model was used. This gave a total of six mean future analysis sets (3 time slices X 2 emission scenarios). For each species, each current predicted distribution run was projected into the future using 24 possible future projections; this produces 240 future projections for each species (10 replicated runs for each species were projected into 24 possible future projections), overall 18,000 future projections for the 75 species. For each future projection, two map sets were created: the potential future probability and thresholded (suitable/non-suitable) distributions.
- 41 - As for current predictions, the means of ten projected replicated runs was calculated, producing 24 possible future distributions for each species. Thresholded future distributions made by Maxent were based on the assumption that species have the ability to disperse freely to newly suitable areas, considered unrealistic for at least some species (Peterson et al. 2002). Giving that information on the dispersal ability of most reptile species is very limited (Cadby et al. 2010; Edgar et al. 2010), two extreme assumptions were used in the analyses: unlimited dispersal and no-dispersal, to allow a full range of possible outcomes - both assumptions are commonly used in the literature. The true response should lie somewhere in between, depending on the species’ ability to track future climate change (Maiorano et al. 2011), although using both assumptions and ignoring the effect of biotic interactions on distributions has been criticized (Araújo & Peterson 2012). For the ‘unlimited dispersal’ assumption, species were assumed to have the ability to move to newly suitable habitats without restriction or giving attention to any barriers. For the ‘no dispersal’ assumption, species were assumed to be incapable of moving to newly suitable areas: if the current occupied area became unsuitable in the future, the species was assumed to become extinct in that area. No account was taken of any interactions among species, such as competition and predation (Peterson et al. 2002), because there is no information about any such interactions to guide the modelling. The average future thresholded distributions of replicated runs were not created automatically by Maxent; they were calculated using the Raster Calculator and Reclassify tools in ArcMap 10 (ESRI, USA). For the unlimited- dispersal assumption, future thresholded distribution maps were used directly to calculate the average future thresholded distributions; each pixel was assigned as a presence (suitable) if it was predicted to be potentially suitable in more than half of the replicated runs (>5); this resulted in having 24 potential future thresholded distribution maps for each species. For the no-dispersal assumption, the resulting thresholded distribution maps were not directly used, but were processed first using ArcMap 10 (ESRI, USA) in such a way as to assign a pixel to be a presence if it had presence value in both the current and future projection. The mean overall thresholded distributions for no-dispersal replicated runs were calculated as in the case of unlimited dispersal.
- 42 - The mean future predictions of the four GCMs were calculated as well; this was done for both probability and thresholded distributions. For probability distributions, a mean of the four global circulation models was calculated, yielding just six mean future probability maps (3 time slices X 2 emission scenarios). Thresholded distributions were done using both dispersal assumptions: a pixel was assigned to be potentially suitable if it had a value of presence in more than two GCMs, this gave six overall thresholded future projections per species (for each of unlimited and no dispersal assumptions).
Comparisons across species Current and future predicted species richness maps were calculated in two different ways: first, by simply adding together the average probability distribution maps of all species (assuming future unlimited dispersal); and second, by adding together the thresholded distribution maps (assuming future unlimited- and no-dispersal assumptions). For current species richness, two maps were created using either probability or thresholded distributions. Future species richness maps were calculated for each of the 24 possible future projections, using either probability (unlimited dispersal) or thresholded (unlimited and no dispersal) distributions. The overall means of future species richness maps across the four GCMs were also calculated using either probability (unlimited dispersal) or thresholded (unlimited and no-dispersal) distributions. Future potential changes in species richness were also calculated by subtracting current species-richness from future species-richness maps. This does not take into consideration the potential future changes in species composition, but just gives an indication of which areas will have an altered (increased or decreased) numbers of species. This resulted in having 24 species richness changes maps either using either probability distributions (unlimited dispersal) or thresholded distributions (either unlimited or no- dispersal). I calculated the average future changes in species richness across the four GCMs. Gains and losses in future species distributions were calculated using current and future thresholded distributions. The current thresholded
- 43 - distribution of each species was reclassified (using the Reclassify tool in ArcMap 10), giving a value of 3 to presence pixels and 0 to absence pixels. For each species, values of every possible future thresholded distribution were added. In the resulting map, a pixel with a value of 4 indicated that this pixel was maintained suitable in the future; 0 indicated that this pixel was maintained unsuitable in the future; 3 indicated that the pixel lost its suitability for the species in this area (unlimited and no dispersal); and 1 indicated that the pixel gained suitability for this species (unlimited dispersal) (Fig. 10). Gains and losses for each species were exported separately to new GIS files. The losses across all the 24 future potential distributions across all species were added together into a species loss map showing which areas are predicted to suffer most in losing species (unlimited and no-dispersal). The data can be expressed as a percentage of the species richness of a pixel. The same was carried out performed for gains in suitability to produce a species gain map to showing which areas are predicted to gain much species (unlimited dispersal), which can be interpretable as potential refugee for the reptile species. A mean value across GCMs of total lost or gained suitability maps were also calculated.
(a) Current distribution (b) Future distribution
Fig. 10: Calculation of future species gain or lost areas
Species turnover, an index of dissimilarity between current and future species composition (Thuiller 2004) was calculated. It is defined as the net
44 - change in the number of species in a particular area (Broennimann et al. 2006). Species turnover is often considered a good measure of community composition change, ecosystem disturbance and the potential impacts of climate change from regional to continental scales (Thuiller 2004; Broennimann et al. 2006). Areas with small turnover values (close to 0) indicate that the species assemblage at these areas is predicted to remain unchanged in the future (no species loss or gain); while areas with a high turnover values (close to 100) indicate that the species assemblage at these areas is predicted to be completely different in the future (i.e. all species occupying the area are predicted to be lost/replaced in the future) (Thuiller 2004; Broennimann et al. 2006). For each future projection, and assuming both dispersal assumptions, species turnover was calculated as the following (following: Peterson et al. 2002; Thuiller et al. 2005; Broennimann et al. 2006): (unlimited dispersal) = 100 ∗
+
+(no dispersal) = 100 ∗
Where SL is the number of potentially lost species, SG is the number of potentially gained species, and SR is the current species richness.
In order to assess the future extinction risk of Egyptian reptiles as a result of climate change, and to determine which species may require more protection in the future, species range changes were calculated: the percentage loss (or gain) in suitable habitats (unlimited- and no-dispersal assumptions). This was calculated by counting the number of suitable pixels for each species in current and future maps, and calculating the average percentage of loss (or gain) in the number of pixels. Each species was then classified into one of the following categories (at each of 24 possible future projections and a mean classification among different GCMs): Extinct (loss of the entire suitable habitat - 100%), Critically Endangered (loss >80%), Endangered (loss 50-80%), Vulnerable (loss 30-50%), Least Concern (loss <30%), gain 1 (gain <30%), gain 2 (gain 30-50%), gain 3 (gain 50-80%), gain
- 45 - 4 (gain 80-100%), and gain 5 (gain >100) (Modified from: Thuiller et al. 2005; Levinsky et al. 2007; Fitzpatrick et al. 2008). Egyptian reptile species were classified according to their world distribution to reflect Egypt’s responsibility on their conservation; this was done following (Gilbert & Zalat 2008). The different classes are: widespread (the species exists in more than one continent and more than one ecological habitat); narrow (the species exists in six or more countries in a consistent band); restricted (the species exists in only 3-5 nearly connected countries); near-endemic (the species exists only in Egypt and a maximum of other two adjacent countries); and endemic (the species is only recorded from Egypt). Species world distribution was roughly determined using online databases (e.g. www.iucnredlist.org, www.catalogueoflife.org, and www.reptile- database.org) and also (Baha El Din 2006a). Distribution patterns within Egypt were determined following (Gilbert & Zalat 2008). This was done based on the number of eco-regions from which each species has been recorded. Egypt’s eco-regions were defined by (Boulos 1999-2006), who divided the country into eight eco-regions: Nile Valley, Oases, Mediterranean strip, Eastern Desert, Western Desert, Red Sea coast, Gebel Elba, and Sinai. Species were classified into three categories: widespread (recorded from four or more eco-regions), narrow (recorded from two or three eco-regions) and localized (recorded from only one eco-region). The number of eco-regions occupied by each species was determined using visual interpretations of species distributions across the different eco-regions. Neither the global nor national distribution classification is a fixed measure, but depends on visual interpretation and personal opinion. The analyses weighted species using their Red List conservation status in Egypt according to IUCN guideline and categories (IUCN Standards and Petitions Subcommittee 2010). Incomplete IUCN assessments were performed using only information derived from distribution records. As required by IUCN, these classifications involve the calculation of various measures: AOO (Area of Occupancy), EOO (Extent of Occurrence), and number of ‘locations’. AOO is defined as “the area within EOO, which is occupied by the species, excluding cases of vagrancy”. A grid size of 4.2 km 2 was used in the calculation of AOO (Annabelle Cuttleod, pers. comm.; following: Gilbert &
- 46 - Zalat 2008; Basuony et al. 2010). This was calculated by counting the number of grid cells occupied by the species and multiplying by the area of each grid cell. EOO is defined as “the area contained within the shortest continuous imaginary boundary which can be drawn to encompass all the known, inferred or projected sites of present occurrence of a taxon, excluding cases of vagrancy” (IUCN Standards and Petitions Subcommittee 2010). EOO is measured by calculating the area of the minimum convex polygon containing the records, i.e. the smallest polygon containing all the records that has no internal angle exceeding 180°: this was calculated using GME software (Geospatial Modelling Environment v0.6 – see: http://www.spatialecology.com/gme/). Any decline in AOO, EOO or number of locations (before and after 1950) were noted. The National Red Data List presented in this study should not be considered as the definitive view of the current status of Egyptian reptiles, but just gives a rough indication of their threat status. A detailed and accurate assessment is needed; a clear example is the status of the Egyptian tortoise, Testudo kleinmanni. It is classified globally as Critically Endangered with very limited current distribution in Egypt, but in this study, it is classified as Vulnerable based on information on its very limited distribution (see below). The global IUCN assessment of Egyptian species was obtained from the IUCN Red List website (IUCN Version 2012.1 - see: http://www.iucnredlist.org/).
Area prioritization for conservation Recently, several algorithms have been available to prioritize areas for conservation and conservation planning; these include Zonation (Moilanen et al. 2005; Moilanen 2007; Moilanen et al. 2012), Marxan (Game & Grantham 2008; Watts et al. 2009), ConsNet (Ciarleglio et al. 2008; Ciarleglio et al. 2009), MultCSync (Moffett et al. 2005), WorldMap (Williams 2001), and ResNet (Sarkar et al. 2002). Using the predictions from the species distribution models, Zonation v3.1 (Moilanen et al. 2012) was used in this study to create a nested spatial conservation prioritization to evaluate the effectiveness and performance of current Protected Area network in Egypt and to prioritize other sites for conservation (i.e. potential areas to expand the current PA network).
47 - Zonation is a framework for conservation prioritization and spatial conservation planning (Moilanen et al. 2012). It is based on hierarchically prioritizing the conservation value of the landscape based on the conservation value of sites (cells) (Moilanen 2007; Moilanen et al. 2012). The software starts with the full extent of the landscape, and then generates rankings of the cells by iteratively removing the least valuable cells from the edges of the landscape according to set rules (e.g. minimizing marginal loss of the conservation value and maintaining high habitat connectivity). The last to be removed are the most important areas (Moilanen et al. 2005; Moilanen et al. 2012). The order of the cell removal is recorded and can be used later to select any given top or bottom percentage of the landscape (e.g. highest or lowest 10%) (Moilanen 2007). In Zonation, there are four different cell-removal rule sets that specify how conservation value is aggregated across features and space, determining which cell leads to smallest marginal loss of biodiversity value: Basic core-area Zonation, Additive benefit function, Target- based planning, and Generalized benefit function (Moilanen et al. 2012). Two of these were used in this study (Basic core-area Zonation and Additive benefit function).
Basic core-area Zonation was used to identify important (or poor) locations where a single or a few species have important occurrences, while Additive benefit function was used to give more weight to locations with high species richness (Moilanen et al. 2012). The resulting maps of conservation importance do not agree completely, which means that there can be species- poor areas with some rare species. The manual suggests running Zonation with both removal options and comparing the results, since this may reveal some interesting information (Moilanen et al. 2012). In the calculations each species is given a weight, allowing some species to be more important to conserve than others. Unequal weights were assigned to all the reptile species using the Global IUCN assessment, species world distribution, distribution patterns within Egypt, and national IUCN species classification. For each element, a score was given (Table 8) to indicate relative importance, and then the sum of the scores gave the relative conservation weight of each species (Table 9).48 - Table 8: Different scores of different parameters used to calculate relative species weight.
Global IUCN status score National IUCN status score Not assessed 1 Least Concern 1 Least concern 1 Vulnerable - D2 2 Vulnerable 2 Endangered 3 Critically Endangered 3
Species world distribution score Distribution patterns within Egypt score Widespread 1 Widespread 1 Narrow 2 Narrow 2 Restricted 3 localized 3 Endemic/Near Endemic 4
It is undesirable to have a highly fragmented network of reserves, and therefore there is a balance to be struck between protecting all the best areas, and maintaining large reserves with good connectivity. This is accomplished in Zonation by the ‘aggregation rule’. I used Distribution Smoothing as the aggregation rule, which estimates the way each species uses the landscape and the spatial scale of dispersal, represented by an α-value for each species. The α-value indicates how species use the surrounding landscape and can be calculated based on the dispersal capability or the home range sizes of the species (Moilanen et al. 2012). I calculated it as the following:
2 [ ]
Dispersal distance needs to be in the same units as the predicted distribution maps (degrees in this study); so the α-value was adjusted by converting the measurement units from km to degrees using the resolution of the maps (2.5 arc-minutes, 0.0416°, about 4.6388 km).
2 ∗ [4.6388] [ ( )] ∗ [0.0416]
The dispersal ability of almost all terrestrial reptile species is very limited (Cadby et al. 2010; Edgar et al. 2010), which makes them more vulnerable to rapid environmental changes (Araujo & Pearson 2005). In this study, the dispersal distance of all reptiles was set to be equal to 1 km, entailing one α-value for all species (=223). Zonation was run using the maps of occurrence probabilities for all the species using both Basic core-area Zonation and Additive benefit function cell removal rules. The number of cells removed at each iteration was set to 10 (=
- 49 - ‘warp factor’). Both the current and mean probability distributions of different future GCMs were used. Zonation results were produced in the form of ASCII raster files, with values ranging from 0 to 1. Areas with Zonation rank values
0.7 were considered to be of high conservation importance. These important areas were then overlaid with the current Protected Area system in Egypt (Fig.
- to show if the network in Egypt is adequate to conserve the species in the face of climate change, and if there are areas outside the current PA boundaries that require special protection measures.
- 50 - Results
Model performance The performance of current distribution models, in terms of mean AUC values, was good. The mean AUC value of 10 repeat model runs for each species ranged from 0.78 to 0.99 with an overall mean of 0.93 ± 0.05. The lowest and highest mean AUC values were for Ptyodactylus siphonorhina (0.78 ± 0.09) and Mesalina pasteuri (0.99 ± 0.01), respectively (Fig. 11). Only one species (Ptyodactylus siphonorhina) had a mean AUC of less than 0.8; there were five species between 0.8 and 0.85, 14 species between 0.85 and 0.9, 19 species between 0.9 and 0.95 and 36 species with mean AUC values greater than 0.95 (Fig. 12). AUC variability among replicate runs was low, with standard deviations of less than 0.1 in 67 species, between 0.1 and 0.2 in six species, and between 0.2 and 0.3 in two species (Naja nubiae and Hemidactylus robustus) (Table 9). Mean AUC values for highly weighted species (weight value ≥ 12) range from 0.887 and 0.993, with a mean of 0.97 ± 0.03. As almost all species have a mean AUC value > 0.8, all models were accepted and processed for further analyses (see Table 9 for a list of mean AUC value, s.d., and the weighting value of each species). A highly significant strong negative correlation was found between mean AUC values and both of the predicted area occupied by the species (in terms of the number of currently predicted suitable pixels; n=75, r s = - 0.85, p<0.005 – Fig. 13) and species current extent of occurrence (EOO - calculated as the minimum convex polygon containing species distribution points; n=75, r s = - 0.77, p<0.005 – Fig. 14); and a significant weak negative correlation between mean AUC values and the number of species unique recorded points used to run the models (n=75, r s = - 0.433, p<0.005 – Fig. 15).
Most influential environmental variables Variables with highest mean permutation importance across all modelled species, and hence potentially the highest contribution to final models, were altitude, Bio4 (temperature seasonality) and Bio13 (precipitation of wettest
- 51 - month). Those with the lowest mean permutation importance, and thus not influencing the final models very much, were the difference between maximum and minimum NDVI, Bio15 (precipitation seasonality), habitat and Bio9 (mean temperature of driest quarter) (Fig. 16). The most influential environmental variable across species was altitude (highest for 34 species), followed by Bio4 (temperature seasonality – 10 species) and Bio13 (precipitation of wettest month – 9 species) (Fig. 17). Two environmental variables were never the most influential variable for any species: the difference between maximum and minimum NDVI and Bio9 (mean temperature of driest quarter).
Species richness Current species richness Using probability distributions, the areas with the highest current species richness are located at the greater Cairo area, the Suez Canal area extending from Suez to Port-Said, around Damietta and Alexandria and scattered small patches on Suez and Aqaba gulfs in Sinai and the Eastern Desert; other such areas are Wadi El-Natrun, Fayoum and scattered locations on the Mediterranean coast (Fig. 18). Using thresholded distributions, the areas with the highest species richness are the Suez Canal area, the greater Cairo southwards towards Fayoum and Beni Suef and eastwards towards Suez and Ismailia, the northern coast of the Nile Delta from Damietta to Rashid, Wadi El- Natrun, around Alexandria, coastal areas of the Suez and Aqaba Gulfs in Sinai and the Eastern Desert, and the north coast and coastal areas in North Sinai (Fig. 20).
Future species richness changes Using probability distributions, under the A2a scenario it is predicted that species richness will increase by 2020 in coastal areas between Safaga and El-Quseir, Bir Abraq area, patchy areas on the north coast from Alexandria to west of Mersa Matruh, both sides of the Suez Canal near El-Salam lake, east of Fayoum in the Nile valley, near Ras Mohamed in South Sinai and scattered areas from east and central Sinai northwards towards the Mediterranean
52 - coast. Species richness is predicted to decline around the Siwa oasis, inland wadis between El-Quseir and Mersa Alam, and small patches south of Abu Zneima in the Suez gulf (Figs. 18, 19). By 2050, species richness is predicted to increase on the northern coast from west Alexandria to Sidi Barrani across the northern edge of the Qattara Depression, in the Nile Valley (around El- Minia and Fayoum, and from Sohag to Edfu), Red Sea coastal areas from Mersa Alam to north of Hurghada, Western Desert oases (Kharga, Dakhla, and Farafra), South Sinai (around Ras Mohamed), and an area from central Sinai northwards to the Gebel El-Hallal area. It is predicted to decline in small areas around Gebel Elba, southwest of El-Quseir, Siwa oasis, Wadi El-Natrun, the greater Cairo, north of Suez and west of Ismailia (Figs. 18, 19). By 2080, species richness is predicted to increase more extensively along the north Mediterranean coast as far south as the northern part of the Qattara Depression, along the Red Sea coast from Mersa Alam to north of Hurghada, in the Nile Valley from Fayoum southwards, Western Desert oases, the Gebel El-Gallala area, on both sides of the northern parts of the Suez Canal, the coasts of South Sinai and a major part of central Sinai northwards to the Gebel El-Hallal area. It is predicted to decline in small areas of Gebel Elba, Wadi El- Natrun, the greater Cairo and scattered sites along the Suez Canal near Ismailia (Figs. 18, 19). Under the B2a scenario, the predicted patterns of change in species richness do not differ much from those of the A2a scenario (see Figs. 18, 19). By 2020, species richness is predicted to increase in Red Sea coastal areas near Berenice and El-Quseir and in small areas near El-Burullus Lake, with a greater area of increased species richness at the Mediterranean coast and in central to east Sinai. No increase is predicted at Bir Abraq and the Suez Canal area, and a greater decline in inland wadis near El-Quseir and at small coastal areas of the Suez and Aqaba gulfs near Zaafarana and north of Dahab. By 2050, there is predicted to be less increase in species richness, compared to the A2a scenario, along the Red Sea coast from south of El-Quseir to north of Hurghada, in South Sinai, and in the Western Desert oases; greater increase in central to east Sinai and in inland wadis west of El-Quseir; and no decline at all in Siwa and the Suez Canal area. By 2080, the overall pattern of species
53 - richness increase is the same as for the A2a scenario, but the magnitudes and areas of increased species richness are lower. Using thresholded distributions there are two assumptions to consider, unlimited dispersal or the complete absence of dispersal. For unlimited dispersal under the A2a scenario, it is predicted that species richness will increase by 2020 in the Bir Abraq area, inland areas of the north coast southwards to northern parts of the Qattara Depression, central Sinai northwards to the Gebel El-Hallal area, west of Cairo, coastal areas between Safaga to south of El-Quseir, and on the western side of the lower Nile Valley (the Tushka area). Declines are predicted in inland wadis from near Berenice northwards to Gebel El-Gallala, the area between Suez, Ismailia and Cairo, coastal areas on the Suez and Aqaba gulfs in Sinai and the Eastern Desert, the Gebel Elba area, Siwa oasis, and northwest of Wadi El-Natrun (Figs. 20, 21). By 2050, species richness is predicted to increase in the Qattara Depression and inland areas of the north coast, the Abraq area, the Gebel El- Gallala area, central Sinai northwards to the Gebel El-Hallal area, the northern part of El-Qaa plain on the eastern side of the Suez Gulf in Sinai, the lower Nile Valley westwards to the Tushka area, and Western Desert oases. Declines are predicted in the Suez Canal area, greater Cairo eastwards towards Suez and Ismailia, Wadi El-Natrun northwards, coastal areas of both the Suez and Aqaba gulfs, inland wadis near the Red Sea coast between Berenice and north of Hurghada, the Gebel Elba area and scattered areas near the North Sinai coast (Figs. 20, 21). By 2080, species richness is predicted to increase over extensive areas covering a large percentage of Egypt, including more than half of the Western Desert (the Qattara Depression, Western Desert oases and Tushka), Gebel El-Gallala, Bir Abraq, coastal areas north of El-Quseir and an area extending from central Sinai northwards to Gebel El-Hallal. Declines are predicted in the Wadi El-Natrun area, greater Cairo eastwards towards Suez and Ismailia, both sides of the Suez Canal, the Suez and Aqaba Gulfs, Gebel Elba, North Sinai north of Gebel El-Hallal and inland wadis from Mersa Alam to Hurghada (Figs. 20, 21). For unlimited dispersal under the B2a scenario, the overall pattern of predicted changes in species richness does not differ much from the A2a scenario (Figs. 20, 21). By 2020, there is predicted to be less increase in the
54 - Abraq area and greater declines in Wadi El-Natrun and the South Sinai coasts. By 2050, there will be less decline in Wadi El-Natrun and northern parts of the Suez Canal, and greater declines in the inland wadis of the Red Sea (west of Mersa Alam northwards) and on South Sinai coasts. By 2080, although the overall pattern of species richness increase does not differ from that of the A2a scenario, the magnitude of the increase is greater in central and southern parts of the Western Desert, Gebel El-Gallala, the northern part of the Nile Delta and coastal areas of the Red Sea; and less in coastal areas of North Sinai. Under the no-dispersal assumption and the A2a scenario, it is predicted that species richness will decline by 2020 in coastal areas of the Suez and Aqaba gulfs, the Suez Canal area, Wadi El-Natrun, the area between Cairo, Suez and Ismailia, Siwa oasis and the Qattara Depression, Gebel Elba, around Berenice, and inland wadis between Mersa Alam and north of Hurghada (Figs. 22, 23). By 2050, species richness is predicted to decline further in the area between Cairo, Ismailia and Suez, coastal areas of the Suez and Aqaba gulfs, the Nile Valley between Fayoum and Assiut, Red Sea inland wadis between Mersa Alam and north of Hurghada, scattered areas around Wadi El-Natrun, northern and central Sinai, and the Gebel Elba area (Figs. 22, 23). By 2080 the greatest decline in species richness is predicted to be from Suez southwards on both sides of the northern part of the Suez Gulf, Wadi El-Natrun northwards, and the area between Cairo, Ismailia and Suez; smaller declines are predicted in coastal areas around the Suez and Aqaba gulfs, the Suez Canal, Eastern Desert inland wadis, the Qattara Depression, Gebel Elba, Siwa oasis and Gebel El-Hallal northwards to the North Sinai coast (Fig. 22, 23). With no-dispersal and under the B2a scenario, the overall pattern of declines in future species richness does not differ much from the A2a scenario, but greater declines are predicted in Wadi El-Natrun, the area between Cairo, Suez and Ismailia, and on coastal areas on both sides of the Suez Gulf (Figs. 22, 23).
55 - Species Gains and Losses Under both dispersal assumptions and under the A2a scenario, the highest predicted species loss by 2020 is predicted to be in coastal areas on both sides of the Suez Gulf, coastal areas in the lower parts of the Aqaba Gulf, the area between the greater Cairo, Ismailia and Suez, Siwa oasis, the base of the Qattara Depression, inland wadis between Hurghada and Mersa Alam, coastal areas between north of Berenice and Abu Ramad, and Wadi El-Natrun northwards (Fig. 24). By 2050, the pattern of species loss is predicted to be the same as in 2020, with greater loss around the lower part of Suez Gulf, Siwa oasis, inland wadis between Hurghada and El-Quseir, northwestern parts of Sinai, around Farafra oasis, Wadi El-Natrun and in the Nile Valley from Fayoum to Minia; losses are predicted to decrease near Berenice (Fig. 24). By 2080, there is predicted to be greater species loss in the area between the greater Cairo, Ismailia and Suez, south of Suez on both sides of the Suez Gulf, and Wadi El-Natrun northwards; somewhat less in the area extending from central to north Sinai, northern and eastern parts of the Qattara Depression, the Berenice area and scattered locations on the north coast. Inland wadis between Hurghada and El-Quseir show smaller losses compared to 2050 (Fig. 24).
Under the B2a scenario, the overall pattern of species loss is much greater than predicted under the A2a scenario (Fig. 24). By 2020, there will be large losses on both sides of the Suez Gulf, Wadi El-Natrun northwards, the area between the greater Cairo, Ismailia, and Suez, inland wadis between Hurghada and El-Quseir, and coastal areas of the southern part of the Aqaba Gulf; these are somewhat less in the Berenice area, Siwa oasis, northern and eastern parts of the Qattara Depression and the Gebel Elba area. By 2050, the same pattern is predicted, with greater losses in Wadi El-Natrun northwards, the area between the greater Cairo, Ismailia and Suez, coastal areas on both sides of the Suez Gulf, Siwa oasis and the Qattara Depression; these are a bit lower in coastal areas of the Mediterranean from Sallum to Sinai, inland wadis near Gebel El-Hallal westwards, around the Farafra and Bahariya oases, coastal areas of the northern part of the Aqaba Gulf, around Fayoum and the Gebel Elba area. By 2080, the overall pattern of species loss does change from that of 2050, with smaller numbers of species being lost on56 - the Mediterranean coast, around Bahariya oasis and Fayoum, northern Sinai, the Qattara Depression and northern coastal areas of the Aqaba gulf. In terms of species loss relative to current (thresholded) species richness, under the A2a scenario the highest relative loss by 2020 is predicted to be in the Western Desert oases (Bahariya, Dakhla, and Kharga oases, and Siwa oasis south-eastwards), El-Gilf El-Kebir, both sides of the Nile Valley between Fayoum and Aswan, and inland wadis between Mersa Alam and Gebel El-Gallala (Fig. 25). This pattern is predicted not to change by 2050, but with relative greater declines in the Western Desert oases (except Bahariya) and relatively smaller declines in El-Gilf El-Kebir and inland and coastal areas of the northern half of the Eastern Desert, followed by Suez Gulf coasts in Sinai and the North Coast (Fig. 25). By 2080, Western Desert oases are predicted to undergo greater relative losses (except Bahariya where the areas of high relative loss shrink), followed by the Mediterranean coast (especially south-east of Sallum), the northern part of the Suez Gulf in Sinai, the area east of greater Cairo and northern and central parts of the Nile Delta. Areas of earlier high relative species loss in the northern part of the Eastern Desert are predicted to shrink in area by 2080 (Fig. 25).
Under B2a scenario, the overall pattern of relative species loss is similar to that of A2a scenario, with greater relative losses in inland wadis of the northern half of the Eastern Desert, around Western Desert oases, Wadi El-Natrun and the Mediterranean coast. By 2050, there is predicted to be greater relative loss in the area between Gebel Elba and Wadi El-Allaqi (Fig. 25). Under unlimited dispersal and the A2a scenario, the highest gains in species by 2020 are predicted to be on the Mediterranean coast west of Alexandria, central and south-eastern parts of the Qattara Depression, eastern and central Sinai northwards towards Gebel El-Hallal, Bir Abraq, and coastal areas north and south of El-Quseir (Fig. 26). By 2050, the predictions have the same overall pattern of species gain, with an expansion in area on the Mediterranean coast, the Qattara Depression and central to north Sinai; and with smaller gains at Bir Abraq (Fig. 26). By 2080, there is predicted to be continuing increases in gains on the Mediterranean coast, the Qattara57 - Depression, north of Siwa, scattered areas on both sides of the Nile Valley from Fayoum southwards, Gebel El-Maghrabi east of Edfu, Gebel El-Gallala and coastal areas between Hurghada and Mersa Alam; somewhat smaller in areas on both sides of the northern part of the Suez Canal and the Western Desert oases (Fig. 26).
Under the B2a scenario and unlimited dispersal, there is predicted to be an overall increase in gains across Egypt relative to the A2a scenario. By 2020, the highest increase in species gains is predicted to be on the Mediterranean coast, the Qattara Depression, areas around the Nile Valley from north of Fayoum to Edfu, the Western Desert oases, Bir Abraq, the coastal area from north of Hurghada to south of El-Quseir, Gebel El-Gallala, the area extending from eastern and central Sinai northwards to Gebel El- Hallal, south of Rafah, and areas on both sides of the northern part of the Suez Canal (Fig. 26). By 2050, a large proportion of the northern half of the Western Desert is predicted to gain many more species (including the Mediterranean coast and Siwa oasis, with the highest gains in the Qattara Depression). Areas with high predicted gains also include the Western Desert oases, the Tushka area northwards, areas on both sides of the Nile Valley from north of Fayoum southwards, Bir Abraq, the northern part of the Nile Delta, Gebel El-Gallala, coastal areas from north of Hurghada to Mersa Alam, an area extending from central to northwestern Sinai and south of Rafah (Fig. 26). By 2080, the pattern of areas of highest predicted gains is similar to that of 2050, with contractions in some areas including around Western Desert oases, Tushka, around the Nile Valley, the northern Nile Delta, Gebel El- Gallala, south of Rafah and the Red Sea coast from north of Hurghada to Mersa Alam (Fig. 26). Predicted gains in species relative to current (thresholded) species richness under the A2a scenario by 2020 are highest on both sides of the lower Nile Valley from Qena southwards, Tushka area, west of Fayoum towards the west and south of the Bahariya oasis, and north and south of Siwa; these are somewhat smaller west and north-west of Farafra, the Qattara Depression northwards towards inland areas of the Mediterranean coast, scattered areas in central Sinai, Kharga southwards and westwards, and a small area south of Damietta (Fig. 27). By 2050, the overall predicted pattern58 - is similar, with increases in the centre of the Qattara Depression northwards and an area extending from west of Fayoum towards west and south of Bahariya (Fig. 27). By 2080, there are predicted to be even greater increases in both area and magnitudes in the Qattara Depression northwards towards inland areas of the Mediterranean coast, north and south of Siwa, an area extending from west of Fayoum towards west and south of Bahariya oasis, around Dakhla and Kharga, and on both sides of the lower Nile Valley especially mountainous areas of the Eastern Desert (Fig. 27).
Under the B2a scenario, the overall pattern of relative gain shows much higher magnitudes compared to the A2a scenario. By 2020, the predicted pattern of relative gains resembles that of 2080 for the A2a scenario, and by 2050 and 2080, there is predicted to be large areas with relatively high gains, mainly in the Qattara Depression northwards, around Western Desert oases, west and north of Fayoum and around the lower part of the Nile Valley especially in mountainous areas of the Eastern Desert (Fig. 27).
Species Turnover Under unlimited dispersal and the A2a scenario, by 2020 the highest predicted turnover in species composition is predicted to be in the Western Desert (south-east of Bahariya westwards to the Libyan borders, around Dakhla, around Kharga down to the Sudanese borders, and north of El-Gilf El-Kebir) and around the Nile Valley from Assiut southwards; values are somewhat lower west and south of Fayoum, north and north-west of the Qattara Depression, and in the Bir Abraq area, as well as scattered inland wadis in the Eastern Desert (Fig. 28). By 2050, a similar pattern is predicted, with slight declines in turnover west of Farafra near the Libyan borders (Fig. 28). By 2080, the pattern again does not change much, with greater expansion in the areas of high turnover around the southern part of the Nile Valley and the Western Desert (south and west of Kharga and Dakhla); and slight increases in turnover in the greater Cairo area, Wadi El-Natrun westwards to Libya, the central Nile Delta and in central to northern Sinai (Fig. 28). Under the B2a scenario, the predicted pattern of species turnover resembles that of the A2a scenario, but with an overall increase in the areas of high turnover, and
- 59 - especially by 2050 continuing to 2080, almost all the southern half of the Western Desert is predicted to undergo high turnover in species composition (Fig. 28). When no-dispersal is allowed, under the A2a scenario the highest turnover in species composition by 2020 is predicted to be in the Bahariya oasis south-westwards towards the Libya border, around Kharga and Dakhla, El-Gilf El-Kebir, scattered areas on both sides of the lower Nile Valley from El- Minia southwards, south of Siwa and scattered mountainous locations in the middle of the Eastern Desert (Fig. 29). The predicted pattern does not change much by 2050 and 2080, with high declines predicted for Farafra westwards by 2050, and increases in El-Gilf El-Kebir by 2050 and 2080 (Fig. 29). Under the B2a scenario, the overall pattern of predicted species turnover does not differ much from the A2a scenario, but with greater increases in El-Gilf El- Kebir, south of Siwa, and areas around Bahariya, Kharga, and Farafra. By 2050 and 2080, there is predicted to be a moderate increase in species turnover in the central Nile Delta westwards to the Mediterranean coast and in scattered mountainous areas of the Eastern Desert (Fig. 29).
Range Changes
With unlimited dispersal, no species is predicted to become extinct in the
future under all or the average of the global circulation models. There are a
couple of species predicted to become extinct by losing their entire area of
suitable habitat in at least one of the future projections: Tarentola mindiae is
predicted to become extinct by 2080 under both emission scenarios of the
CSIRO and NIES99 models, and under the A2a scenario of the HadCM3
model; and Hemidactylus robustus is predicted to become extinct by 2080
under both emission scenarios of the CSIRO model, and under the A2a
scenario of the HadCM3 model (Table 10 - Fig. 30).
Using the average gain or loss of suitable habitat across the four
different global circulation models (Table 10), only one species is predicted to
be classified as Critically Endangered (i.e. predicted to lose more than 80% of
suitable habitat) by 2020: this species is Hemidactylus robustus under the A2a
scenario. By 2050, two species are predicted to be classified as Critically
- 60 - Endangered under both emission scenarios (Hemidactylus robustus and Tarentola mindiae). The number of such species is predicted to increase by 2080 to eight species: four under both emission scenarios (Eumeces schneiderii, Malpolon moilensis, Tarentola mindiae and Trapelus mutabilis), three under only the A2a scenario (Cerastes vipera, Eryx jaculus and Ptyodactylus guttatus) and one under only the B2a scenario (Hemidactylus robustus) (Tables 10, 12 - Fig. 30). Five species are predicted to be classified as Endangered (i.e. predicted to lose 50-80% of suitable habitat) by 2020: one under both emission scenarios (Tarentola mindiae), one under only the A2a scenario (Pristurus flavipunctatus) and three under only the B2a scenario (Hemidactylus robustus, Malpolon moilensis and Ptyodactylus guttatus). By 2050, six species are now predicted to be classified as Endangered under both emission scenarios (Eryx jaculus, Eumeces schneiderii, Malpolon moilensis, Pristurus flavipunctatus, Ptyodactylus guttatus and Trapelus mutabilis). By 2080, 16 species are predicted to be so classified: seven under both emission scenarios (Acanthodactylus longipes, Agama spinosa, Leptotyphlops macrorhynchus, Naja haje, Pristurus flavipunctatus, Ptyodactylus siphonorhina and Tarentola mauritanica), six under only the A2a scenario (Hemidactylus robustus, Laudakia stellio, Psammophis schokari, Sphenops sepsoides, Uromastyx aegyptia and Walterinnesia aegyptia) and three under only the B2a scenario (Cerastes vipera, Eryx jaculus and Ptyodactylus guttatus) (Tables 10, 12 - Fig. 30). Eight species are predicted to be classified as Vulnerable (i.e. predicted to lose 30-50% of suitable habitat) by 2020: two under both emission scenarios (Eryx jaculus and Trapelus mutabilis), three under only the A2a scenario (Malpolon moilensis, Ptyodactylus guttatus and Stenodactylus petrii) and three under only the B2a scenario (Pristurus flavipunctatus, Uromastyx aegyptia and Walterinnesia aegyptia). By 2050, eleven species are predicted to be classified as Vulnerable: seven under both emission scenarios (Acanthodactylus longipes, Leptotyphlops macrorhynchus, Naja haje, Ptyodactylus siphonorhina, Tarentola mauritanica, Uromastyx aegyptia and Walterinnesia aegyptia), one under only the A2a scenario (Cerastes vipera) and three under only the B2a scenario (Agama spinosa, Telescopus dhara
- 61 - and Tropiocolotes tripolitanus). By 2080, ten species are so classified: six under both emission scenarios (Acanthodactylus aegyptius, Malpolon monspessulanus, Scincus scincus, Telescopus dhara, Tropiocolotes tripolitanus and Uromastyx ocellata), one under only the A2a scenario (Stenodactylus petrii) and three under only the B2a scenario (Psammophis schokari, Uromastyx aegyptia and Walterinnesia aegyptia) (Tables 10, 12 - Fig. 30). Twenty-two species are classified as Least Concern (i.e. predicted to lose less than 30% of suitable habitat) by 2020: 13 under both emission scenarios, six under only the A2a scenario and three under only the B2a scenario. By 2050, 16 species are so classified: seven under both emission scenarios, seven under only the A2a scenario and two under only the B2a scenario. By 2080, only six are so classified: four under both emission scenarios and one each under only the A2a or B2a scenarios (Tables 10, 12 - Fig. 30). For those that are predicted to gain rather than to lose range under the unlimited dispersal assumption, three species are predicted to gain less than 30% of their current range under all combinations of time slices and emission scenarios. Some species are predicted the same, but not under all combinations of times and scenarios. By 2020, there are 28 of these species: 16 under both emission scenarios, four under only the A2a scenario and eight under only the B2a scenario. By 2050, there are 17 of these species: five under both emission scenarios, six under only the A2a scenario and six under only the B2a scenario. By 2080, there are ten such species: three under both emission scenarios, two under only the A2a scenario and five under only the B2a scenario (Tables 10, 12 - Fig. 31). Twelve species are predicted to gain 30-50% of their currently occupied area by 2020: four under both emission scenarios, six under only the A2a scenario and two under only the B2a scenario. By 2050, there are seven of these species: one under both emission scenarios, one under only the A2a scenario and five under only the B2a scenario. By 2080, there are eight such species: two under both emission scenarios, four under only the A2a scenario and two under only the B2a scenario (Tables 10, 12 - Fig. 31).
- 62 - Ten species are predicted to gain 50-80% of their currently occupied area by 2020: three under both emission scenarios, four under only the A2a scenario and three under only the B2a scenario. By 2050, there are 14 such species: nine at both emission scenarios, three under only the A2a scenario and two under only the B2a scenario. By 2080, there are nine such species: two under both emission scenarios, two under only the A2a scenario and five under only the B2a scenario (Tables 10, 12 - Fig. 31). Five species are predicted to gain 80-100% of their currently occupied area by 2020: two under both emission scenarios, two under only the A2a scenario and one under only the B2a scenario. By 2050, there are four such species: one under both emission scenarios, one under just the A2a scenario and two under just the B2a scenario. By 2080, there are five such species: three under just the A2a scenario and two under just the B2a scenario (Tables 10, 12 - Fig. 31). Five species are predicted to gain more than 100% of their currently occupied area under all combinations of time slices and emission scenarios. Some species are predicted to gain the same under at least one combination. By 2020, there are three under just the B2a scenario. By 2050, there are ten such species: eight under both emission scenarios and two under just the B2a scenario. By 2080, there are 18 such species: 13 under both emission scenarios, four under just the A2a scenario and one under just the B2a scenario (Tables 10, 12 - Fig. 31). The percentages of species falling under each category are shown in Fig. 33 a & b. The overall trend in predicted gain/loss (Fig. 34) indicates that under the assumption of unlimited dispersal there will be a continuous increasing gain in range for the average species with time, with little difference between emission scenarios; by 2080 under the A2a scenario there is slightly more gain and more variability than expected. The average range change across different taxonomic groups seems to be the highest for Varanidae (160% by 2020, and up to 600-800% increase by 2080); while for other groups, this ranges from 6% to 156% increase (Fig. 35). When assuming no dispersal at all, of course no gains in area are possible, merely losses. Again no species is predicted to become extinct
- 63 -
under all global circulation models. Two species are predicted to become
extinct in at least one of the projections: Tarentola mindiae is predicted to
become extinct by 2050 under the A2a scenario, and by 2080 at both emission
scenarios of the CSIRO model, and also under the A2a scenario of the
HadCM3 model; and Hemidactylus robustus is predicted to become extinct by
2080 under both scenarios of the CSIRO model, and under the A2a scenario
of the HadCM3 model (Tables 11, 13 - Fig. 32).
One species is predicted to be classified as Critically Endangered by
2020 (Hemidactylus robustus - under the A2a scenario). Three species are so
classified by 2050: two under both emission scenarios (Hemidactylus robustus
and Tarentola mindiae) and one under just the A2a scenario (Trapelus
mutabilis). There are ten such species by 2080: five under both emission
scenarios (Eumeces schneiderii, Malpolon moilensis, Ptyodactylus guttatus,
Tarentola mindiae and Trapelus mutabilis), four under just the A2a scenario
(Acanthodactylus longipes, Cerastes vipera, Eryx jaculus and Ptyodactylus
siphonorhina) and one under just the B2a scenario (Hemidactylus robustus)
(Tables 11, 13 - Fig. 32).
Seven species are predicted to be classified as Endangered by 2020: five under both emission scenarios (Malpolon moilensis, Pristurus flavipunctatus, Ptyodactylus guttatus, Tarentola mindiae and Uromastyx aegyptia) and two under just the B2a scenario (Hemidactylus robustus and Trapelus mutabilis). By 2050, there are 11 species so classified: nine under both emission scenarios (Acanthodactylus longipes, Eryx jaculus, Eumeces schneiderii, Malpolon moilensis, Pristurus flavipunctatus, Ptyodactylus guttatus, Ptyodactylus siphonorhina, Telescopus dhara and Uromastyx aegyptia) and one under each of A2a and B2a scenarios (Cerastes vipera and Trapelus mutabilis, respectively). In 2080, there are 22 such species: nine under both emission scenarios (Agama spinosa, Leptotyphlops macrorhynchus, Naja haje, Pristurus flavipunctatus, Sphenops sepsoides, Tarentola mauritanica, Telescopus dhara, Tropiocolotes steudneri and Uromastyx aegyptia), seven under just the A2a scenario (Acanthodactylus aegyptius, Hemidactylus robustus, Laudakia stellio, Psammophis schokari, Scincus scincus, Uromastyx ocellata and Walterinnesia aegyptia) and six under just the B2a scenario (Acanthodactylus longipes, Cerastes vipera, Eryx - 64 -
jaculus, Platyceps saharicus, Psammophis sibilans and Ptyodactylus
siphonorhina) (Tables 11, 13 - Fig. 32).
Thirteen species are classified as Vulnerable by 2020: eight under both
emission scenarios (Acanthodactylus longipes, Eryx jaculus, Leptotyphlops
macrorhynchus, Mesalina pasteuri, Platyceps saharicus, Psammophis
sibilans, Ptyodactylus siphonorhina and Telescopus dhara), one under just the
A2a scenario (Trapelus mutabilis) and four under just the B2a scenario
(Agama spinosa, Echis pyramidum, Tropiocolotes steudneri and Walterinnesia
aegyptia). By 2050, there are 17 such species: 13 under both emission
scenarios (Acanthodactylus aegyptius, Agama spinosa, Leptotyphlops
macrorhynchus, Naja haje, Platyceps saharicus, Psammophis schokari,
Psammophis sibilans, Spalerosophis diadema, Sphenops sepsoides,
Tarentola mauritanica, Tropiocolotes steudneri, Tropiocolotes tripolitanus and
Walterinnesia aegyptia), two under just the A2a scenario (Laudakia stellio and
Scincus scincus) and two under just the B2a scenario (Cerastes vipera and
Mesalina pasteuri). By 2080, 20 species are so classified: six under both
emission scenarios (Chalcides ocellatus, Echis pyramidum, Malpolon
monspessulanus, Platyceps florulentus, Spalerosophis diadema and
Tropiocolotes tripolitanus), eight under just the A2a scenario (Eirenis
coronella, Mesalina bahaeldini, Naja nubiae, Ophisops occidentalis, Platyceps
saharicus, Psammophis sibilans, Stenodactylus petrii and Tropiocolotes
bisharicus) and six under just the B2a scenario (Acanthodactylus aegyptius,
Laudakia stellio, Psammophis schokari, Scincus scincus, Uromastyx ocellata
and Walterinnesia aegyptia) (Tables 11, 13 - Fig. 32).
Thirty-five species are predicted to be classified as Least Concern under all possible combinations of time slices and emission scenarios. Other than these species, some species are so classified under at least one combination of settings. 25 species are predicted to be classified as Least Concern by 2020: 21 under both emission scenarios and four under just the A2a scenario. By 2050, 14 species are so classified: eleven under both emission scenarios, one under just the A2a scenario and two under just the B2a scenario. By 2080, there are seven such species: one under both emission scenarios and six under just the B2a scenario (Tables 11, 13 - Fig. 32). - 65 - The percentages of species predicted to be in each category under the no-dispersal assumption are shown in Fig. 33 c & d. The overall trend (Fig. 34) indicates that there will be a continuous increase in species loss through time, with few differences between emission scenarios except a greater-than- expected loss by 2020 under the B2a scenario and by 2080 under the A2a scenario. The average range decline across different taxonomic groups seems to be low for Varanidae (3-5% loss), followed by the tortoises (10-20%). The highest average loss by 2020 is predicted for the Agamidae (21-28%), followed by the Gekonidae (25%) and then the snakes (19-24%). By 2050, the highest average loss is predicted for the Geckonidae (31%), Agamidae (30%), snakes (29%) and Scincidae (26%); by 2080, for Agamidae (37-42%), Snakes (35-39%), Scincidae (32-39%) then by Gekonidae (36-38%) (Fig. 35).
Reptile species classifications According to the current IUCN Red List website (http://www.iucnredlist.org/), 19 Egyptian reptiles have been assessed globally: one is classified as Critically Endangered (Testudo kleinmanni), two as Vulnerable (Acanthodactylus pardalis and Trapelus savignii) and 16 as Least Concern. Fifty-six species have not been classified globally yet. Among the reptile species excluded from this study, two near-endemic species are in the IUCN global Red List: Philochortus zolii is Critically Endangered [B1 ab(iii)] and Telescopus hoogstraali is Endangered [B1ab(iii)] (Böhme & Baha El Din 2006b; Disi et al. 2006). According to Egypt’s national Red List assessment, three species are classified as Endangered (Leptotyphlops cairi, Chamaeleo africanus and Uromastyx ocellata), 24 as Vulnerable and 48 as Least Concern (for the global and national IUCN classification of the Egyptian reptiles, see Table 5). According to their world distribution, 6 species are classified as Endemic or Near-Endemic (Acanthodactylus aegyptius, Mesalina bahaeldini, Tarentola mindiae, Testudo kleinmanni, Trapelus savignii and Tropiocolotes bisharicus), eight as Restricted (Acanthodactylus pardalis, Naja nubiae, Ophisops occidentalis, Ptyodactylus siphonorhina, Sphenops sepsoides, Stenodactylus mauritanicus, Uromastyx ornata and Walterinnesia aegyptia),
- 66 - 52 species as Narrow and 9 as widespread (Table 5). Two endemics (Hemidactylus foudaii and Tropiocolotes bisharicus) and five near-endemics (Acanthodactylus pardalis, Philochortus zolii, Platyceps sinai, Telescopus hoogstraali and Tropiocolotes nubicus) were not included in this study due to their limited number of unique records. According to their distribution within Egypt, nine species are classified as Localized (Eirenis coronella, Mesalina bahaeldini, Mesalina pasteuri, Natrix tessellata, Ophisops occidentalis, Platyceps florulentus, Stenodactylus mauritanicus, Uromastyx ornata and Varanus niloticus), 31 as Narrow and 35 as Widespread (Table 5). Species with highest weighting scores were found to be Testudo kleinmanni, Trapelus savignii, Acanthodactylus pardalis, Mesalina bahaeldini, Ophisops occidentalis, Stenodactylus mauritanicus, Uromastyx ornata, Tarentola mindiae and Tropiocolotes bisharicus (Table 5).
Reptile records in Protected Areas Using database records, the highest number of recorded species per Protected Area was found at St Katherine, followed by Gebel Elba, Wadi El- Gemal and Lake Qarun (Table 14). No records were available from eight Protected Areas (Saluga & Ghazal, Ashtum El-Gamil, Sanur Cave, Nile Islands, Red Sea northern islands, El-Dababya, El-Sallum Gulf and Mt Kamel Meteor). Using the thresholded predicted distributions, the number of climatically suitable species per Protected Area was found to be highest at Lake Burullus, Nabq, Gebel Elba, Ashtum El-Gamil, Taba and Wadi El-Gemal (Table 14). There is a non-significant correlation between the number of recorded and climatically suitable species across Protected Areas (n=23, r s =0.49, p=0.12 one-tailed – Fig. 36). The highest difference between the number of predicted and recorded species was for Lake Burullus, Nabq, Siwa, Abu Galum, Ras Mohamed, Taba and Al-Ahrash. There is a significant positive correlation between the area of the Protected Areas and both the number of recorded species (n=23, r s =0.64, p=0.001 – Fig. 37) and the number of predicted climatically suitable species (thresholded) (n=23, r s =0.46, p=0.03 – Fig. 37).
- 67 - Under the assumption of unlimited dispersal and the A2a scenario, the highest predicted loss of species by 2020 is predicted to be at Ras Mohamed, followed by Lake Qarun, Wadi El-Assuti, Wadi Degla and the White Desert. By 2050, Wadi Degla is predicted to lose the most species, followed by St Katherine, Wadi Allaqi, Zaranik, Wadi El-Assuti, the White Desert and Wadi El- Rayan. By 2080, again Wadi Degla is predicted to lose the most species, followed by Ras Mohamed, Zaranik, Wadi Allaqi, the White Desert, St Katherine, Lake Qarun, El-Omayed and Wadi El-Gemal (Table 14). Under the B2a scenario, the highest predicted species loss by 2020 is predicted to be at Ras Mohamed, followed by Wadi Degla, the White Desert, Wadi El-Assuti, St Katherine and Lake Qarun. By 2050, the highest is Wadi Degla, Ras Mohamed and the White Desert; and by 2080, Wadi Degla, Wadi El-Assuti, the White Desert and Zaranik (Table 14). Under the A2a scenario, the highest predicted species gain by 2020 is for El-Dababya, Siwa and El-Omayed; by 2050, Siwa, Lake Qarun and Wadi El-Assuti; by 2080, Siwa and Taba (Table 14). Under the B2a scenario, the highest predicted species gain by 2020 is for Siwa and the White Desert; by 2050, Wadi El-Assuti, Siwa and the White Desert; by 2080, El-Omayed, Siwa and the White Desert (Table 14). Under the assumption of no-dispersal and the A2a scenario, the highest number of species lost by 2020 is predicted to be at Wadi Degla, Ras Mohamed, Wadi Allaqi and the White Desert; by 2050, Wadi Degla, Wadi Allaqi and Zaranik; and by 2080, Wadi Degla, Ras Mohamed, Zaranik and Nabq (Table 14). Under the B2a scenario, the highest predicted species loss by 2020 is at Ras Mohamed and Wadi Degla; by 2050, Ras Mohamed, Wadi Degla and the White Desert; and by 2080, Wadi Degla, Ras Mohamed, Wadi El-Assuti, White Desert and Zaranik (Table 14).
Area prioritization for conservation Using the assumption of ‘additive benefit function’, the areas with the highest current prioritization value were located on the Mediterranean coast from Rafah to Sallum, high-elevation areas of South Sinai, the Suez Canal area, greater Cairo north- and eastwards towards Suez, Ismailia and Sharqia and
- 68 - southwards towards Fayoum and El-Minia, the area between Cairo and Alexandria (including Wadi El-Natrun), coastal areas of both Suez and Aqaba Gulfs, the Red Sea coast, the Gebel Elba area, Siwa oasis, the Qattara Depression (especially the central and northern parts), scattered locations in the Nile Valley from Qena to Aswan, inland areas of North Sinai (Gebel El- Hallal), the Nile Delta, around Farafra and Kharga oases, inland wadis in the Eastern Desert, and moderate-elevation wadis in South and central Sinai (Fig. 38). The overall pattern of prioritization value does not seem to change very much in climate projections into the future. According to the A2a scenario, there will be a restricted decline confined to small area south of Siwa oasis (by
- and small wadi area west of Safaga (at all time slices) (Figs. 38, 39). By
2050, prioritization value is predicted to be increased in the Bir Abraq area,
Gebel El-Gallala southwards, western parts of central Sinai, east and south-
east of Suez, Siwa oasis, inland western parts of the Mediterranean coast, the
area between west of Bahariya oasis and Fayoum, inland wadis between
Mersa Alam and Safaga, and a small area on the Sudanese border (southwest
of Wadi El-Allaqi) (Figs. 38, 39). By 2080, there is predicted to be more areas
characterized by increased prioritization value, including west of Qarun Lake
and west of Bahariya oasis; followed by a large proportion of the northern part
of the Western Desert, inland wadis in the Eastern Desert (from Gebel El-
Gallala to Gebel Elba), both sides of the Nile Valley from Fayoum to Edfu,
around the Kharga, Dakhla and Farafra oases, and eastern and western parts
of central Sinai (Figs. 38, 39).
In the B2a scenario, by 2020 and 2050, there will be restricted declines in prioritization value confined to south of Siwa oasis, a small area north of El- Gilf El-Kebir and scattered locations in Eastern-Desert wadis (between Mersa Alam and Safaga) (Figs. 38, 39). By 2050, prioritization value is predicted to increase in the Abraq area southwards to Gebel Elba, Gebel El-Gallala, inland areas of the Mediterranean coast, Siwa oasis, west of Fayoum towards Bahariya and eastern and western parts of central Sinai (Figs. 38, 39). By 2080, there will be more such priority areas, including a major part of the northern half of the Western Desert, the Abraq area southwards to Gebel Elba, inland wadis from Gebel El-Gallala southwards to El-Quseir, the Nile Valley
69 - between Fayoum and Edfu, central Sinai, and around the Farafra, Dakhla and Kharga oases (Figs. 38, 39).
The mean prioritization value in all models was found to be higher in Protected Area than outside them, with overall a slight increase in prioritization value in the future. The difference in prioritization value between inside and outside Protected Areas seems to decline in the future, especially by 2050 and 2080 under the B2a scenario (Fig. 42). Using the ‘core-area function’ assumption, areas with the highest current prioritization value were located in high-elevation wadis in South Sinai, Gebel Elba, Siwa oasis, the northern part of the Qattara Depression, the Suez Canal area, coastal areas of the Aqaba Gulf, Red Sea coastal areas from south of Safaga to south of Halayeb, the Mediterranean coast from Sallum to Rafah and the Nile Valley from Qena to the Sudanese border. Slightly lower priority was given to non-coastal areas in North Sinai (Gebel El-Hallal); the greater Cairo area eastwards to Ismailia and Suez, westwards towards Wadi El-Natrun and southwards in the Nile Valley towards Fayoum and Qena; and coastal areas on both sides of the Suez Gulf (Fig. 40). The overall pattern of prioritization value does not seem to change very much in the future, but there are more changes than when additive benefit function was used. Under the A2a scenario, prioritization value is predicted to decline by 2020 in El-Gilf El-Kebir, around Farafra oasis and south of Siwa; while increase north of Siwa, south of the Qattara Depression, around Bahariya, south-east and south-west of Suez, east of El-Gilf El-Kebir (on the Sudanese border) and near Hurghada (Figs. 40, 41). By 2050, the pattern of prioritization value decline resembles that of 2020, but with greater decline around Farafra oasis, and increases from around Fayoum westwards to the southern part of the Qattara Depression, north of the Qattara Depression, north of Siwa, east of El-Gilf El-Kebir eastwards to the Nile Valley, the Red Sea coast between west of El-Quseir to Gebel El-Gallala, western parts of central Sinai and Bir Abraq southwards to small areas of Gebel Elba (Figs. 40, 41). By 2080, the overall pattern of prioritization value change does not change much from 2050: there are greater declines around Siwa, and smaller declines (and shrinking areas) around Farafra; but increased prioritization70 - value in both area and amount in some areas, especially west of Fayoum towards the Qattara Depression and east of El-Gilf El-Kebir (Figs. 40, 41). As under the B2a scenario, prioritization value is predicted to decline by 2020 south of Siwa, around Farafra, El-Gilf El-Kebir, east of Cairo and west of Hurghada; while increase north of Siwa, south of the Qattara Depression, east of Bahariya, around Fayoum, along the Red Sea coast from Gebel El-Gallala to south-west of Safaga, east of El-Gilf El-Kebir on the Sudanese border, south-east of Aswan and eastern and western parts of central Sinai (Figs. 40, 41). By 2050, prioritization value is predicted to decline in El-Gilf El-Kebir, south of Siwa, around Farafra and in between the greater Cairo and Suez; while increase between Fayoum and south of Fayoum westwards to the Libya border, the southern part of the Western Desert near Sudan, north of El-Gilf El-Kebir, Bir Abraq southwards to Gebel Elba, Eastern Desert inland wadis from Gebel El-Gallala to Safaga and scattered locations in central Sinai (Figs. 40, 41). By 2080, prioritization value is predicted to decline in El-Gilf El-Kebir, south of Siwa, around Farafra and east of Cairo; while increase in an area extending from Fayoum southwards to El-Minia and westwards to the Libyan border, Red Sea inland wadis from Gebel El-Gallala southwards to El-Quseir, Bir Abraq southwards to Gebel Elba, around the Nile valley from Edfu southwards and westwards (including Tushka), and scattered locations in central Sinai (Figs. 40, 41). Mean prioritization value in all cases were found to be higher inside Protected Area than outside, with overall a moderate increase predicted in the future (except by 2020 under the A2a scenario). The difference in prioritization value between inside and outside Protected Areas seems to be higher using the ‘core area’ rather than the ‘additive benefit’ function, with slightly smaller differences in the future (Figs. 42, 43).
71 - Table 9: Mean and standard deviation of AUC values for each species
Species Mean AUC AUC SD
Weighting score 1 Cyrtopodion scabrum 0.951 0.097 2 2 Hemidactylus flaviviridis 0.961 0.105 8 3 Hemidactylus robustus 0.899 0.289 8 4 Hemidactylus turcicus 0.955 0.021 1 5 Pristurus flavipunctatus 0.991 0.004 8 6 Ptyodactylus guttatus 0.953 0.026 4 7 Ptyodactylus hasselquistii 0.932 0.031 2 8 Ptyodactylus siphonorhina 0.781 0.09 3 9 Stenodactylus mauritanicus 0.989 0.012 18 10 Stenodactylus petrii 0.939 0.034 2 11 Stenodactylus sthenodactylus 0.85 0.041 2 12 Tarentola annularis 0.906 0.046 2 13 Tarentola mauritanica 0.978 0.014 2 14 Tarentola mindiae 0.97 0.019 16 15 Tropiocolotes bisharicus 0.986 0.017 16 16 Tropiocolotes nattereri 0.975 0.015 4 17 Tropiocolotes steudneri 0.879 0.037 2 18 Tropiocolotes tripolitanus 0.95 0.036 4 19 Agama spinosa 0.964 0.019 4 20 Laudakia stellio 0.983 0.008 4 21 Pseudotrapelus sinaitus 0.881 0.08 2 22 Trapelus mutabilis 0.947 0.026 2 23 Trapelus pallidus 0.943 0.036 2 24 Trapelus savignii 0.982 0.007 32 25 Uromastyx aegyptia 0.945 0.022 2 26 Uromastyx ocellata 0.923 0.077 12 27 Uromastyx ornata 0.99 0.005 18 28 Chamaeleo africanus 0.963 0.043 12 29 Chamaeleo chamaeleon 0.976 0.013 2 30 Acanthodactylus aegyptius 0.936 0.05 4 31 Acanthodactylus boskianus 0.889 0.02 2 32 Acanthodactylus longipes 0.962 0.022 4 33 Acanthodactylus pardalis 0.983 0.017 24 34 Acanthodactylus scutellatus 0.859 0.03 2 35 Mesalina bahaeldini 0.978 0.023 24 36 Mesalina guttulata 0.845 0.055 2 37 Mesalina olivieri 0.951 0.042 2
- 72 -
Species Mean AUC AUC SD
Weighting score 38 Mesalina pasteuri 0.993 0.007 12 39 Mesalina rubropunctata 0.839 0.071 2 40 Ophisops occidentalis 0.992 0.006 18 41 Varanus griseus 0.892 0.04 2 42 Varanus niloticus 0.985 0.021 12 43 Chalcides cf. humilis 0.87 0.155 4 44 Chalcides ocellatus 0.952 0.015 1 45 Eumeces schneiderii 0.961 0.043 4 46 Scincus scincus 0.87 0.08 2 47 Sphenops sepsoides 0.92 0.027 3 48 Trachylepis quinquetaeniata 0.956 0.021 2 49 Trachylepis vittata 0.988 0.012 8 50 Leptotyphlops cairi 0.948 0.058 12 51 Leptotyphlops macrorhynchus 0.926 0.113 8 52 Eryx colubrinus 0.91 0.113 8 53 Eryx jaculus 0.982 0.012 2 54 Eirenis coronella 0.985 0.018 12 55 Lytorhynchus diadema 0.894 0.047 2 56 Macroprotodon cucullatus 0.985 0.023 4 57 Malpolon moilensis 0.874 0.076 2 58 Malpolon monspessulanus 0.98 0.017 2 59 Natrix tessellata 0.978 0.012 6 60 Platyceps florulentus 0.957 0.027 6 61 Platyceps rogersi 0.943 0.071 2 62 Platyceps saharicus 0.882 0.097 4 63 Psammophis aegyptius 0.84 0.055 2 64 Psammophis schokari 0.934 0.03 2 65 Psammophis sibilans 0.979 0.009 4 66 Spalerosophis diadema 0.935 0.036 1 67 Telescopus dhara 0.936 0.074 4 68 Naja haje 0.961 0.026 4 69 Naja nubiae 0.897 0.214 12 70 Walterinnesia aegyptia 0.887 0.075 12 71 Cerastes cerastes 0.838 0.044 2 72 Cerastes vipera 0.922 0.038 2 73 Echis coloratus 0.878 0.11 2 74 Echis pyramidum 0.917 0.128 2 75 Testudo kleinmanni 0.969 0.026 48
- 73 -
Fig. 11: Box-and-whiskers plot for mean AUC values across 75 studied species. The horizontal dark line in the middle of the box indicates the median; the box indicating the 1 st and 3 rd quartiles; and the Whiskers indicate minimum and maximum mean AUC values.
Fig. 12: Frequency distribution of species mean AUC values showing the number of species at different ranges of mean AUC values.
Fig. 13: The correlation between species mean AUC value and its predicted area of suitable habitats (in terms of the number of currently predicted suitable pixels of the thresholded distribution) (n=75, r s =-0.85, p<0.005).
- 74 -
Fig. 14: The correlation between species mean AUC value and its current extent of occurrence (calculated as the minimum convex polygon containing species distribution points) in 1000 Km 2 (n=75, r s =-0.77, p<0.005).
Fig. 15: The correlation between species mean AUC value and the number of its unique recorded points used to run the models (n=75, r s =-0.433, p- value<0.005).
- 75 -
Fig. 16: Relative contribution of environmental variables to the final model, represented by the mean value of average permutation importance across species (± 95% confidence limit).
Fig. 17: The number of species at which each environmental variable was considered as the most influential environmental variable. Two variables are not shown (the difference between maximum and minimum NDVI and Bio9), as they have not been shown as the most influential environmental variable for any species.
- 76 - Current
Fig. 18: Mean predicted reptile species richness using the summation of species predicted probability distributions (current and future – assuming unlimited dispersal). Colour gradient ranked from grey (low species richness) to red (high species richness).y
A2a
2020 2050 2080
B2a
2020 2050 2080
77 - A2a
2020 2050 2080
B2a2020 2050 2080
Fig. 19: Future potential species richness change as a result of anthropogenic climate change (using species probability distributions – assuming unlimited dispersal); calculated as the difference between each of future species richness maps and current species richness (shown in Fig. 17). Colour gradient indicates how much future species richness change is: grey indicates no much change, dark green indicates high future species richness increase, and dark red indicates high future species richness decline.
- 78 - Current
Fig. 20: Mean predicted reptile species richness using the summation of species thresholded predicted distributions (current and future - assuming unlimited dispersal). Colour gradient ranked from green (low species richness) to red (high species richness).
A2a
2020 2050 2080
B2a
2020 2050 2080
79 - A2a
2020 2050 2080
B2a2020 2050 2080
Fig. 21: Future potential species richness change as a result of anthropogenic climate change (using predicted species thresholded distributions – assuming unlimited dispersal); calculated as the difference between each of future species richness maps and current species richness (shown in Fig. 19). Colour gradient indicates how much future species richness change is: grey indicates no much change, dark green indicates high future species richness increase, and dark red indicates high future species richness decline.
- 80 - Current
Fig. 22: Mean predicted reptile species richness using the summation of species thresholded predicted distributions (current and future - assuming no- dispersal). Colour gradient ranked from green (low species richness) to red (high species richness).
A2a
2020 2050 2080
B2a
2020 2050 2080
81 - A2a
2020 2050 2080
B2a2020 2050 2080
Fig. 23: Future potential species richness change (decline) as a result of anthropogenic climate change (using predicted species thresholded distributions – assuming no-dispersal); calculated as the difference between each of future species richness maps and current species richness (shown in Fig. 21). Colour gradient indicates how much future species richness decline is: grey indicates (no much change) to dark red (high future species richness decline).
82 - A2a
2020 2050 2080
B2a2020 2050 2080
Fig. 24: Potential future species loss as a result of anthropogenic climate change (assuming both dispersal assumptions: unlimited and no- dispersal).
For each species possible future projection, currently suitable pixels predicted to loss its suitability in the future are exported to separate files, then average loss across different global circulation models was calculated. The summation of mean future loss across species was then performed to show which area will potentially loss much species in the future. Colour gradient indicate how much species will be lost in the future: grey indicates no species loss, dark green indicates low species loss, and dark red indicates high species loss.
83 - A2a
2020 2050 2080
B2a2020 2050 2080
Fig. 25: Relative potential future species loss (assuming both dispersal assumptions: unlimited and no-dispersal); calculated as the percentage between the number of species predicted to be lost (Fig. 23) and current thresholded species richness (Fig. 19) for each pixel. Grey indicated low relative species loss and dark red indicated high relative species loss.
84 - A2a
2020 2050 2080
B2a2020 2050 2080
Fig. 26: Potential future species gain as a result of anthropogenic climate change (assuming unlimited dispersal).
For each species possible future projection, currently non-suitable pixels predicted to gain suitability in the future (assuming unlimited dispersal) are exported to separate files, then average gain across different global circulation models was calculated. The summation of mean future gain across species was then performed to show which area will potentially gain much species in the future. Colour gradient indicate how much species will be gained in the future: grey indicates no species gain, dark green indicates low species gain, and dark red indicates high species gain.
85 - A2a
2020 2050 2080
B2a2020 2050 2080
Fig. 27: Relative potential future species gain (unlimited dispersal assumption); calculated as the percentage between the number of gained species (unlimited dispersal - Fig. 25) and current thresholded species richness (Fig. 19) for each pixel. Grey colour indicates low relative species gain and dark red indicates high relative species gain.
86 - A2a
2020 2050 2080
B2a2020 2050 2080
Fig. 28: Future species turnover (a measure of dissimilarity between current and future species composition) assuming unlimited dispersal. Colour range from grey (low species turnover – small species composition change in the future) to dark red (high species turnover – high species composition change in the future).
- 87 -
A2a
2020 2050 2080
B2a
2020 2050 2080
Fig. 29: Future species turnover (a measure of dissimilarity between current and future species composition) assuming no-dispersal. Colour range from grey (low species turnover – small species composition change in the future) to dark red (high species turnover – high species composition change in the future).
- 88 - Table 10: Species classification according to future species range change (percentage of suitable habitats lost or gained - assuming unlimited dispersal).
Abbreviations used: Critically Endangered “CR”: loss>80%; Endangered “EN”: loss 50- 80%; Vulnerable “VU”: loss 30-50%; Least Concern “LC”: loss<30 %; Gain 1: gain <30%; Gain 2: gain 30-50%; Gain 3: gain 50-80%; Gain 4: gain 80-100%; Gain 5: gain
100%.
Species
A2 2020 A2 2050 A2 2080 B2 2020 B2 2050 B2 2080 1 Cyrtopodion scabrum Gain 5 Gain 5 Gain 5 Gain 5 Gain 5 Gain 5 2 Hemidactylus flaviviridis Gain 5 Gain 5 Gain 5 Gain 5 Gain 5 Gain 5 3 Hemidactylus robustus CR CR EN EN CR CR 4 Hemidactylus turcicus Gain 2 Gain 3 Gain 3 Gain 2 Gain 3 Gain 3 5 Pristurus flavipunctatus EN EN EN VU EN EN 6 Ptyodactylus guttatus VU EN CR EN EN EN 7 Ptyodactylus hasselquistii Gain 1 Gain 3 Gain 5 Gain 1 Gain 4 Gain 5 8 Ptyodactylus siphonorhina LC VU EN LC VU EN 9 Stenodactylus mauritanicus Gain 1 Gain 1 Gain 1 Gain 1 Gain 1 Gain 1 10 Stenodactylus petrii LC LC VU Gain 1 LC LC 11 Stenodactylus sthenodactylus Gain 4 Gain 5 Gain 5 Gain 4 Gain 5 Gain 5 12 Tarentola annularis Gain 4 Gain 5 Gain 5 Gain 5 Gain 5 Gain 5 13 Tarentola mauritanica Gain 1 VU EN LC VU EN 14 Tarentola mindiae EN CR CR EN CR CR 15 Tropiocolotes bisharicus LC Gain 1 LC Gain 1 LC LC 16 Tropiocolotes nattereri Gain 3 Gain 5 Gain 5 Gain 5 Gain 5 Gain 5 17 Tropiocolotes steudneri Gain 1 Gain 1 Gain 1 Gain 1 Gain 1 Gain 1 18 Tropiocolotes tripolitanus LC LC VU LC VU VU 19 Agama spinosa LC LC EN LC VU EN 20 Laudakia stellio Gain 1 LC EN Gain 3 Gain 1 LC 21 Pseudotrapelus sinaitus Gain 1 Gain 3 Gain 4 Gain 1 Gain 3 Gain 3 22 Trapelus mutabilis VU EN CR VU EN CR 23 Trapelus pallidus Gain 2 Gain 3 Gain 4 Gain 1 Gain 3 Gain 3 24 Trapelus savignii Gain 1 Gain 4 Gain 5 Gain 1 Gain 3 Gain 5 25 Uromastyx aegyptia LC VU EN VU VU VU 26 Uromastyx ocellata LC LC VU LC LC VU 27 Uromastyx ornata Gain 5 Gain 5 Gain 5 Gain 5 Gain 5 Gain 5 28 Chamaeleo africanus Gain 2 Gain 3 Gain 5 Gain 1 Gain 1 Gain 3 29 Chamaeleo chamaeleon Gain 4 Gain 5 Gain 5 Gain 4 Gain 5 Gain 5 30 Acanthodactylus aegyptius LC LC VU LC LC VU 31 Acanthodactylus boskianus Gain 1 Gain 1 Gain 2 Gain 1 Gain 1 Gain 1 32 Acanthodactylus longipes LC VU EN Gain 1 VU EN 33 Acanthodactylus pardalis Gain 2 Gain 3 Gain 1 Gain 3 Gain 3 Gain 2 34 Acanthodactylus scutellatus Gain 2 Gain 3 Gain 3 Gain 2 Gain 3 Gain 3 35 Mesalina bahaeldini Gain 2 Gain 1 Gain 1 Gain 1 Gain 2 Gain 1 36 Mesalina guttulata Gain 2 Gain 3 Gain 5 Gain 2 Gain 3 Gain 5
- 89 -
Species
A2 2020 A2 2050 A2 2080 B2 2020 B2 2050 B2 2080 37 Mesalina olivieri Gain 4 Gain 5 Gain 5 Gain 5 Gain 5 Gain 5 38 Mesalina pasteuri LC Gain 3 Gain 5 LC Gain 3 Gain 5 39 Mesalina rubropunctata Gain 3 Gain 5 Gain 5 Gain 3 Gain 5 Gain 5 40 Ophisops occidentalis Gain 1 LC LC Gain 1 Gain 1 LC 41 Varanus griseus Gain 1 Gain 3 Gain 4 Gain 1 Gain 3 Gain 3 42 Varanus niloticus Gain 5 Gain 5 Gain 5 Gain 5 Gain 5 Gain 5 43 Chalcides cf. humilis Gain 5 Gain 5 Gain 5 Gain 5 Gain 5 Gain 5 44 Chalcides ocellatus Gain 1 Gain 1 Gain 1 Gain 1 Gain 1 Gain 1 45 Eumeces schneiderii LC EN CR LC EN CR 46 Scincus scincus LC LC VU Gain 1 LC VU 47 Sphenops sepsoides Gain 1 Gain 1 EN Gain 1 Gain 1 LC 48 Trachylepis quinquetaeniata Gain 3 Gain 5 Gain 5 Gain 3 Gain 5 Gain 5 49 Trachylepis vittata Gain 3 Gain 5 Gain 5 Gain 3 Gain 5 Gain 5 50 Leptotyphlops cairi Gain 1 Gain 3 Gain 5 Gain 1 Gain 2 Gain 4 51 Leptotyphlops macrorhynchus LC VU EN LC VU EN 52 Eryx colubrinus Gain 1 Gain 1 Gain 3 Gain 1 Gain 1 Gain 2 53 Eryx jaculus VU EN CR VU EN EN 54 Eirenis coronella Gain 2 Gain 1 LC Gain 3 Gain 2 Gain 1 55 Lytorhynchus diadema Gain 1 LC LC Gain 1 Gain 1 LC 56 Macroprotodon cucullatus Gain 2 Gain 3 Gain 5 Gain 2 Gain 3 Gain 3 57 Malpolon moilensis VU EN CR EN EN CR 58 Malpolon monspessulanus Gain 1 LC VU Gain 1 LC VU 59 Natrix tessellata Gain 1 Gain 1 Gain 2 Gain 1 Gain 1 Gain 2 60 Platyceps florulentus Gain 1 Gain 1 Gain 2 Gain 1 Gain 2 Gain 1 61 Platyceps rogersi Gain 3 Gain 5 Gain 5 Gain 2 Gain 4 Gain 5 62 Platyceps saharicus LC Gain 1 Gain 2 LC Gain 1 Gain 1 63 Psammophis aegyptius Gain 2 Gain 1 Gain 1 Gain 1 Gain 2 Gain 1 64 Psammophis schokari Gain 1 LC EN Gain 1 Gain 1 VU 65 Psammophis sibilans LC LC Gain 1 LC LC LC 66 Spalerosophis diadema Gain 1 Gain 2 Gain 2 Gain 1 Gain 2 Gain 2 67 Telescopus dhara LC LC VU LC VU VU 68 Naja haje LC VU EN LC VU EN 69 Naja nubiae Gain 3 Gain 5 Gain 3 Gain 4 Gain 3 Gain 5 70 Walterinnesia aegyptia LC VU EN VU VU VU 71 Cerastes cerastes Gain 3 Gain 4 Gain 5 Gain 2 Gain 4 Gain 4 72 Cerastes vipera Gain 1 VU CR LC LC EN 73 Echis coloratus Gain 1 Gain 1 Gain 1 LC LC Gain 1 74 Echis pyramidum LC LC LC LC LC LC 75 Testudo kleinmanni Gain 1 Gain 2 Gain 2 Gain 1 Gain 1 Gain 1
- 90 - Table: 11: Species classification according to future species range change (percentage of suitable habitats lost - assuming no-dispersal).
Abbreviations used: Critically Endangered “CR”: loss>80%; Endangered “EN”: loss 50-80%; Vulnerable “VU”: loss 30-50%; Least Concern “LC”: loss<30 %.
Species
A2 2020 A2 2050 A2 2080 B2 2020 B2 2050 B2 2080 1 Cyrtopodion scabrum LC LC LC LC LC LC 2 Hemidactylus flaviviridis LC LC LC LC LC LC 3 Hemidactylus robustus CR CR EN EN CR CR 4 Hemidactylus turcicus LC LC LC LC LC LC 5 Pristurus flavipunctatus EN EN EN EN EN EN 6 Ptyodactylus guttatus EN EN CR EN EN CR 7 Ptyodactylus hasselquistii LC LC LC LC LC LC 8 Ptyodactylus siphonorhina VU EN CR VU EN EN 9 Stenodactylus mauritanicus LC LC LC LC LC LC 10 Stenodactylus petrii LC LC VU LC LC LC 11 Stenodactylus sthenodactylus LC LC LC LC LC LC 12 Tarentola annularis LC LC LC LC LC LC 13 Tarentola mauritanica LC VU EN LC VU EN 14 Tarentola mindiae EN CR CR EN CR CR 15 Tropiocolotes bisharicus LC LC VU LC LC LC 16 Tropiocolotes nattereri LC LC LC LC LC LC 17 Tropiocolotes steudneri LC VU EN VU VU EN 18 Tropiocolotes tripolitanus LC VU VU LC VU VU 19 Agama spinosa LC VU EN VU VU EN 20 Laudakia stellio LC VU EN LC LC VU 21 Pseudotrapelus sinaitus LC LC LC LC LC LC 22 Trapelus mutabilis VU CR CR EN EN CR 23 Trapelus pallidus LC LC LC LC LC LC 24 Trapelus savignii LC LC LC LC LC LC 25 Uromastyx aegyptia EN EN EN EN EN EN 26 Uromastyx ocellata LC LC EN LC LC VU 27 Uromastyx ornata LC LC LC LC LC LC 28 Chamaeleo africanus LC LC LC LC LC LC 29 Chamaeleo chamaeleon LC LC LC LC LC LC 30 Acanthodactylus aegyptius LC VU EN LC VU VU 31 Acanthodactylus boskianus LC LC LC LC LC LC 32 Acanthodactylus longipes VU EN CR VU EN EN 33 Acanthodactylus pardalis LC LC LC LC LC LC 34 Acanthodactylus scutellatus LC LC LC LC LC LC 35 Mesalina bahaeldini LC LC VU LC LC LC 36 Mesalina guttulata LC LC LC LC LC LC 37 Mesalina olivieri LC LC LC LC LC LC
- 91 -
Species
A2 2020 A2 2050 A2 2080 B2 2020 B2 2050 B2 2080 38 Mesalina pasteuri VU LC LC VU VU LC 39 Mesalina rubropunctata LC LC LC LC LC LC 40 Ophisops occidentalis LC LC VU LC LC LC 41 Varanus griseus LC LC LC LC LC LC 42 Varanus niloticus LC LC LC LC LC LC 43 Chalcides cf. humilis LC LC LC LC LC LC 44 Chalcides ocellatus LC LC VU LC LC VU 45 Eumeces schneiderii LC EN CR LC EN CR 46 Scincus scincus LC VU EN LC LC VU 47 Sphenops sepsoides LC VU EN LC VU EN 48 Trachylepis quinquetaeniata LC LC LC LC LC LC 49 Trachylepis vittata LC LC LC LC LC LC 50 Leptotyphlops cairi LC LC LC LC LC LC 51 Leptotyphlops macrorhynchus VU VU EN VU VU EN 52 Eryx colubrinus LC LC LC LC LC LC 53 Eryx jaculus VU EN CR VU EN EN 54 Eirenis coronella LC LC VU LC LC LC 55 Lytorhynchus diadema LC LC LC LC LC LC 56 Macroprotodon cucullatus LC LC LC LC LC LC 57 Malpolon moilensis EN EN CR EN EN CR 58 Malpolon monspessulanus LC LC VU LC LC VU 59 Natrix tessellata LC LC LC LC LC LC 60 Platyceps florulentus LC LC VU LC LC VU 61 Platyceps rogersi LC LC LC LC LC LC 62 Platyceps saharicus VU VU VU VU VU EN 63 Psammophis aegyptius LC LC LC LC LC LC 64 Psammophis schokari LC VU EN LC VU VU 65 Psammophis sibilans VU VU VU VU VU EN 66 Spalerosophis diadema LC VU VU LC VU VU 67 Telescopus dhara VU EN EN VU EN EN 68 Naja haje LC VU EN LC VU EN 69 Naja nubiae LC LC VU LC LC LC 70 Walterinnesia aegyptia LC VU EN VU VU VU 71 Cerastes cerastes LC LC LC LC LC LC 72 Cerastes vipera LC EN CR LC VU EN 73 Echis coloratus LC LC LC LC LC LC 74 Echis pyramidum LC LC VU VU LC VU 75 Testudo kleinmanni LC LC LC LC LC LC
- 92 - Table 12: Number of species at each species range change category (assuming unlimited dispersal)
Category
CCCma CSIRO HadCM3 NIES99 Overall mean A2a B2a A2a B2a A2a B2a A2a B2a A2a B2a 2020 2050 2080 2020 2050 2080 2020 2050 2080 2020 2050 2080 2020 2050 2080 2020 2050 2080 2020 2050 2080 2020 2050 2080 2020 2050 2080 2020 2050 2080 Extinct 0 0 0 0 0 0 0 0 2 0 0 2 0 0 2 0 0 0 0 0 1 0 0 1 0 0 0 0 0 0 Critically Endangered 0 1 6 1 1 4 1 4 6 2 3 5 2 2 9 1 2 4 2 8 19 3 7 11 1 2 7 0 2 5 Endangered 2 7 8 4 4 8 1 5 9 2 5 7 2 7 7 1 7 8 4 8 8 5 10 10 2 6 13 4 6 10 Vulnerable 5 6 11 8 9 9 5 11 11 9 8 9 5 2 10 8 5 8 9 4 2 9 5 6 4 8 7 5 10 9 Least Concern 26 17 4 29 20 16 17 13 3 14 14 9 16 15 4 17 16 10 12 12 6 8 6 4 19 14 5 16 10 8 Gain 1 21 19 11 19 19 12 23 16 7 21 14 8 26 10 9 25 14 11 26 8 4 12 10 9 23 14 8 27 14 11 Gain 2 11 4 9 5 8 7 11 7 8 13 5 6 9 6 5 9 8 5 7 4 1 10 5 5 10 2 6 6 6 4 Gain 3 7 7 6 3 3 3 5 6 4 6 10 3 7 15 5 6 7 7 3 8 6 8 8 3 7 12 4 6 11 7 Gain 4 1 3 6 2 4 2 4 2 3 3 2 6 3 4 2 2 1 5 2 2 4 3 4 5 4 2 3 3 3 2 Gain 5 2 11 14 4 7 14 8 11 22 5 14 20 5 14 22 6 15 17 10 21 24 17 20 21 5 15 22 8 13 19
Table 13: Number of species at each species range change category (assuming no-dispersal)
Category
CCCma CSIRO HadCM3 NIES99 Overall mean A2a B2a A2a B2a A2a B2a A2a B2a A2a B2a 2020 2050 2080 2020 2050 2080 2020 2050 2080 2020 2050 2080 2020 2050 2080 2020 2050 2080 2020 2050 2080 2020 2050 2080 2020 2050 2080 2020 2050 2080 Extinct 0 0 0 0 0 0 0 1 2 0 0 2 0 0 2 0 0 0 0 0 1 0 0 1 0 0 0 0 0 0 Critically Endangered 0 2 6 2 1 4 1 4 8 2 3 5 2 2 9 1 2 4 2 9 20 4 9 12 1 3 9 0 2 6 Endangered 4 10 14 7 6 12 4 10 15 5 8 12 4 10 15 5 10 10 8 13 11 8 14 14 5 10 16 7 10 15 Vulnerable 11 18 12 14 14 17 9 15 10 14 15 14 8 6 10 10 10 16 10 11 7 10 9 10 9 15 14 12 15 12 Least Concern 60 45 43 52 54 42 61 45 40 54 49 42 61 57 39 59 53 45 55 42 36 53 43 38 60 47 36 56 48 42
- 93 -
(a) Average
(b) CCCma (c) CSIRO
(d) HadCM3 (e) NIES99
Fig. 30: Number of species at each future species range change classification across global circulation models and the mean of different global circulation models (loss – unlimited dispersal).
Abbreviations used: EX: Extinct; CR: Critically Endangered; EN: Endangered; VU: Vulnerable; and LC: Least Concern.
- 94 -
(a) Average
(b) CCCma (c) CSIRO
(d) HadCM3 (e) NIES99
Fig. 31: Number of species at each future species range change classification across global circulation models and the mean of different global circulation models (gain – unlimited dispersal).
- 95 -
(a) Average
(b) CCCma (c) CSIRO
(d) HadCM3 (e) NIES99
Fig. 32: Number of species at each future species range change classification across global circulation models and the mean of different global circulation models (loss – no-dispersal).
Abbreviations used: EX: Extinct; CR: Critically Endangered; EN: Endangered; VU: Vulnerable; and LC: Least Concern.
- 96 -
(a) A2a (unlimited dispersal) (b) B2a (unlimited dispersal)
(c) A2a (no-dispersal) (d) B2a (no-dispersal)
Fig. 33: Percentage of each future specie range change classification assuming unlimited dispersal (a and b) and no-dispersal (c and d).
- 97 -
(unlimited dispersal)
(no-dispersal)
Fig. 34: The overall pattern of future mean species range change (mean percentage of gain or loss) under unlimited and no-dispersal assumptions.
- 98 -
(unlimited dispersal)
(no-dispersal)
Fig. 35: Average future range change across different taxonomic groups assuming either unlimited dispersal or no-dispersal assumptions.
The taxa contain variable numbers of species; Snakes (25 species), Gekonidae (18), Lacertidae (11), Agamidae (9), Scincidae (7), Chamaeleonidae (2), Varanidae (2) and Turtles (1).
- 99 -
Fig. 36: The correlation between number of recorded and predicted species per Protected Area (n=23, r s = 0.49, p=0.12). Seven Protected Areas were not involved in the analysis as they do not have any recorded or predicted reptile species.
Fig. 37: The correlation between the area of the Protected Area (in 100 km 2 ) and either of the number of recorded or predicted species per Protected Area. In both cases, seven Protected Areas were not involved in the analysis as they do not have any recorded or predicted reptile species (For recorded species, n=23, r s =0.638, P<0.005 – For predicted species: n=23, r s =0.46, P<0.05).
- 100 - Table 14: Number of reptile species currently recorded or predicted and the number of predicted future species loss (UD and ND) or gain (UD) in each Protected Areas.
For the name and location of the Protected Areas: see Fig. 5. Only Protected Areas with current or future species existence are listed in the table.
Protected Areas
1 2 3 4 5 7 9 10 11 12 13 14 16 17 18 19 21 22 23 24 26 27 29
Recorded species 8 13 9 15 27 28 22 15 15 1 2 2 4 7 16 5 15 2 9 24 11
1
Predicted species 42 40 39 42 47 43 47 42 32 23
49 42 47 51 23 43 22 45 11 18 4
(UD)
species loss
A2a-2020
6 2 1 2 4 4 5 3 3 5
3 1 4 2 5 2 5 1 2 1
A2a-2050 4 6 3 1 3 6 3 5 6 5
4 4 4 2 7 3 5 1 2 2 1 A2a-2080 12 11 5 7 6 7 7 5 8 6
1 6 5 5 12 4 8 7 2 4 2 B2a-2020 7 4 2 2 3 5 5 3 2 6
4 1 3 2 6 2 6 3 2 4 1 B2a-2050 8 4 3 1 3 3 4 5 4 5
4 2 3 3 9 3 8 2 2 4 1 B2a-2080 9 8 3 2 5 5 6 4 4 9
6 3 4 3 12 3 8 4 2 3 2
(UD)
species gained
A2a-2020
2
3
1 2
2
1
1
3 2 2 1 4
A2a-2050 3 2 5 2 1 1 6 2 1 6
2 1 3 4
8 5 3 3 5 2 A2a-2080 4 5 6 7 4 5 7 5 3 1
3 2 8 4 1 9 7 3 3 5 4 B2a-2020 3 1 1 3
2 3 1 1 3
1 1 2 1
4 4 1 2 3 1 B2a-2050 4 1 3 3
4 5 3
7
1
4 3 1 6 6 4 2 5 3 B2a-2080 4 4 4 8 3 5 6 4 2 5
2 1 6 4 2 7 7 4 3 6 3
(ND)
species loss
A2a-2020
5 2 1 2 4 4 4 2 5 4 4 1 4 2 6 2 5 1 4 2 1 A2a-2050 5 7 3 1 4 5 4 5 7 5 5 5 4 2 8 3 5 1 3 3 2 A2a-2080 12 11 5 7 8 7 8 5 9 6 10 6 6 5 12 4 8 7 3 4 1 B2a-2020 8 3 2 2 3 6 5 3 4 6 4 1 4 2 7 2 6 3 4 3 2 B2a-2050 10 4 3 1 4 3 5 5 6 5 4 3 4 3 9 3 7 2 4 4 1 B2a-2080 10 8 3 2 5 6 7 4 7 9 7 5 5 3 12 3 8 5 3 3 2
- 101 - Current
Fig. 38: Current and future conservation prioritization ranked value (using Zonation algorithm - Additive benefit function). Colours range from grey (low conservation value) to dark red (high conservation value).
A2a
2020 2050 2080
B2a
2020 2050 2080
102 - A2a
2020 2050 2080
B2a2020 2050 2080
Fig. 39: Future change in conservation prioritization value (using Zonation algorithm - Additive benefit function); calculated as the difference between future and current conservation prioritization value (Fig. 36). Grey colour indicates no change in prioritization value; dark red indicates high future decline, and dark green indicates high future increase.
- 103 - Current
Fig. 40: Current and future conservation prioritization ranked value (using Zonation algorithm – Core-Area function). Colours range from grey (low conservation value) to dark red (high conservation value).
A2a
2020 2050 2080
B2a
2020 2050 2080
104 - A2a
2020 2050 2080
B2a2020 2050 2080
Fig. 41: Future change in conservation prioritization value (using Zonation algorithm – Core-Area function); calculated as the difference between future and current conservation prioritization value (Fig. 38). Grey colour indicates no change in prioritization value; dark red indicates high future decline, and dark green indicates high future increase.
- 105 -
Fig. 42: Mean prioritization value (± 95% confidence limits - using Additive benefit function) across Protected Areas (PAs – grey coloured) and non-Protected areas (non-PAs – white coloured) at current and future.
Fig. 43: Mean prioritization value (± 95% confidence limits - using Core-Area function) across Protected Areas (PAs – grey coloured) and non- Protected areas (non-PAs – white coloured) at current and future.
- 106 - Discussion
Model performance From the AUC values, the models of all species discriminated much better than random; with all species having a mean AUC value greater than 0.7, this indicated at least an overall moderate discrimination ability. About 73% of the species had a mean AUC value greater than 0.9, indicating high discrimination ability (Franklin 2009). The negative correlations found between AUC and the area occupied, and with the extent of occurrence, concur with findings of other studies (e.g. Brotons et al. 2004; Elith et al. 2006; Hernandez et al. 2006). Species ecological characteristics have been shown to affect model accuracy, with more localized or rarer species being easier to model with higher accuracy than widespread species, regardless of sample size. This is probably because rare species are usually habitat specialists, show low environmental tolerance, and are environmentally or geographically restricted compared to widespread species (Stockwell & Peterson 2002; Brotons et al. 2004; Elith et al. 2006; Hernandez et al. 2006; Jiménez-Valverde et al. 2008; Franklin et al. 2009; Newbold et al. 2009b). Widespread species are more likely to be generalists occupying a wide range of habitats and climates, making it difficult to distinguish between suitable and unsuitable habitats (Franklin et al. 2009). This result may require further scrutiny (Elith et al. 2006), as it is very easy to get high AUC scores when modelling species distributions with low relative occurrence area (the proportion between the extent of species records and the extent of the study area – i.e. high extrapolation) (Jiménez-Valverde et al. 2008; Lobo et al. 2008). The smaller is the relative occurrence area (localized, rare, or endemic species), the greater is the number of available absences outside the limits of species records and better the model describes the data; this may be an inevitable result for species with small relative occurrence areas (Jiménez-Valverde et al. 2008; Lobo et al. 2008). Accordingly, some have advised against using AUC to compare model performances of species having different relative occurrence areas (Lobo et al. 2008). Given that most of the Egyptian herpetofauna (about 60%) are narrowly distributed, occupying less than 10% of Egypt’s area (Baha El Din 2006a), this may be the reason for
- 107 - having high mean AUC scores in this study. This is one of the main drawbacks of using AUC for testing model accuracy (Lobo et al. 2008). There is no evidence of a strong correlation between model accuracy and the number of records used to run the models (see: Elith et al. 2006; Newbold et al. 2009b). The weak negative correlation found in this study does not concur with the findings of other studies (e.g. Stockwell & Peterson 2002; Kadmon et al. 2003; Hernandez et al. 2006), which found that species with larger sample sizes seemed to have higher model accuracy (but see: de Pous et al. 2011). A possible reason for my finding is that the majority of Egyptian reptiles have localized distributions with few records compared to the large study area, resulting in small relative occurrence areas and consequently high model performance.
Variables contributing to the models Choosing the most appropriate variables that limit species distributions is a challenge in many species distribution modelling studies. They should be selected on the basis that they are ecologically meaningful and have high explanatory power (Beaumont et al. 2008). Environmental variables are substitutes, in general, for those variables that affects species distribution directly through physiological mechanisms, so poor selection of the variables used in the model (or the unavailability of data for variables thought to have direct effects on species distributions) may affect the association between the species and the climate (Araújo & Peterson 2012). In this study, I used available environmental layers thought to have an ecological meaning. Altitude was found to be the most effective variable for many species. The use of the two NDVI variables in the modelling of Egyptian reptiles proved to be not very useful, and probably future studies done on the same species and scale should not use them. Maximum NDVI was the most important variable only for Echis coloratus (although this information should be interpreted with caution), with an average permutation importance across species of 5.2 ± 5.9. The variable recording the difference between maximum and minimum NDVI was not the most important variable for any species, with an average permutation importance across species of 2.6 ± 4.1. NDVI has
108 - been used in many species distribution modelling studies done on a variety of species groups; including mammals (Torres et al. 2010; Hu & Jiang 2011; Soultan 2011), reptiles (Zabalaga 2009; Costa et al. 2010; Kgosiesele 2010; Taheri 2010; Carvalho et al. 2011; de Pous et al. 2011; Huang et al. 2011), amphibian (Tarkhnishvili et al. 2009; Beukema et al. 2010), birds (Niamir 2009), and even fungi (Flory et al. 2012). Some studies show a high importance of NDVI in species distribution modelling applications (e.g. Egbert et al. 2002; Anderson et al. 2006; Kgosiesele 2010; Taheri 2010), while others show only minor contributions (e.g. Torres et al. 2010; Soultan 2011). In Soultan’s study on the effect of climate change on Egyptian antelopes, NDVI had only a minor contribution to the models (Soultan 2011). As most of Egypt area is almost bare, except the Nile Valley and the Nile Delta, a large proportion of Egypt has very small NDVI values, making their use in modelling Egyptian fauna questionable. The habitat map produced by the BioMAP project seems to have made only a low contribution to the reptile models (with an average permutation importance across species of 3.9 ± 5.1), the most important variable for just one species (Leptotyphlops macrorhynchus). This may be because of the series of conversions and rescaling processes that converted it from vector to a relatively coarse raster format, or because many of the habitat categories are correlated with other variables, such as altitude. Neither slope nor aspect were used to run the models, although they are thought to affect the reptile distributions. They have been used in two other species distribution modelling studies done on Egyptian antelopes and a relatively high contributions to the final models were found (El Alqamy et al. 2010; Soultan 2011). Having an average indication of either slope or aspect across Egypt at the coarse resolution used here (~ 5 km) seems not to be that helpful. Giving just one value for either of them on a grid of 25-km 2 squares does not provide accurate information (especially with the moderate degree of uncertainty associated with some species records). Involving them in further studies at smaller scales may be more useful; these would need high- resolution variables and records with only minor levels of positional uncertainty.
109 - Species richness and turnover The pattern of species richness of the Egyptian herpetofauna from this study is consistent with the findings of (Baha El Din 2006a - see Fig. 44). The main pattern of herpetofauna hotspots in Egypt was found to be around the greater Cairo (including Wadi El-Natrun and Fayoum area), North Sinai, Gebel Elba area, and margins of Nile Delta eastwards to the Suez Canal area. Despite the high degree of concordance, this study shows fewer numbers of species in the high mountains of South Sinai, the Gebel Elba area and the western parts of the Mediterranean coast.
Fig. 44: The number of recorded amphibian and non-marine reptile species per a grid of half degree, comparing the results of (Baha El Din 2006a) (left) to the results of this study (right).
The current predicted pattern of species richness (using either probability or thresholded distributions) is consistent with the recorded species richness, but gives more emphasis to the Suez Canal area, western Mediterranean coast, both sides of the Suez Gulf and a narrow strip across the northern part of the Nile Delta. Gathering information from other studies discussing the species richness pattern of different taxonomic groups in Egypt is very helpful to show which areas share highest biodiversity or require more protection. Although produced with different methodologies, adding up species richness maps of this study to those of butterflies and mammals (Gilbert & Zalat 2008; Basuony et al. 2010) indicates the most important biodiversity areas. Using the accumulated pattern of species richness of these three groups, the hotspots of biodiversity were at the periphery of greater Cairo, in the high mountain areas of South Sinai, the coastal areas of the Aqaba and
- 110 - Suez gulfs, the Suez Canal area and the narrow strip on the Mediterranean coast from Rafah to Sallum (Fig. 45). A separate study is probably needed to focus on producing such a map using one consistent methodology and a greater variety of species groups, to show areas of highest species richness across Egypt.
Fig. 45: Accumulated species richness map of three taxonomic groups (butterflies, mammals, and reptiles), showing areas of high species richness. Species richness maps for butterflies and mammals were obtained from the results of (Gilbert & Zalat 2008; Basuony et al. 2010)
There are no published studies known to me that discuss the effect of climate change on Egyptian reptiles (see above), obviating any comparison of main findings of this study to others. Information on the reptiles in adjacent countries (e.g. Sudan and Libya) is very limited, making it not possible to compare patterns of species distributions, or make assumptions about possible compensations or migrations of Egyptian reptiles as a result of climate change. Areas predicted to lose a high number of species in the future (and so potentially require more attention; assuming either dispersal abilities) are the Suez Canal area, coastal areas of both Suez and Aqaba gulfs, Wadi El-Natrun, around the greater Cairo, Siwa oasis and small inland wadis of the Red Sea. Other areas are predicted to benefit from climate change, increasing their number of species (assuming unlimited dispersal); these include the area between the west Mediterranean coast to the Qattara Depression, middle to north Sinai, Red Sea coastal areas, Western Desert oases, and southern parts of the Nile Valley (for more details, see above). This indicates that some areas
- 111 - with currently high predicted species richness may lose many species in the future; some of these areas are already under a certain degree of protection and so it might be easier to maintain them in the future (e.g. Siwa oasis). Others (e.g. Wadi El-Natrun, the Suez Canal area and Red Sea inland wadis) are unprotected and measures are needed to conserve them (see later). The Qattara Depression may benefit from species shifting away from Siwa and Wadi El-Natrun; some species from inland Red Sea wadis are predicted to shift eastwards to Red Sea coastal areas. This is not likely to happen in the near future because of the limited dispersal ability of most Egyptian reptiles. For a country like Egypt with limited resources and budgets dedicated to wildlife conservation, attention and effort may have to be concentrated in important areas that are predicted to lose species in the future, rather than expanding the current Protected Areas network to cover those areas predicted to increase species richness in the future, especially since most reptiles probably cannot track suitable climatic conditions in the near future. Some areas with increased species richness in the future are not well-investigated yet (e.g. the Qattara Depression) and more research is required to indicate their need for protection. There is only one comparable study of the impact of climate change on Egyptian taxa - that of Leach (2011) on mammals and butterflies. The predicted future changes in species richness as a result of climate change from my study do not seem to concur with Leach’s. Mammal species richness is predicted to decline in the Mediterranean and Red Sea areas (40-60%) and increase elsewhere (80-100%); while for butterflies almost all of Egypt is predicted to decline (40-60%) except southern Egypt which is predicted to increase (40-60%) (Leach 2011). Species richness by itself is not adequate as an indicator of biotic change. It is possible to maintain the same number of species in the future, yet change in species composition. The largest change in species composition in the future is predicted to be in the great area of the Western Desert (including the oases, El-Gilf El-Kebir area, and the area between Wadi El-Natrun, the Mediterranean coast, the Qattara Depression and Siwa oasis), inland wadis of the Eastern Desert and Gebel El-Hallal area. No other studies have discussed
- 112 -
the potential change in future species composition in Egypt as a result of
climate change.
Fairly similar results are evident in other studies performed on different continents or taxonomic groups. In his study on the effect of climate change on the European reptiles, de Pous (2011) predicted high species loss and turnover in a priority conservation hotspot in Europe (the Iberian Peninsula), with great variation in the predicted patterns of future species richness and species loss across different future projections. The same study predicted high species turnover in most parts of Europe except the central areas (assuming unlimited dispersal), while such areas under limited dispersal were restricted to three relatively small areas (de Pous 2011). Ihlow et al. (2012) predicted a strong effect of climate change on global chelonian species richness, with the highest impact on four current chelonian hotspots, possibly because of non- analogous climate conditions in the future. According to Maiorano et al. (2011), mammal species richness of the Mediterranean basin is predicted to have important changes in the future due to climate change; with highest predicted species richness decline and highest species turnover in Spain, North Africa and the Middle East. In another study, Currie (2001) predicted the effect of climate change on species richness patterns in trees and different vertebrate groups in the conterminous United States. Climate change had a variable effect on different groups: the response of trees was variable, but severe effects were predicted on birds and mammals, while potential benefits were predicted for reptiles and amphibians (Currie 2001). A combination of a temperature increase of 3° C and a 20% decline in rainfall was predicted to lead to the loss of about 15% of species richness in the tropical forests of Mesoamerica (Golicher et al. 2012).
Range Changes & important species for conservation Two species are predicted to loss their entire suitable habitats in at least one future projection: Tarentola mindiae and Hemidactylus robustus. Tarentola mindiae is a near endemic species recorded just from northwest Egypt and northern Cyrenaica (eastern Libya); its distribution in Egypt is restricted to Siwa oasis, the Qattara Depression and their periphery (Baha El Din 2006a -
- 113 - see Fig. 46(a)). According to the IUCN, it is classified globally as Least Concern (Baha El Din 2006c), and nationally as Vulnerable in this study (due its limited number of locations). From the future range changes predicted in this study, it is classified as Endangered by 2020 and as Critically Endangered by 2050 and 2080 (under all emission scenarios and dispersal assumptions). Modelling the current distribution of Tarentola mindiae shows that its current suitable areas are at Siwa oasis and the Qattara Depression, with unconfirmed possible suitability in Wadi El-Natrun, El-Omayed protectorate, and Lake Qarun & Wadi El-Rayan protectorates in the Fayoum area. Further investigation on the existence of Tarentola mindiae in Fayoum area and Wadi El-Natrun is required, although it is unlikely to be found there due to extensive collecting efforts done in these areas without recording it. The existing Protected Area of Siwa has a high conservation responsibility for Tarentola mindiae. Constructing a new Protected Area in the easternmost part of the Qattara Depression (or expansion of El-Omayed protectorate southwards) seems to be important to conserve this species. Hemidactylus robustus is a localized species that ranges from East Africa coast from Zanzibar to southern Egypt, Arabia, east to Pakistan; its distribution in Egypt is on the Red Sea coast from El-Quseir southwards (Baha El Din 2006a - Fig. 46(b)). Hemidactylus robustus has not been classified yet for the IUCN Red List, and classified nationally as Vulnerable based on its limited number of locations. Under A2a scenario, it is classified as Critically Endangered by 2020 and 2050 and predicted to maintain part of its lost distribution by 2080 (assuming either dispersal assumptions); while under B2a scenario, it is classified as Endangered by 2020 and as Critically Endangered by 2050 and 2080 (assuming either dispersal assumptions). More than half of its current distribution in Egypt is located within Wadi El-Gemal and Gebel Elba Protected Areas, which indicates that it is hypothetically protected. Both protectorates (northwards towards Safaga on the Red Sea coast) have great responsibility to conserve this species, and such threatened species should be taken in consideration before giving permission for any new development activities (e.g. tourism) near the Red Sea coast, especially in Wadi El-Gemal protectorate. If it is recorded there at high prevalence, a suggested expansion of either Protected Areas is required to cover unprotected coastal zone
- 114 -
between both protectorates (north and south of Berenice), although the
protection of this area should already be the concern of both protectorates as
it is located very close to their buffer zone. No information is available on the
distribution or the status of Hemidactylus robustus in the coastal areas of
Sudan, so possible compensation from the south is not clear.
Other than Tarentola mindiae, there are other eleven endemic/near-
endemic reptile species. Three are endemic to Egypt: Hemidactylus foudaii,
Mesalina bahaeldini and Tropiocolotes bisharicus; and eight are near-endemic
to Egypt: Acanthodactylus aegyptius, A. pardalis, Philochortus zolii, Platyceps
sinai, Telescopus hoogstraali, Testudo kleinmanni, Trapelus savignii and
Tropiocolotes nubicus. Mesalina bahaeldini is a common and widespread
species that has a world distribution confined to high mountains of South Sinai
(Baha El Din 2006a). It is classified as Least Concern by IUCN (Werner &
Baha El Din 2006a) and as Vulnerable in this study (because of limited
number of locations). Climate change is predicted to have mild effect on its
distribution, ranging from up to 50% suitability gain (assuming unlimited
dispersal) to up to 50% suitability loss (‘Endangered’ - assuming no dispersal).
Hemidactylus foudaii is a rare and localized species that has a world distribution restricted to the Gebel Elba region (Baha El Din 2006a). It is classified by IUCN as Least Concern (Baha El Din 2006b) and was not assessed nationally in this study due to its limited number of records, although it probably should be classified at least as Vulnerable due to its limited number of locations. It was not possible to assess the potential effect of climate change on the distribution of Hemidactylus foudaii due to the limited number of available records.
Tropiocolotes bisharicus has a distribution confined to southeast of Egypt, although its range is likely to extend southwards into Sudan along the Red Sea (Baha El Din 2006a). It has not yet been classified for the IUCN Red List, and was classified as Vulnerable in this study. Climate change seems to have mild effect on its future distribution that ranges from small gain (up to 30% - assuming unlimited dispersal) to up to 30% loss of its suitable habitats (‘Least Concern’), reaching up to 50% loss (‘Vulnerable’) by 2080 under A2a scenario (assuming no-dispersal). Almost all the world distribution of Mesalina - 115 - bahaeldini is within the St. Katherine Protectorate; this gives great responsibility to St. Katherine to conserve the species. Gebel Elba Protectorate has the responsibility for conserving the whole world range of both Hemidactylus foudaii and Tropiocolotes bisharicus. The status of the Egyptian tortoise, Testudo kleinmanni, is a well-known issue in Egypt wildlife conservation, and several studies have been carried out within the last ten years on its conservation status (e.g.: Geffen 1990; Baha El Din et al. 2003; Perälä 2006; Attum et al. 2007). Testudo kleinmanni is a near- endemic species restricted to southeastern corner of the Mediterranean, extending as a narrow strip from the western Negev in the east westwards to the Gulf of Sirt in Libya (Geffen 1990; Baha El Din 2006a). It is one of the smallest and most endangered tortoise species in the Mediterranean basin of North Africa and the Middle East, with the most restricted range of all tortoises in this area (Attum et al. 2007). All available records for this species from Egypt (including historic records) are shown in Fig. 46(k). The current realized range of this species is currently very limited, as it has been subjected to severe pressures in the near past causing the extirpation of its former distribution range (Baha El Din 2006a). Reasons for its range reduction include severe over-grazing, over-collecting for pet-trade, massive reclamation schemes, and extensive habitat destruction accompanied with coastal development for tourism and urban expansion (Geffen 1990; Baha El Din 2006a; Attum et al. 2007). It is considered by some authors as effectively extinct within Egypt between the early 1970s and the early 1990s (Perälä
- until two female tortoises were confiscated by an animal collector inside El-Omayed Protectorate in 2003 (Attum et al. 2007). It seems still to exist in the wild in low populations in two Protected Areas; Zaranik and El-Omayed (Mindy Baha El-Din, pers. comm.). It is classified globally by IUCN as Critically Endangered [A2abcd+3d] (Perälä 2003) and as Vulnerable (D2) in this study (just owing to its very limited number of locations). In this study, all available Egyptian records of Testudo kleinmanni were used to model its suitable habitats in the current and future. The results show low impact of future climate change on the distribution of Testudo kleinmanni; the effect ranges from gaining up to 50% more than its historical distribution (assuming unlimited dispersal) to the loss of less than 30% of its historical distribution
- 116 - (‘Least Concern’ - assuming no-dispersal). This shows that climate change is not really a major threat, except possible future habitat destruction from the predicted rise in sea level of the Mediterranean Sea. Suitable areas for the distribution of Testudo kleinmanni (shown in Fig. 46-k) should not be interpreted as the current predicted distribution of it, but may be considered as potential areas for re-introduction. The current status of the Egyptian tortoise in Libya is not clear, although it seems to exist there in higher numbers than in Egypt. The major threat for the Libyan populations seems to be over-collection for the pet-trade, with several confiscations of dozens of individuals in pet markets (Mindy Baha El-Din, pers. comm.). There are a number of captive- breeding tortoises in two enclosures in Egypt where upward of 70 tortoises breed annually (Mindy Baha El-Din, pers. comm.). A separate study is needed using the Egyptian tortoise’s available records from Egypt and the adjacent countries to construct a robust model that show the most effective locations for re-introduction. In such a study, layers indicating the habitat status of the study area (the Mediterranean coast area extending from the Negev towards the Libyan Mediterranean coast) should be used if available. Such layers include current land-uses (e.g. agriculture, tourism, etc.), protection status (protected/non-protected), and the habitat destruction status. Areas which are not suitable any more for re-introduction should be excluded as not to interfere with the model; this includes some areas on the Egyptian Mediterranean coast that have already suffered from severe habitat destruction in the past, and areas with high human populations. Trapelus savignii has a very small world range, existing in just Egypt, Palestine and Israel, with most of its world distribution lying within Egypt (Baha El Din 2006a). Its distribution in Egypt is narrow, occupying the area from east of Cairo eastwards through northern Eastern Desert towards much of north Sinai (Baha El Din 2006a). It is classified as Vulnerable by IUCN (Werner & Baha El Din 2006b) and also nationally in this study. Potential impacts of climate change on the distribution of Trapelus savignii seem to be minor; ranging from gaining up to more than 100% of its current occupancy (assuming unlimited dispersal) to the loss of less than 30% of its current occupancy (‘Least Concern’ - assuming no dispersal).
- 117 -
Acanthodactylus pardalis has a restricted distribution confined to
northwest Egypt and northern Cyrenaica in Libya; its distribution in Egypt is
narrow, extending from Cairo and Fayoum northwestwards towards Sallum,
across the Mediterranean coast (Baha El Din 2006a). It is classified as
Vulnerable by IUCN (Böhme & Baha El Din 2006a) and also nationally in this
study. This species is considered one of Egypt’s most threatened reptiles
species and has disappeared recently from its previous distribution (Baha El
Din 2006a). Habitat degradation and loss (because of large-scale plowing for
winter cereals agriculture and extensive tourism development), over-collection
pressure and over-grazing are the major factors causing the destruction of its
suitable habitats between Alexandria and Mersa Matruh, leaving reasonably
healthy populations just between Mersa Matruh and Sallum (Baha El Din
2006a). Potential impacts of climate change on the distribution of
Acanthodactylus pardalis seem to be minor; ranging from gaining up to 80%
(assuming unlimited dispersal) to the loss of less than 30% of suitable habitats
(‘Least Concern’ - assuming no dispersal).
Acanthodactylus aegyptius has a restricted distribution that is confined to Egypt, northwest of the Negev and the Gaza strip; its distribution in Egypt includes North Sinai, the northernmost part of the Eastern Desert, throughout the northern Western Desert (including Bahariya and Kharga oases, Wadi El- Natrun and the easternmost edge of the Qattara Depression), and along the west periphery of the Nile Valley (Baha El Din 2007); and has been recently recorded from the west of Farafra Oasis (Werner & Ashkenazi 2010). It has not been assessed yet for IUCN Red List, possibly because of its recent recognition as new species by (Baha El Din 2007), and is classified as Least Concern in this study. A moderately high impact of climate change on the distribution of Acanthodactylus aegyptius is predicted, with a potential loss of up to 50% of its current suitable habitat by 2050 (‘Endangered’ - under all emission scenarios and dispersal ability assumptions), reaching up to 80% loss by 2080 under the A2a scenario (‘Critically Endangered’ - no-dispersal assumption).
Philochortus zolii is recorded from just three widely separated locations in Egypt and southwest Libya, with a very restricted distribution in Egypt confined to very small saltmarsh tracts at Wadi El-Natrun area (Baha El Din - 118 -
2006a). It is classified by IUCN as Critically Endangered [B1ab(iii)] because of
its presumably small extent of occurrence (<100 km
2
), its fragmented
distribution, and severe habitat destruction and quality decline in Egypt and
presumably in Libya (Böhme & Baha El Din 2006b). It is considered to be the
most threatened reptile species in Egypt, due to imminent habitat destruction
of Wadi El-Natrun area and the pressure of over-collection (Baha El Din
2006a).
Telescopus hoogstraali has a small global geographical distribution including just Sinai, the Negev and Jordan; with a rare and localized distribution in Egypt confined to St. Katherine area and Gebel El-Maghara in Sinai (Baha El Din 2006a). It is classified by IUCN as Endangered [B1ab(iii)] because of its small extent of occurrence (<5000 km 2 ), its fragmented distribution, and the continuing decline in the extent and habitat quality (Disi et al. 2006).
Tropiocolotes nubicus has a world distribution confined to Egypt and Sudan; with a restricted distribution immediately bordering the southern part of the Nile Valley (Baha El Din 2006a). It is classified as Data Deficient by IUCN as it has been recently described and its full range, status, threats and ecological requirements are uncertain (Bohm & Richman 2010).
Platyceps sinai is restricted to a small geographic area including just South Sinai, the Negev and Jordan; it has a rare and localized distribution in Egypt and considered a near threatened species in Egypt (Baha El Din 2006a). It has not been yet classified for IUCN Red List.
Available number of records for each of Philochortus zolii, Telescopus hoogstraali, Tropiocolotes nubicus and Platyceps sinai was inadequate to involve them in this study analyses; and hence their national Red Data List status and the potential impacts of climate change on their distribution can not be assessed.
Zaranik and El-Omayed protectorates have the responsibility to conserve the remaining populations of Testudo kleinmanni and to urge the halting of habitat degradation around their peripheries. Zaranik is the only Egyptian protectorate (with the possible exception of Al-Ahrash) that contains Trapelus savignii, giving it greater responsibility to conserve this species. The - 119 - current distribution of Acanthodactylus aegyptius is included in a two already established Protected Areas, Zaranik and Wadi El-Rayan, which means that the populations at those protectorates are potentially protected. The construction of Protected Areas in Wadi El-Natrun and the easternmost part of the Qattara Depression can offer an additive help in its conservation. The current limited distribution of Acanthodactylus pardalis in Egypt does not include any Protected Areas. The construction of a Protected Area on the Mediterranean coast between Mersa Matruh and Sallum seems to be essential for its conservation. Alternatively, the expansion of the recently declared marine protectorate, El-Sallum Gulf to cover threatened habitats along the western part of the Mediterranean coast could be a measure to protect this species. The construction of a Protected Area in the Wadi El- Natrun area seems to be essential for the conservation of Philochortus zolii. Protected Areas of South Sinai have the responsibility for the conservation of Telescopus hoogstraali and Platyceps sinai; with a possible need to construct a new Protected Area in the region of Gebel El-Maghara close to Gebel El- Hallal in North Sinai to conserve Telescopus hoogstraali. The current distribution of Tropiocolotes nubicus does not include any Protected Areas, and so it is assumed not to be protected. Unfortunately, the construction of a new Protected Area in the area of lower Nile seems not to be possible due to the high number of inhabitants and its extensive agricultural activities. Eight species are classified as Critically Endangered in at least one mean future projection: Acanthodactylus longipes, Cerastes vipera, Eryx jaculus, Eumeces schneiderii, Malpolon moilensis, Ptyodactylus guttatus, P.siphonorhina and Trapelus mutabilis (For detailed results, see above). The conservation of these species should be considered in Egypt’s conservation management plans. Maps showing the current distribution of each of these species and their predicted suitable habitats are shown in Fig. 46. In this study, three reptile species are classified nationally, according to IUCN red list, as Endangered: Chamaeleo africanus, Leptotyphlops cairi and Uromastyx ocellata. The three species have a narrow distribution in Egypt and globally. First two are not classified yet by IUCN, while the third, Uromastyx ocellata, is classified as Least Concern (Spawls 2011). There is no predicted impact of climate change on their future distributions, except for Uromastyx
- 120 - ocellata which is predicted to loss up to 50% (Vulnerable) of its distribution by 2080 due to climate change. Other studies discussing predicted future range changes as a result of climate change show fairly similar results. Araújo et al. (2006) predicted less deleterious impacts of climate change than expected beforehand for the amphibia and reptiles of Europe, with most species tending to expand their distributions in the future (assuming unlimited dispersal) due to the warming of the cooler northern parts of Europe, allowing new colonization. They suggested that the highest loss of suitability, assuming unlimited dispersal, was in the south-western parts of Europe (including the Iberian Peninsula), while south-eastern parts were predicted high species gain. Under the perhaps more realistic assumption of no dispersal, most species were predicted losses in habitat suitability by 2050 (Araújo et al. 2006). In a more recent relevant study, de Pous (2011) predicted that under unlimited dispersal, 50% of European reptile species should tend to expand in the future, while assuming no dispersal predicted that 78% of species would contract. de Pous (2011) suggested that eight (unlimited dispersal) or 21 (no dispersal) speceis would become extinct in at least one future projection, and a further two were consistently projected to undergo > 80% loss of their currently suitable habitats (Iberolacerta bonnali and Podarcis tiliguerta). Compared with the predicted responses of Egyptian reptiles in this study, de Pous (2011) suggests that European species will suffer more from climate change. There are no other comparable studies from North Africa or the Middle East. From their comprehensive study of the potential responses of terrestrial mammals in the Mediterranean basin to climate change, Maiorano et al. (2011) suggested that a substantial number of Mediterranean mammals will be severely threatened by 2100, particularly some endemics. Assuming unlimited dispersal, responses ranged between 100% loss to substantial gains (almost ten-fold) of currently suitable habitats. One species was predicted to lose its entire suitable habitat across all scenarios and models, and 16 species were predicted to become extinct in at least one future projection (Maiorano et al. 2011). As before, assuming no dispersal led to greater losses and more modest gains, with 21 species predicted to lose their entire suitable habitats in at least one future projection (Maiorano et al. 2011).
- 121 - The study by Ihlow et al. (2012) predicted that climate change would cause the range contraction of 86% of the global chelonian species, with about 12% of the species predicted to be completely located outside their current realized niche. Sauer et al. (2011) suggested a predicted average range loss of 70-80% of freshwater assemblages within low mountain ranges by 2080; range change varied from almost complete loss to modest gains of currently suitable habitats. Similar results were found by Domisch et al. (2011) on macroinvertebrates of the submontane regions of Central Europe, with predicted extinction of one species to substantial range gains of others. Ben Rais Lasram et al. (2010) predicted a severe impact of climate change on a large number of Mediterranean fish species, with 25 and 45 species predicted to be listed on the IUCN Red List by 2060 and 2100, respectively. They predicted that 6 and 14 fish species would become extinct by 2060 and 2100, respectively, and that the ranges of endemic species would become highly fragmented in the future.
Area prioritization for conservation & Protected Areas coverage In my study, Protected Areas had higher mean prioritization value (currently, and in the future) compared to outside the protectorates using both additive benefit and core area functions of the Zonation algorithm. Using additive benefit function, areas with current high prioritization value are the Suez Canal area, the Nile Valley and its Delta, the Qattara Depression, high elevation wadis in South Sinai, and the coastal areas of the Red Sea, the Mediterranean Sea and the Aqaba & Suez Gulfs. These areas also show high species richness, reflecting the nature of additive benefit function which gives more weight to locations with high species richness (Moilanen et al. 2012). Using core-area function, areas with current high prioritization values are high elevation wadis in South Sinai, Gebel Elba, Siwa oasis, the Suez Canal area, the Red Sea coast, the Mediterranean coast and the Nile Valley. There is not much difference between the overall patterns of areas of high prioritization value using either function, with overall higher prioritization value using additive benefit function (especially along the north Mediterranean coast from Sallum to Rafah, Wadi El-Natrun, Suez Canal area and around Cairo). This
- 122 -
result is not as expected; using core-area function should identify important (or
poor) locations where a single or a few species have important occurrences
(Moilanen et al. 2012), and this should not be affected by the pattern of
species richness unless these high species-richness locations also contain
important occurrences of single or few species.
In the future, a higher prioritization value is predicted to be given to the
area between Bir Abraq and Gebel Elba, Gebel El-Gallala southwards,
Western Desert oases, western part of middle Sinai and an area extending
from inland areas of the Mediterranean coast to the Qattara Depression. It is
predicted also that areas outside the protectorates will have higher mean
prioritization value in the future (especially using Additive benefit function),
although the mean prioritization value of Protected Areas is predicted to
remain higher than outside them (Figs. 42, 43).
Although Protected Areas show higher mean prioritization value than outside them, Egypt’s Protected Area network seems to be inadequate to conserve Egyptian reptiles. New Protected Areas may be needed to cover unprotected areas with high species richness in the middle to north Sinai (especially around the Gebel El-Hallal area), the Suez Canal area, both sides of Suez Gulf and Red Sea inland wadis between Hurghada and Mersa Alam. Stricter protection is required in already-established Protected Areas, including Gebel Elba, Siwa oasis and the Protected Areas in South Sinai. In the future, further protection is needed in areas of the Suez Canal, coasts of South Sinai, Wadi El-Natrun, and Red Sea inland wadis; and stricter protection measures in already established Protected Areas, such as Siwa and Gebel Elba. Regarding the potential future change in species composition, more attention should be given to the already-established Protected Areas predicted to have high species turnover in the future, e.g. Siwa oasis and El-Gilf El- Kebir. More attention is also needed in areas with current high species richness which are predicted to have high species turnover in the future, such as Wadi El-Natrun, the Gebel El-Hallal area and inland wadis in the Red Sea: these areas may need new Protected Areas. Special concern should be given as well to unprotected areas with high prioritization value; such as the Suez Canal area, the Qattara Depression, and the coastal areas of the Red Sea, the - 123 - Mediterranean Sea and the Aqaba and Suez gulfs. For already-established Protected Areas having high prioritization value, stricter protection is required to maintain these areas, e.g. Gebel Elba, the Protected Areas in South Sinai and the Protected Areas near Western Desert oases. More fieldwork is required to show the current status of areas predicted to have increased prioritization value in the future, to evaluate their conservation status and show if any of these areas should be declared as new Protected Areas; e.g. Gebel El-Gallala, Gebel El-Hallal and the Qattara Depression. Further fieldwork is also needed in unprotected areas that contain threatened species, to show if any of these areas are worth considering as new protectorates. The Qattara Depression seems to be one of these areas, considered important for the conservation of Acanthodactylus aegyptius, A. longipes, Cerastes vipera, Eumeces schneiderii, Malpolon moilensis, Ptyodactylus siphonorhina, Tarentola mindiae and Trapelus mutabilis. Other such sites are Wadi El- Natrun for Acanthodactylus aegyptius, Cerastes vipera, Malpolon moilensis, Philochortus zolii, Ptyodactylus siphonorhina and Trapelus mutabilis (and possibly Tarentola mindiae); the Gebel El-Hallal area for Cerastes vipera, Malpolon moilensis and Ptyodactylus guttatus (and possibly Telescopus hoogstraali); the Suez Gulf coasts for Malpolon moilensis, Cerastes vipera, Hemidactylus robustus and Ptyodactylus guttatus; and the western part of the Mediterranean coast (between Mersa Matruh and Sallum) for the conservation of Acanthodactylus pardalis. The area of the Qattara Depression is unexplored and not easy to access. It is one of a number of proposed new Protected Areas characterized by high habitat diversity and very high degree of threat (Baha El Din 1998). It has the highest priority among the proposed protectorates, as it supports considerable biodiversity including rare and endangered species, and is considered the last refuge for several endangered key species for Egypt and internationally (Baha El Din 1998), despite the paucity of published information on the status of its fauna and flora in the literature. The coastal areas of the Suez Gulf seem to be an important area for both of reptiles and mammals, and also birds; It is considered an important path of bird migration and three important bird areas are located in this area (Ain Sukhna, El-Qaa plain and Gebel El-Zeit) (Baha El Din 1999, 2001; Basuony et al. 2010), with no
- 124 - Protected Areas on either sides. The area of Ain Sukhna and its periphery has been subjected to great pressure in the last 15 years: the high levels of air pollution from tonnes of dust spewed out daily after the construction of a large cement factory has caused the death of desert vegetation within a radius of 15 km (Baha El Din 1999, 2001); rapid habitat degradation from fast-growing tourism, overgrazing and quarrying; and unplanned new government projects such as the new shipping port, airport, large tourism-associated activities and projects and a heavy-industry zone. All these are thought to jeopardize wildlife (Baha El Din 1999, 2001) in the Ain Sukhna area. The El-Qaa plain (on the eastern side of Suez Gulf) is important for the conservation of the Dorcas gazelle, as it represents a refuge for the largest remaining population in Sinai, and is also subject to unplanned coastal tourism development (Baha El Din 1999, 2001). The conservation importance of the Suez Gulf coastal areas should be considered before giving permissions to construct new industrial or tourism activities; otherwise the same fate of habitat destruction as happened on the Mediterranean coast will occur in this area. The Suez Canal area (especially the western side) shows high human population and agricultural activity, which may militate against the construction of new Protected Areas in this area. The eastern side of the Suez Canal seems to have many fewer sources of disturbance, which makes it potentially suitable for a new Protected Area after verification of the current status of its biodiversity. Gathering information from other studies discussing the effectiveness of Egypt‘s Protected Areas network to cover important biodiversity hotspots can help to show which areas require more protection across different taxonomic groups. According to Gilbert & Zalat (2008) and Basuony et al. (2010), the network seems to be adequate to conserve Egyptian butterfly hotspots, except the Mediterranean coast between Alexandria and Sallum, but inadequate to conserve important mammal areas, with new Protected Areas needed to be constructed in the lower Nile Valley, along the north coast between Alexandria and Sallum, top part of the Suez Gulf, and perhaps the Qattara Depression. The findings of Basuony et al. (2010) are consistent, to some extent, with the results of this study, with the need to construct new Protected Areas at the coasts of Suez Gulf, Mediterranean coast (from Mersa Matruh to Sallum) and the Qattara Depression.
- 125 - Some other studies have used Zonation to prioritize areas for conservation, but using other taxa. Simaika (2012) suggested that the Protected Area network in Africa is not enough to conserve its dragonflies, with a need for the protection of an additional 5% of the area of Africa. Klorvuttimontara (2010) demonstrated that about 50% of areas of high prioritization value in Thailand are currently protected, and that will not change very much in the future. He predicted that the conservation value of the Protected Areas of Thailand to conserve butterflies should remain similar in the future, although increasing the quality of existing Protected Areas would enhance the conservation status of the butterflies species in Thailand. Tognelli et al. (2011) showed that the Protected Area network of Argentina contain only a limited number of Xenarthran mammals, so more Protected Areas are needed to conserve them. However, areas of high prioritization identified by Zonation were not suitable for new Protected Areas because they are owned by private landowners (Tognelli et al. 2011). Simialrly Corbalán et al. (2011) suggested that the current reserve network of Argentinean Patagonia is not enough to conserve its lizard species. de Pous et al. (2011) used the ConsNet Prioritization algorithm to assess the effectiveness of the Conservation Area network in Morocco to conserve the herpetofauna under the current climate. They demonstrated the need for a major increase in the network to guarantee their conservation. In Araújo et al.’s study of the effectiveness of Protected Areas and the Natura 2000 networks to conserve European plant and terrestrial vertebrates under climate change, 58% and 63% of these species were predicted to be lost by 2080 from Protected Areas and Natura 2000 areas, respectively. Birds and mammals were predicted to have a higher proportion of losers than winners inside Protected Areas in all future scenarios, whilst there was a variable response of amphibians and an increased suitability for reptiles. The Natura 2000 sites were less effective in conservation compared to Protected Areas, although both were predicted to perform better than elsewhere, particularly if more conservation efforts were given to biodiversity conservation specifically against climate change (Araújo et al. 2011). Maiorano et al. (2011) demonstrated that the current Protected Areas of the Mediterranean basin will strongly be affected by climate change, with highest predicted number of lost
- 126 - species (25-43%) located in the drier Protected Areas of North Africa, the Middle East and Spain. Coetzee et al. (2009) showed that the South African Important Bird Areas are expected to be inadequate to conserve endemic birds under climate change, with severe range changes predicted in the future. They suggest the need for new Important Bird Areas and Protected Areas to conserve the refugia of these species (Coetzee et al. 2009).
The limitations of projecting into the future Using species distribution models for extrapolations is risky and must be treated carefully (Elith et al. 2010; Araújo & Peterson 2012). Possible uncertainties resulting from extrapolation beyond the limits of training data can be assessed from the calculation of the ‘Multivariate Environmental Similarity Surfaces’ (MESS), available in recent versions of Maxent (Elith et al. 2010). MESS is a measure of the similarity of any given pixel to a reference set of pixels of chosen predictor variables; it is used to determine novel climates by giving negative values for dissimilar pixels where at least one variable has a value outside the training range (Elith et al. 2010; Elith et al. 2011). Novel climates can then be used as a mask to inhibit the use of certain areas by the models, or as a measure of prediction uncertainty (Elith et al. 2010). In my study, MaxEnt produced MESS maps at each possible combination of global circulation models, emission scenarios and time slices. The mean MESS value across different global circulation models in Egypt is shown in Fig. 47; depicting which areas are predicted to have novel climate conditions in the future at each possible combination of emission scenarios and time slices. MESS output maps are continuous maps with values ranging from -100 (red) to +100 (blue). Positive values indicate similar future climate conditions compared to the current (the darker the blue, the higher is the similarity), while the negative values indicate locations of dissimilar climate compared to the current (the darker is the red, the higher dissimilarity). Under both emission scenarios at 2020 and 2050 and under the B2a scenario at 2080, areas with predicted non-analogue climates (i.e. the most dissimilar novel climates) are located at east of El-Gilf El-Kebir, the Red Sea coast from Mersa Alam southwards, south of Wadi El-Allaqi near the Sudanese borders, near Qena,
- 127 - and over small patches on the Mediterranean coast and Suez Canal area. By 2080 and under A2a scenario, larger areas are predicted to be subjected to novel climates, with much higher degree of dissimilarity extending to a high proportion of the Western Desert (including the oases), larger coastal areas on the Red Sea (from south of Hurghada southwards), eastern and northern Sinai, the Suez Canal area and around Cairo. The Protected Areas of Wadi El- Gemal, Gebel Elba and Wadi El-Allaqi (and possibly Siwa, Taba and Zaranik) are therefore susceptible to potential novel climates in the future. Future projections in areas of high future climate dissimilarity should be interpreted with caution.
Conclusion My results suggest that about 10% of the Egyptian reptiles will suffer greatly in the future due to climate change. Eight species are predicted to lose up to 80% of their suitable habitats in the future. The current network of Egyptian Protected Areas appears to be inadequate to conserve Egyptian reptiles; new Protected Areas are probably needed at Wadi El-Natrun, the Qattara Depression, eastern side of Suez Canal, Gebel El-Gallal area, coastal areas of Aqaba Gulf, western Mediterranean coasts between Mersa Matruh and Sallum, and Gebel El-Hallal area in North Sinai. More strict protection is required in the Protected Areas of St Katherine, Siwa oasis and Gebel Elba.
- 128 -
(a) Tarentola mindiae (b) Hemidactylus robustus
(c) Eumeces schneiderii (d) Malpolon moilensis
(e) Trapelus mutabilis (f) Ceraster vipera
Fig. 46: Maps showing actual distribution and suitable distribution areas for some Egyptian reptile species.
- 129 -
(g) Eryx jaculus (h) Ptyodactylus guttatus
(i) Acanthodactylus longipes (j) Ptyodactylus siphonorhina
(k) Testudo kleinmanni
Fig. 46 cont. Maps showing actual distribution and suitable distribution areas for some Egyptian reptile species.
130 - A2a
2020 2050 2080 B2a
2020 2050 2080
Fig. 47: Average MESS (Multivariate Environmental Similarity Surfaces) maps of different global circulation models showing areas of future novel climates.
Colours ranges from blue (Positive values - similar future climate conditions compared to the current; the darker the blue, the higher is the similarity) to Red (negative values - locations of dissimilar climates compared to the current; the darker is the red, the higher dissimilarity). Results at the areas marked with dark red colour (non- analogous climates) should be interpreted with caution.
- 131 - References
Alkemade R., Bakkenes M. & Eickhout B. (2011) Towards a general relationship between climate change and biodiversity: an example for plant species in Europe. Regional Environmental Change 11: 143-150 (DOI: 10.1007/s10113-010-0161-1). Anderson R.P. (2003) Real vs. artefactual absences in species distributions: tests for Oryzomys albigularis (Rodentia: Muridae) in Venezuela. Journal of Biogeography 30: 591-605. Anderson R.P., Lew D. & Peterson A.T. (2003) Evaluating predictive models of species’ distributions: criteria for selecting optimal models. Ecological Modelling 162: 211-232 (DOI: 10.1016/s0304-3800(02)00349-6). Anderson R.P., Peterson A.T. & Egbert S.L. (2006) Vegetation-index models predict areas vulnerable to purple loosestrife (Lythrum salicaria) invasion in Kansas. Southwestern Naturalist 51: 471-480. Araújo M.B., Alagador D., Cabeza M., Nogues-Bravo D. & Thuiller W. (2011) Climate change threatens European conservation areas. Ecology Letters 14: 484-492 (DOI: 10.1111/j.1461-0248.2011.01610.x). Araújo M.B. & New M. (2007) Ensemble forecasting of species distributions. Trends in Ecology & Evolution 22: 42-47 (DOI: 10.1016/j.tree.2006.09.010). Araújo M.B. & Pearson R.G. (2005) Equilibrium of species’ distributions with climate. Ecography 28: 693-695 (DOI: 10.1111/j.2005.0906- 7590.04253.x). Araújo M.B. & Peterson A.T. (2012) Uses and misuses of bioclimatic envelope modeling. Ecology 93: 1527-1539 (DOI: 10.1890/11-1930.1). Araújo M.B. & Rahbek C. (2006) Ecology. How does climate change affect biodiversity? Science 313: 1396-1397 (DOI: 10.1126/science.1131758). Araújo M.B., Thuiller W. & Pearson R.G. (2006) Climate warming and the decline of amphibians and reptiles in Europe. Journal of Biogeography 33: 1712-1728 (DOI: 10.1111/j.1365-2699.2006.01482.x). Attum O., Esawy M.M., Farag W.E., Gad A.E., Baha El Din S.M., et al. (2007) Returning them back to the wild: movement patterns of repatriated Egyptian tortoises, Testudo kleinmanni Loetet, 1883 (Sauropsida, Testudinidae). Zoology in the Middle East 41: 35-40. Baha El Din S.M. (1998) Towards establishing a network plan for protected areas in Egypt - Draft consultative document. Nature Conservation Sector (NCS), Egyptian Environmental Affairs Agency (EEAA) - (EU/GOE PROJECT/ SEM/D4/22D/027A: Egypt).
- 132 - Baha El Din S.M. (1999) Directory of Important Bird Areas in Egypt, 113 pp. BirdLife International and Palm Press. ISBN 9-7750-8925-5. Baha El Din S.M. (2001) Egypt. Pages 241-264 in Fishpool LDC, Evans MI, eds. Important Bird Areas in Africa and associated islands: Priority sites for conservation. Newbury and Cambridge, UK: Pisces Publications and BirdLife International (BirdLife Conservation Series No. 11). Baha El Din S.M. (2006a) A guide to the Reptiles and Amphibians of Egypt. The American University in Cairo Press. Baha El Din S.M. (2006b) Hemidactylus foudaii. In: IUCN 2012. IUCN Red List of Threatened Species. Version 2012.1. www.iucnredlist.org. Downloaded on 07 August 2012. Baha El Din S.M. (2006c) Tarentola mindiae. In: IUCN 2012. IUCN Red List of Threatened Species. Version 2012.1. www.iucnredlist.org. Downloaded on 02 August 2012. Baha El Din S.M. (2007) A new lizard of the Acanthodactylus scutellatus group (Squamata-Lacertidae) from Egypt. Zoology in the Middle East 40: 21-
Baha El Din S.M., Attum O. & Baha El Din M. (2003) Status of Testudo kleinmanni and T. werneri in Egypt. Chelonian Conservation Biology 4: 648-655. Baldwin R.A. (2009) Use of Maximum Entropy Modeling in Wildlife Research. Entropy 11: 854-866 (DOI: 10.3390/E11040854). Basuony M.I., Gilbert F. & Zalat S. (2010) The Mammals of Egypt: Atlas, Red Data listing & Conservation. BioMAP & CultNat, EEAA & Bibliotheca Alexandrina, Cairo (available at: http://ecology.nottingham.ac.uk/~plzfg/). Beaumont L.J., Hughes L. & Pitman A.J. (2008) Why is the choice of future climate scenarios for species distribution modelling important? Ecology Letters 11: 1135-1146 (DOI: 10.1111/j.1461-0248.2008.01231.x). Bellard C., Bertelsmeier C., Leadley P., Thuiller W. & Courchamp F. (2012) Impacts of climate change on the future of biodiversity. Ecology Letters 15: 365–377 (DOI: 10.1111/j.1461-0248.2011.01736.x). Ben Rais Lasram F., Guilhaumon F., Albouy C., Somot S., Thuiller W., et al. (2010) The Mediterranean Sea as a ‘cul-de-sac’ for endemic fishes facing climate change. Global Change Biology 16: 3233-3245 (DOI: 10.1111/j.1365-2486.2010.02224.x). Beukema W., de Pous P., Donaire D., Escoriza D., Bogaerts S., et al. (2010) Biogeography and contemporary climatic differentiation among Moroccan Salamandra algira. Biological Journal of the Linnean Society 101: 626-641 (DOI: 10.1111/j.1095-8312.2010.01506.x).
- 133 - Bohm M. & Richman N. (2010) Tropiocolotes nubicus. In: IUCN 2012. IUCN Red List of Threatened Species. Version 2012.1. www.iucnredlist.org. Downloaded on 07 August 2012. Böhme W. & Baha El Din S.M. (2006a) Acanthodactylus pardalis. In: IUCN
- IUCN Red List of Threatened Species. Version 2012.1. www.iucnredlist.org. Downloaded on 04 August 2012. Böhme W. & Baha El Din S.M. (2006b) Philochortus zolii. In: IUCN 2012. IUCN Red List of Threatened Species. Version 2012.1. www.iucnredlist.org. Downloaded on 07 August 2012. Bombi P. & D’Amen M. (2011) Scaling down distribution maps from atlas data: a test of different approaches with virtual species. Journal of Biogeography 39: 640–651 (DOI: 10.1111/j.1365-2699.2011.02627.x). Bombi P., Salvi D. & Bologna M.A. (2012) Cross-scale predictions allow the identification of local conservation priorities from atlas data. Animal Conservation 15: 378-387 (DOI: 10.1111/j.1469-1795.2012.00526.x). Boulos L. (1999-2006) Flora of Egypt. Vols I - IV. Al Hadara Publishing, Cairo, Egypt. Boyce M.S., Vernier P.R., Nielsen S.E. & Schmiegelow F.K.A. (2002) Evaluating resource selection functions. Ecological Modelling 157: 281-
Brading P., El-Gabbas A., Zalat S. & Gilbert F. (2009) Biodiversity economics: the value of pollination services to Egypt. Egyptian Journal of Biology 11: 46-51. Brito J.C., Fahd S., Martínez-Freiría F., Tarroso P., Larbes P., et al. (2011) Climate change and peripheral populations: predictions for a relict Mediterranean viper. Acta Herpetologica 6: 105-118. Broennimann O., Thuiller W., Hughes G., Midgley G.F., Alkemade J.M.R., et al. (2006) Do geographic distribution, niche property and life form explain plants’ vulnerability to global change? Global Change Biology 12: 1079-1093 (DOI: 10.1111/j.1365-2486.2006.01157.x). Brotons L., Thuiller W., Araújo M.B. & Hirzel A.H. (2004) Presence-absence versus presence-only modelling methods for predicting bird habitat suitability. Ecography 27: 437-448 (DOI: 10.1111/j.0906- 7590.2004.03764.x). Buisson L., Thuiller W., Casajus N., Lek S. & Grenouillet G. (2010) Uncertainty in ensemble forecasting of species distribution. Global Change Biology 16: 1145-1157 (DOI: 10.1111/j.1365-2486.2009.02000.x). Cadby C.D., While G.M., Hobday A.J., Uller T. & Wapstra E. (2010) Multi-scale approach to understanding climate effects on offspring size at birth and date of birth in a reptile. Integrative Zoology 5: 164-175 (DOI: 10.1111/j.1749-4877.2010.00201.x).
- 134 - Carvalho S.B., Brito J.C., Crespo E.J. & Possingham H.P. (2010) From climate change predictions to actions - conserving vulnerable animal groups in hotspots at a regional scale. Global Change Biology 16: 3257-3270 (DOI: 10.1111/j.1365-2486.2010.02212.x). Carvalho S.B., Brito J.C., Crespo E.J. & Possingham H.P. (2011) Incorporating evolutionary processes into conservation planning using species distribution data: a case study with the western Mediterranean herpetofauna. Diversity & Distributions 17: 408-421 (DOI: 10.1111/j.1472-4642.2011.00752.x). Ciarleglio M., Barnes J.W. & Sarkar S. (2008) ConsNet—A tabu search approach to the spatially coherent conservation area network design problem. Journal of Heuristics 16: 537-557 (DOI: 10.1007/s10732-008- 9098-7). Ciarleglio M., Wesley Barnes J. & Sarkar S. (2009) ConsNet: new software for the selection of conservation area networks with spatial and multi- criteria analyses. Ecography 32: 205-209 (DOI: 10.1111/j.1600- 0587.2008.05721.x). Coetzee B.W.T., Robertson M.P., Erasmus B.F.N., van Rensburg B.J. & Thuiller W. (2009) Ensemble models predict Important Bird Areas in southern Africa will become less effective for conserving endemic birds under climate change. Global Ecology & Biogeography 18: 701-710 (DOI: 10.1111/j.1466-8238.2009.00485.x). Corbalán V., Tognelli M.F., Scolaro J.A. & Roig-Juñent S.A. (2011) Lizards as conservation targets in Argentinean Patagonia. Journal for Nature Conservation 19: 60-67 (DOI: 10.1016/j.jnc.2010.05.004). Costa G.C., Nogueira C., Machado R.B. & Colli G.R. (2010) Sampling bias and the use of ecological niche modeling in conservation planning: a field evaluation in a biodiversity hotspot. Biodiversity & Conservation 19: 883-899 (DOI: 10.1007/s10531-009-9746-8). Crick H.Q.P., Dudley C., Glue D.E. & Thomson D.L. (1997) UK birds are laying eggs earlier. Nature 388: 526-526 (DOI: 10.1038/41453). Currie D.J. (2001) Projected effects of climate change on patterns of vertebrate and tree species richness in the conterminous United States. Ecosystems 4: 216-225 (DOI: 10.1007/s10021-001-0005-4). de Pous P. (2011) Climate change and European reptiles: from large-scale spatial range shift projections towards a more realistic assessment of species responses. M.Sc. thesis, University of East Anglia, UK (pp 64). de Pous P., Beukema W., Weterings M., Dümmer I. & Geniez P. (2011) Area prioritization and performance evaluation of the conservation area network for the Moroccan herpetofauna: a preliminary assessment. Biodiversity & Conservation 20: 89-118 (DOI: 10.1007/s10531-010- 9948-0).
- 135 - DeMatteo K.E. & Loiselle B.A. (2008) New data on the status and distribution of the bush dog (Speothos venaticus): Evaluating its quality of protection and directing research efforts. Biological Conservation 141: 2494-2505 (DOI: 10.1016/j.biocon.2008.07.010). Dennis R.L.H. & Thomas C.D. (2000) Bias in butterfly distribution maps: the influence of hot spots and recorder’s home range. Journal of Insect Conservation 4: 73-77 (DOI: 10.1023/a:1009690919835). Disi M., Werner Y. & Baha El Din S.M. (2006) Telescopus hoogstraali. In: IUCN 2012. IUCN Red List of Threatened Species. Version 2012.1. www.iucnredlist.org. Downloaded on 07 August 2012. Domisch S., Jahnig S.C. & Haase P. (2011) Climate-change winners and losers: stream macroinvertebrates of a submontane region in Central Europe. Freshwater Biology 56: 2009-2020 (DOI: 10.1111/j.1365- 2427.2011.02631.x). Edgar P., Foster J. & Baker J. (2010) Reptile Habitat Management Handbook. Amphibian and Reptile Conservation, Bournemouth. Egbert S.L., Martínez-Meyer E., Ortega-Huerta M. & Peterson A.T. (2002) Use of datasets derived from time-series AVHRR imagery as surrogates for land cover maps in predicting species’ distributions. Igarss 2002: Ieee International Geoscience and Remote Sensing Symposium and 24 th
Canadian Symposium on Remote Sensing, Vols I-Vi, Proceedings: 2337-2339. El Alqamy H., Ismael A., Abdelhameed A., Nagy A., Hamada A., et al. (2010) Predicting the status and distribution of the Nubian Ibex (Capra nubiana) in the high-altitude mountains of South Sinai (Egypt). Galemys: Newsletter of the Spanish Society for the Preservation and Study of Mammals 22: 517-530. Elith J., Graham C.H., Anderson R.P., Dudík M., Ferrier S., et al. (2006) Novel methods improve prediction of species’ distributions from occurrence data. Ecography 29: 129-151 (DOI: 10.1111/j.2006.0906- 7590.04596.x). Elith J., Kearney M. & Phillips S. (2010) The art of modelling range-shifting species. Methods in Ecology & Evolution 1: 330-342 (DOI: 10.1111/j.2041-210X.2010.00036.x). Elith J. & Leathwick J. (2007) Predicting species distributions from museum and herbarium records using multiresponse models fitted with multivariate adaptive regression splines. Diversity & Distributions 13: 265-275 (DOI: 10.1111/j.1472-4642.2007.00340.x). Elith J. & Leathwick J.R. (2009) Species Distribution Models: ecological explanation and prediction across space and time. Annual Review of Ecology Evolution & Systematics 40: 677-697 (DOI: 10.1146/annurev.ecolsys.110308.120159).
136 - Elith J., Phillips S.J., Hastie T., Dudik M., Chee Y.E., et al. (2011) A statistical explanation of MaxEnt for ecologists. Diversity & Distributions 17: 43-57 (DOI: 10.1111/j.1472-4642.2010.00725.x). Farren A., Prodöhl P.A., Laming P. & Reid N. (2010) Distribution of the common lizard (Zootoca vivipara) and landscape favourability for the species in Northern Ireland. Amphibia-Reptilia 31: 387-394 (DOI: 10.1163/156853810791769428). Feeley K.J. & Silman M.R. (2011) Keep collecting: accurate species distribution modelling requires more collections than previously thought. Diversity & Distributions 17: 1132-1140 (DOI: 10.1111/j.1472- 4642.2011.00813.x). Fernández-Moya J., San Miguel-Ayanz A., Cañellas I. & Gea-Izquierdo G. (2010) Variability in Mediterranean annual grassland diversity driven by small-scale changes in fertility and radiation. Plant Ecology 212: 865- 877 (DOI: 10.1007/s11258-010-9869-8). Ficetola G.F., Thuiller W. & Miaud C. (2007) Prediction and validation of the potential global distribution of a problematic alien invasive species - the American bullfrog. Diversity & Distributions 13: 476-485 (DOI: 10.1111/j.1472-4642.2007.00377.x). Fitzpatrick M.C., Gove A.D., Sanders N.J. & Dunn R.R. (2008) Climate change, plant migration, and range collapse in a global biodiversity hotspot: the Banksia (Proteaceae) of Western Australia. Global Change Biology 14: 1337-1352 (DOI: 10.1111/j.1365-2486.2008.01559.x). Flory A.R., Kumar S., Stohlgren T.J. & Cryan P.M. (2012) Environmental conditions associated with bat white-nose syndrome mortality in the north-eastern United States. Journal of Applied Ecology 49: 680-689 (DOI: 10.1111/j.1365-2664.2012.02129.x). Fouquet A., Ficetola G.F., Haigh A. & Gemmell N. (2010) Using ecological niche modelling to infer past, present and future environmental suitability for Leiopelma hochstetteri, an endangered New Zealand native frog. Biological Conservation 143: 1375-1384 (DOI: 10.1016/j.biocon.2010.03.012). Franklin J. (2009) Mapping species distributions: spatial Inference and prediction. Cambridge University Press, Cambridge, UK. Franklin J., Wejnert K.E., Hathaway S.A., Rochester C.J. & Fisher R.N. (2009) Effect of species rarity on the accuracy of species distribution models for reptiles and amphibians in southern California. Diversity & Distributions 15: 167-177 (DOI: 10.1111/j.1472-4642.2008.00536.x). Game E.T. & Grantham H.S. (2008) Marxan user manual: for Marxan version 1.8.10. Univ. of Queensland, St. Lucia, Queensland, Australia, and Pacific Marine Analysis and Research Association, Vancouver, BC, Canada (www.uq.edu.au/marxan/index.html).
137 - Geffen E. (1990) The threatenned Egyptian tortoise (Testudo kleinmanni) proposal for a reserve at Holot Agur (western Negev, Israel). British Herpetological Society Bulletin No. 32. Gilbert F. & Zalat S. (2008) The Butterflies of Egypt: Atlas, Red Data listing & Conservation. BioMAP, EEAA, Cairo (available at: http://ecology.nottingham.ac.uk/~plzfg/). Golicher D.J., Cayuela L. & Newton A.C. (2012) Effects of Climate Change on the Potential Species Richness of Mesoamerican Forests. Biotropica 44: 284-293 (DOI: 10.1111/j.1744-7429.2011.00815.x). Graham C.H., Elith J., Hijmans R.J., Guisan A., Peterson A.T., et al. (2008) The influence of spatial errors in species occurrence data used in distribution models. Journal of Applied Ecology 45: 239-247 (DOI: 10.1111/j.1365-2664.2007.01408.x). Graham C.H., Ferrier S., Huettman F., Moritz C. & Peterson A.T. (2004) New developments in museum-based informatics and applications in biodiversity analysis. Trends in Ecology & Evolution 19: 497-503 (DOI: 10.1016/j.tree.2004.07.006). Graham C.H. & Hijmans R.J. (2006) A comparison of methods for mapping species ranges and species richness. Global Ecology & Biogeography 15: 578-587 (DOI: 10.1111/j.1466-822x.2006.00257.x). Grenouillet G., Buisson L., Casajus N. & Lek S. (2011) Ensemble modelling of species distribution: the effects of geographical and environmental ranges. Ecography 34: 9-17 (DOI: 10.1111/j.1600-0587.2010.06152.x). Guisan A. & Rahbek C. (2011) SESAM - a new framework integrating macroecological and species distribution models for predicting spatio- temporal patterns of species assemblages. Journal of Biogeography 38: 1433-1444 (DOI: 10.1111/j.1365-2699.2011.02550.x). Guisan A. & Thuiller W. (2005) Predicting species distribution: offering more than simple habitat models. Ecology Letters 8: 993-1009 (DOI: 10.1111/j.1461-0248.2005.00792.x). Hannah L. (2011) Climate Change Biology. Elsevier Ltd. Hannah L., Lovejoy T.E. & Schneider S.H. (2005) Biodiversity and climate change in context in Lovejoy TE, Hannah L, eds. Climate Change and Biodiversity, Yale University Press, New Haven, CT, USA. Hernandez P.A., Graham C.H., Master L.L. & Albert D.L. (2006) The effect of sample size and species characteristics on performance of different species distribution modeling methods. Ecography 29: 773-785 (DOI: 10.1111/j.0906-7590.2006.04700.x). Hijmans R.J., Cameron S.E., Parra J.L., Jones P.G. & Jarvis A. (2005) Very high resolution interpolated climate surfaces for global land areas.
138 - International Journal of Climatology 25: 1965-1978 (DOI: 10.1002/Joc.1276). Hirzel A.H., Hausser J., Chessel D. & Perrin N. (2002) Ecological-Niche Factor Analysis: How to Compute Habitat-Suitability Maps without Absence Data? Ecology 83: 2027-2036 (DOI: 10.1890/0012- 9658(2002)083[2027:enfaht]2.0.co;2). Hirzel A.H. & Le Lay G. (2008) Habitat suitability modelling and niche theory. Journal of Applied Ecology 45: 1372-1381 (DOI: 10.1111/j.1365- 2664.2008.01524.x). Holcombe T.R., Stohlgren T.J. & Jarnevich C.S. (2010) From points to forecasts: predicting invasive species habitat suitability in the near term. Diversity 2: 738-767 (DOI: 10.3390/d2050738). Holt A.C., Salkeld D.J., Fritz C.L., Tucker J.R. & Gong P. (2009) Spatial analysis of plague in California: niche modeling predictions of the current distribution and potential response to climate change. International Journal of Health Geographics 8: 38 (DOI: 10.1186/1476- 072X-8-38). Hoyle M. & James M. (2005) Global warming, human population pressure, and viability of the world’s smallest butterfly. Conservation Biology 19: 1113- 1124 (DOI: 10.1111/j.1523-1739.2005.00166.x). Hu J. & Jiang Z. (2011) Climate change hastens the conservation urgency of an endangered ungulate. PLoS One 6: e22873 (DOI: 10.1371/journal.pone.0022873). Huang Y., Dai Q., Chen Y.Y., Wan H.F., Li J.T., et al. (2011) Lizard species richness patterns in China and its environmental associations. Biodiversity & Conservation 20: 1399-1414 (DOI: 10.1007/s10531-011- 0033-0). Hughes L. (2000) Biological consequences of global warming: is the signal already apparent? Trends in Ecology & Evolution 15: 56-61. Hutchinson G.E. (1957) Concluding remarks. Cold Spring Harbour Symposium on Quantitative Biology 22: 415-427. Ibanez I., Clark J.S., Dietze M.C., Feeley K., Hersh M., et al. (2006) Predicting biodiversity change: outside the climate envelope, beyond the species- area curve. Ecology 87: 1896-1906. Ihlow F., Dambach J., Engler J.O., Flecks M., Hartmann T., et al. (2012) On the brink of extinction? How climate change may affect global chelonian species richness and distribution. Global Change Biology 18: 1520- 1530 (DOI: 10.1111/j.1365-2486.2011.02623.x). IPCC (2007) Climate Change 2007: Synthesis Report. Contribution of Working Groups I, II and III to the Fourth Assessment Report of the
139 - Intergovernmental Panel on Climate Change. IPCC, Geneva, Switzerland. IUCN Standards and Petitions Subcommittee (2010) Guidelines for Using the IUCN Red List Categories and Criteria. Version 8.1. Downloadable from http://intranet.iucn.org/webfiles/doc/SSC/RedList/RedListGuidelines.pdf. Jarnevich C.S. & Reynolds L.V. (2011) Challenges of predicting the potential distribution of a slow-spreading invader: a habitat suitability map for an invasive riparian tree. Biological Invasions 13: 153-163 (DOI: 10.1007/s10530-010-9798-4). Jiménez-Valverde A., Lobo J.M. & Hortal J. (2008) Not as good as they seem: the importance of concepts in species distribution modelling. Diversity & Distributions 14: 885-890 (DOI: 10.1111/j.1472-4642.2008.00496.x). Kadmon R., Farber O. & Danin A. (2003) A systematic analysis of factors affecting the performance of climatic envelope models. Ecological Applications 13: 853-867 (DOI: 10.1890/1051- 0761(2003)013[0853:ASAOFA]2.0.CO;2). Kadmon R., Farber O. & Danin A. (2004) Effect of roadside bias on the accuracy of predictive maps produced by bioclimatic models. Ecological Applications 14: 401-413 (DOI: 10.1890/02-5364). Kelly M. & Meentemeyer R.K. (2002) Landscape dynamics of the spread of sudden oak death. Photogrammetric Engineering & Remote Sensing 68: 1001-1009. Kgosiesele E. (2010) Predictive distribution modelling of Timon lepida in Spain. M.Sc. thesis, University of Twente (ITC), the Netherlands (pp 87). Klorvuttimontara S. (2010) Modelling the effectiveness of protected areas for conserving tropical species under future climate warming. PhD. Leach K. (2011) How effective will Egypt’s protected areas network be under future climate change. M.Sc. thesis, the University of Nottingham, UK (pp 85). Levine R.S., Peterson A.T. & Benedict M.Q. (2004) Geographic and ecologic distributions of the Anopheles gambiae complex predicted using a genetic algorithm. American Journal of Tropical Medicine & Hygiene 70: 105-109. Levinsky I., Skov F., Svenning J.C. & Rahbek C. (2007) Potential impacts of climate change on the distributions and diversity patterns of European mammals. Biodiversity & Conservation 16: 3803-3816 (DOI: 10.1007/s10531-007-9181-7). Leyequien E., Verrelst J., Slot M., Schaepman-Strub G., Heitkönig I.M.A., et al. (2007) Capturing the fugitive: Applying remote sensing to terrestrial
140 - animal distribution and diversity. International Journal of Applied Earth Observation & Geoinformation 9: 1-20 (DOI: 10.1016/j.jag.2006.08.002). Liu C.R., Berry P.M., Dawson T.P. & Pearson R.G. (2005) Selecting thresholds of occurrence in the prediction of species distributions. Ecography 28: 385-393 (DOI: 10.1111/j.0906-7590.2005.03957.x). Lobo J.M., Jimenez-Valverde A. & Real R. (2008) AUC: a misleading measure of the performance of predictive distribution models. Global Ecology & Biogeography 17: 145-151 (DOI: 10.1111/j.1466-8238.2007.00358.x). Luoto M., Poyry J., Heikkinen R.K. & Saarinen K. (2005) Uncertainty of bioclimate envelope models based on the geographical distribution of species. Global Ecology & Biogeography 14: 575-584 (DOI: 10.1111/j.1466-822x.2005.00186.x). Maiorano L., Falcucci A., Zimmermann N.E., Psomas A., Pottier J., et al. (2011) The future of terrestrial mammals in the Mediterranean basin under climate change. Philosophical Transactions of the Royal Society of London (Series B: Biological Sciences) 366: 2681-2692 (DOI: 10.1098/rstb.2011.0121). Marini M.A., Barbet-Massin M., Lopes L.E. & Jiguet F. (2009) Predicted climate-driven bird distribution changes and forecasted conservation conflicts in a neotropical savanna. Conservation Biology 23: 1558-1567 (DOI: 10.1111/j.1523-1739.2009.01258.x). Marmion M., Parviainen M., Luoto M., Heikkinen R.K. & Thuiller W. (2009) Evaluation of consensus methods in predictive species distribution modelling. Diversity & Distributions 15: 59-69 (DOI: 10.1111/j.1472- 4642.2008.00491.x). Martínez-Meyer E. (2005) Climate change and biodiversity: some considerations in forecasting shifts in species’ potential distributions. Biodiversity Informatics 2: 42-55. Meentemeyer R.K., Cunniffe N.J., Cook A.R., Filipe J.A.N., Hunter R.D., et al. (2011) Epidemiological modeling of invasion in heterogeneous landscapes: spread of sudden oak death in California (1990–2030). Ecosphere 2: art17 (DOI: 10.1890/es10-00192.1). Mika A.M., Weiss R.M., Olfert O., Hallett R.H. & Newman J.A. (2008) Will climate change be beneficial or detrimental to the invasive swede midge in North America? Contrasting predictions using climate projections from different general circulation models. Global Change Biology 14: 1721-1733 (DOI: 10.1111/j.1365-2486.2008.01620.x). Moffett A., Garson J. & Sarkar S. (2005) MultCSync: a software package for incorporating multiple criteria in conservation planning. Environmental Modelling & Software 20: 1315-1322 (DOI: 10.1016/j.envsoft.2004.10.001).
141 - Moilanen A. (2007) Landscape Zonation, benefit functions and target-based planning: Unifying reserve selection strategies. Biological Conservation 134: 571-579 (DOI: 10.1016/j.biocon.2006.09.008). Moilanen A., Franco A.M., Early R.I., Fox R., Wintle B., et al. (2005) Prioritizing multiple-use landscapes for conservation: methods for large multi- species planning problems. Proceedings of the Royal Society of London Series B-Biological Sciences 272: 1885-1891 (DOI: 10.1098/rspb.2005.3164). Moilanen A., Meller L., Leppänen, J., Pouzols F.M., Arponen A., et al. (2012) Zonation spatial conservation planning framework and software v.3.1, User manual. www.helsinki.fi/bioscience/consplan. Morueta-Holme N., Flojgaard C. & Svenning J.C. (2010) Climate change risks and conservation implications for a threatened small-range mammal species. PLoS One 5: e10360 (DOI: 10.1371/journal.pone.0010360). Newbold T. (2009) The value of species distribution models as a tool for conservation and ecology in Egypt and Britain. Ph.D. thesis, University of Nottingham, UK (312p). Newbold T. (2010) Applications and limitations of museum data for conservation and ecology, with particular attention to species distribution models. Progress in Physical Geography 34: 3-22 (DOI: 10.1177/0309133309355630). Newbold T., Gilbert F., Zalat S., El-Gabbas A. & Reader T. (2009a) Climate- based models of spatial patterns of species richness in Egypt’s butterfly and mammal fauna. Journal of Biogeography 36: 2085-2095 (DOI: 10.1111/j.1365-2699.2009.02140.x). Newbold T., Reader T., El-Gabbas A., Berg W., Shohdi W.M., et al. (2010) Testing the accuracy of species distribution models using species records from a new field survey. Oikos 119: 1326-1334 (DOI: 10.1111/j.1600-0706.2009.18295.x). Newbold T., Reader T., Zalat S., El-Gabbas A. & Gilbert F. (2009b) Effect of characteristics of butterfly species on the accuracy of distribution models in an arid environment. Biodiversity & Conservation 18: 3629- 3641 (DOI: 10.1007/s10531-009-9668-5). Niamir A. (2009) Distribution modelling of the Short-toed Eagle in relation to potential food availability. M.Sc. thesis, University of Twente (ITC), the Netherlands (pp 75). Nori J., Urbina-Cardona J.N., Loyola R.D., Lescano J.N. & Leynaud G.C. (2011) Climate change and American Bullfrog invasion: what could we expect in South America? PLoS One 6: e25718 (DOI: 10.1371/journal.pone.0025718).
142 - Parmesan C. (2006) Ecological and evolutionary responses to recent climate change. Annual Review of Ecology Evolution & Systematics 37: 637- 669 (DOI: 10.1146/annurev.ecolsys.37.091305.110100). Parry M.L., Canziani O.F., Palutikof J.P., van der Linden P.J. & Hanson C.E. (2007) Climate change 2007: impacts, adaptation and vulnerability (Contribution of Working Group II to the Fourth Assessment Report of the Intergovernmental Panel on Climate Change, Cambridge, UK): Cambridge University Press: 211-272. Pearson R.G. (2007) Species’ distribution modeling for conservation educators and practitioners. Synthesis. American Museumof Natural History. Available at http://ncep.amnh.org. Pearson R.G. & Dawson T.P. (2003) Predicting the impacts of climate change on the distribution of species: are bioclimate envelope models useful? Global Ecology & Biogeography 12: 361-371 (DOI: 10.1046/j.1466- 822X.2003.00042.x). Pearson R.G., Raxworthy C.J., Nakamura M. & Peterson A.T. (2007) Predicting species distributions from small numbers of occurrence records: a test case using cryptic geckos in Madagascar. Journal of Biogeography 34: 102-117 (DOI: 10.1111/j.1365-2699.2006.01594.x). Perälä J. (2003) Testudo kleinmanni. In: IUCN 2012. IUCN Red List of Threatened Species. Version 2012.1. www.iucnredlist.org. Downloaded on 30 July 2012. Perälä J. (2006) Assessment of the Threatened Status of Testudo werneri Perälä, 2001 (Testudines: Testudinidae) for the IUCN Red List. Chelonian Conservation & Biology 5: 57-66 (DOI: 10.2744/1071- 8443(2006)5[57:aottso]2.0.co;2). Peterson A.T. (2006) Ecologic niche modeling and spatial patterns of disease transmission. Emerging Infectious Disease 12: 1822-1826. Peterson A.T., Ortega-Huerta M.A., Bartley J., Sanchez-Cordero V., Soberon J., et al. (2002) Future projections for Mexican faunas under global climate change scenarios. Nature 416: 626-629 (DOI: 10.1038/416626a). Peterson A.T., Soberón J., Pearson R.G., Anderson R.P., Martínez-Meyer E., et al. (2011) Ecological Niches and Geographic Distributions. Princeton University Press. Phillips S.J., Anderson R.P. & Schapire R.E. (2006) Maximum entropy modeling of species geographic distributions. Ecological Modelling 190: 231-259 (DOI: 10.1016/j.ecolmodel.2005.03.026). Phillips S.J. & AT&T Research (2011) A brief tutorial on maxent. Available at: http://www.cs.princeton.edu/~schapire/maxent/tutorial/tutorial.doc - Last accessed 29 May 2012.
143 - Phillips S.J. & Dudik M. (2008) Modeling of species distributions with Maxent: new extensions and a comprehensive evaluation. Ecography 31: 161- 175 (DOI: 10.1111/j.0906-7590.2008.5203.x). Phillips S.J., Schapire R.E. & Dudík M. 2004. A Maximum Entropy Approach to Species Distribution Modeling. Proceedings of the 21st International Conference on Machine Learning, Banff, Canada. Pineda E. & Lobo J.M. (2009) Assessing the accuracy of species distribution models to predict amphibian species richness patterns. Journal of Animal Ecology 78: 182-190 (DOI: 10.1111/j.1365-2656.2008.01471.x). Pulliam H.R. (2000) On the relationship between niche and distribution. Ecology Letters 3: 349-361 (DOI: 10.1046/j.1461-0248.2000.00143.x). R Development Core Team (2012) R: A language and environment for statistical computing. R Foundation for Statistical Computing, Vienna, Austria. ISBN 3-900051-07-0, URL http://www.R-project.org/. Rashid M.R. (2007) Monitoring Habitat Change and Its Relation to Sand Lizard Population Dynamics with Multi Temporal Remote Sensing: A Case Study of Terschelling and Vlieland, The Netherlands. M.Sc. thesis, University of Twente (ITC), the Netherlands (pp 59). Raxworthy C.J., Martínez-Meyer E., Horning N., Nussbaum R.A., Schneider G.E., et al. (2003) Predicting distributions of known and unknown reptile species in Madagascar. Nature 426: 837-841 (DOI: 10.1038/nature02205). Reddy S. & Dávalos L.M. (2003) Geographical sampling bias and its implications for conservation priorities in Africa. Journal of Biogeography 30: 1719-1727 (DOI: 10.1046/j.1365- 2699.2003.00946.x). Reside A.E., Watson I., VanDerWal J. & Kutt A.S. (2011) Incorporating low- resolution historic species location data decreases performance of distribution models. Ecological Modelling 222: 3444-3448 (DOI: 10.1016/j.ecolmodel.2011.06.015). Richmond O.M., McEntee J.P., Hijmans R.J. & Brashares J.S. (2010) Is the climate right for pleistocene rewilding? Using species distribution models to extrapolate climatic suitability for mammals across continents. PLoS One 5: e12899 (DOI: 10.1371/journal.pone.0012899). Rödder D. (2009) How to predict the future? On niches and potential distributions of amphibians and reptiles in a changing climate Ph.D. thesis, Friedrich-Wilhelms-Universität Bonn (291p). Rödder D., Kielgast J., Bielby J., Schmidtlein S., Bosch J., et al. (2009) Global amphibian extinction risk assessment for the panzootic chytrid fungus. Diversity 1: 52-66 (DOI: 10.3390/d1010052).
144 - Rödder D., Schmidtlein S., Schick S. & Lötters S. (2011) Climate envelope models in systematics and evolutionary research: theory and practice. in Hodkinson T, Jones MB, Waldren S, Parnell JAN, eds. Climate change, ecology and systematics, Cambridge University Press, Cambridge (UK), 524 pp.: 243-264. Rödder D. & Weinsheimer F. (2009) Will future anthropogenic climate change increase the potential distribution of the alien invasive Cuban treefrog (Anura: Hylidae)? Journal of Natural History 43: 1207-1217 (DOI: 10.1080/00222930902783752). Saathoff E., Olsen A., Sharp B., Kvalsvig J.D., Appleton C.C., et al. (2005) Ecologic covariates of hookworm infection and reinfection in rural Kwazulu-natal/south Africa: a geographic information system-based study. American Journal of Tropical Medicine & Hygiene 72: 384-391. Sanchez A.C., Osborne P.E. & Haq N. (2011) Climate change and the African baobab (Adansonia digitata L.): the need for better conservation strategies. African Journal of Ecology 49: 234-245 (DOI: 10.1111/j.1365-2028.2011.01257.x). Sarkar S., Aggarwal A., Garson J., Margules C.R. & Zeidler J. (2002) Place prioritization for biodiversity content. Journal of Biosciences 27: 339- 346 (DOI: 10.1007/bf02704964). Sauer J., Domisch S., Nowak C. & Haase P. (2011) Low mountain ranges: summit traps for montane freshwater species under climate change. Biodiversity & Conservation 20: 3133-3146 (DOI: 10.1007/s10531-011- 0140-y). Saupe E.E., Papes M., Selden P.A. & Vetter R.S. (2011) Tracking a medically important spider: climate change, ecological niche modeling, and the brown recluse (Loxosceles reclusa). PLoS One 6: e17731 (DOI: 10.1371/journal.pone.0017731). Sergio C., Figueira R., Draper D., Menezes R. & Sousa A.J. (2007) Modelling bryophyte distribution based on ecological information for extent of occurrence assessment. Biological Conservation 135: 341-351 (DOI: 10.1016/j.biocon.2006.10.018). Simaika J.P. (2012) Practical conservation planning from local to continental scales using freshwater invertebrates.
Soberón J.M., Llorente J.B. & Oñate L. (2000) The use of specimen-label databases for conservation purposes: an example using Mexican Papilionid and Pierid butterflies. Biodiversity & Conservation 9: 1441- 1466 (DOI: 10.1023/a:1008987010383). Songer M., Delion M., Biggs A. & Huang Q. (2012) Modeling Impacts of Climate Change on Giant Panda Habitat. International Journal of Ecology 2012: 1-12 (DOI: 10.1155/2012/108752).145 - Soultan A.A. (2011) Identifying the current and future distribution of antelopes in Egypt under different scenarios of climate change. M.Sc. thesis, University of East Anglia, UK (pp 95). Spawls S. (2011) Uromastyx ocellata. In: IUCN 2012. IUCN Red List of Threatened Species. Version 2012.1. www.iucnredlist.org. Downloaded on 04 August 2012. Stockwell D. & Peters D. (1999) The GARP modelling system: problems and solutions to automated spatial prediction. International Journal of Geographical Information Science 13: 143-158 (DOI: 10.1080/136588199241391). Stockwell D.R.B. & Peterson A.T. (2002) Effects of sample size on accuracy of species distribution models. Ecological Modelling 148: 1-13 (DOI: 10.1016/S0304-3800(01)00388-X). Suarez A.V. & Tsutsui N.D. (2004) The value of museum collections for research and society. Bioscience 54: 66 (DOI: 10.1641/0006- 3568(2004)054[0066:tvomcf]2.0.co;2). Taheri S. (2010) Hyper temporal NDVI images for modelling and prediction the habitat distribution of Balkan green lizard (Lacerta trilineata) - Case study: Crete (Greece). M.Sc. thesis, University of Twente (ITC), the Netherlands (pp 54). Tarkhnishvili D., Serbinova I. & Gavashelishvili A. (2009) Modelling the range of Syrian spadefoot toad (Pelobates syriacus) with combination of GIS- based approaches. Amphibia-Reptilia 30: 401-412 (DOI: 10.1163/156853809788795137). Taubmann J., Theissinger K., Feldheim K.A., Laube I., Graf W., et al. (2011) Modelling range shifts and assessing genetic diversity distribution of the montane aquatic mayfly Ameletus inopinatus in Europe under climate change scenarios. Conservation Genetics 12: 503-515 (DOI: 10.1007/s10592-010-0157-x). Thomas C.D., Cameron A., Green R.E., Bakkenes M., Beaumont L.J., et al. (2004) Extinction risk from climate change. Nature 427: 145-148 (DOI: 10.1038/nature02121). Thorn J.S., Nijman V., Smith D. & Nekaris K.A.I. (2009) Ecological niche modelling as a technique for assessing threats and setting conservation priorities for Asian slow lorises (Primates: Nycticebus). Diversity & Distributions 15: 289-298 (DOI: 10.1111/j.1472-4642.2008.00535.x). Thuiller W. (2004) Patterns and uncertainties of species’ range shifts under climate change. Global Change Biology 10: 2020-2027 (DOI: 10.1111/j.1365-2486.2004.00859.x). Thuiller W. (2007) Biodiversity: climate change and the ecologist. Nature 448: 550-552 (DOI: 10.1038/448550a).
146 - Thuiller W., Lafourcade B., Engler R. & Araújo M.B. (2009) BIOMOD – a platform for ensemble forecasting of species distributions. Ecography 32: 369-373 (DOI: 10.1111/j.1600-0587.2008.05742.x). Thuiller W., Lavorel S., Araújo M.B., Sykes M.T. & Prentice I.C. (2005) Climate change threats to plant diversity in Europe. Proceedings of the National Academy of Sciences USA 102: 8245-8250 (DOI: 10.1073/pnas.0409902102). Tognelli M.F., Abba A.M., Bender J.B. & Seitz V.P. (2011) Assessing conservation priorities of xenarthrans in Argentina. Biodiversity & Conservation 20: 141-151 (DOI: DOI 10.1007/s10531-010-9951-5). Tolba M.K. & Saab N.W. (2009) Arab environment: climate change - impact of climate change on arab countries. Arab forum for environment and dvelopment. Torres J., Brito J.C., Vasconcelos M.J., Catarino L., Goncalves J., et al. (2010) Ensemble models of habitat suitability relate chimpanzee (Pan troglodytes) conservation to forest and landscape dynamics in Western Africa. Biological Conservation 143: 416-425 (DOI: 10.1016/j.biocon.2009.11.007). Ward D.F. (2007) Modelling the potential geographic distribution of invasive ant species in New Zealand. Biological Invasions 9: 723-735 (DOI: 10.1007/s10530-006-9072-y). Warren D.L. & Seifert S.N. (2011) Ecological niche modeling in Maxent: the importance of model complexity and the performance of model selection criteria. Ecological Applications 21: 335-342 (DOI: 10.1890/10-1171.1). Watts M.E., Ball I.R., Stewart R.S., Klein C.J., Wilson K., et al. (2009) Marxan with Zones: Software for optimal conservation based land- and sea-use zoning. Environmental Modelling & Software 24: 1513-1521 (DOI: 10.1016/j.envsoft.2009.06.005). Werner Y. & Baha El Din S.M. (2006a) Mesalina bahaeldini. In: IUCN 2012. IUCN Red List of Threatened Species. Version 2012.1. www.iucnredlist.org. Downloaded on 04 August 2012. Werner Y. & Baha El Din S.M. (2006b) Trapelus savignii. In: IUCN 2012. IUCN Red List of Threatened Species. Version 2012.1. www.iucnredlist.org. Downloaded on 04 August 2012. Werner Y.L. & Ashkenazi S. (2010) Notes on some Egyptian Lacertidae, including a new subspecies of Mesalina, involving the Seligmann effect. Turkish Journal of Zoology 34: 123-133 (DOI: 10.3906/Zoo-0905-8). Wieczorek J., Guo Q.G. & Hijmans R.J. (2004) The point-radius method for georeferencing locality descriptions and calculating associated uncertainty. International Journal of Geographical Information Science 18: 745-767 (DOI: 10.1080/13658810412331280211).
147 - Williams P.H. (2001) WORLDMAP Version 4. Priority areas for biodiversity. http://www.nhm.ac.uk/science/projects/worldmap
Wilms T.M., Wagner P., Shobrak M., Rödder D. & Böhme W. (2011) Living on the edge? – On the thermobiology and activity pattern of the large herbivorous desert lizard Uromastyx aegyptia microlepis Blanford, 1875 at Mahazat as-Sayd Protected Area, Saudi Arabia. Journal of Arid Environments 75: 636-647 (DOI: 10.1016/j.jaridenv.2011.02.003). Wisz M.S., Hijmans R.J., Li J., Peterson A.T., Graham C.H., et al. (2008) Effects of sample size on the performance of species distribution models. Diversity & Distributions 14: 763-773 (DOI: 10.1111/j.1472- 4642.2008.00482.x). Wooten J.A. & Gibbs H.L. (2012) Niche divergence and lineage diversification among closely related Sistrurus rattlesnakes. Journal of Evolutionary Biology 25: 317-328 (DOI: 10.1111/j.1420-9101.2011.02426.x). Yen J.D.L., Thomson J.R., Vesk P.A. & Mac Nally R. (2011) To what are woodland birds responding? Inference on relative importance of in-site habitat variables using several ensemble habitat modelling techniques. Ecography 34: 946-954 (DOI: 10.1111/j.1600-0587.2011.06651.x). Yost A.C., Petersen S.L., Gregg M. & Miller R. (2008) Predictive modeling and mapping sage grouse (Centrocercus urophasianus) nesting habitat using Maximum Entropy and a long-term dataset from Southern Oregon. Ecological Informatics 3: 375-386 (DOI: 10.1016/j.ecoinf.2008.08.004). Zabalaga N.A. (2009) Influence of vegetation types and environmental variables in structuring Podarcis erhardii spatial heterogeneity in Crete, Greece. M.Sc. thesis, University of Twente (ITC), the Netherlands (60p). Zahran M.A. & Willis A.J. (2009) The Vegetation of Egypt, 2nd edn. Springer Publisher, Germany. Zeilhofer P., dos Santos E.S., Ribeiro A.L., Miyazaki R.D. & dos Santos M.A. (2007) Habitat suitability mapping of Anopheles darlingi in the surroundings of the Manso hydropower plant reservoir, Mato Grosso, Central Brazil. International Journal of Health Geographic 6: 7 (DOI: 10.1186/1476-072X-6-7).148 - Appendices
Appendix 1: A full list of the published literature used to extract records
Al-Hussaini AH (1959) The vertebrate fauna of the Bahariya oasis (excluding fishes and mammals). Ain Shams Science Bulletin 4:103-107. Badir MA & El-Nagar MH (1994) Some ecological studies on the fauna of Saint Katherine area in South Sinai with special reference to the Agamid lizard Agma stellio. Journal of the Egyptian-German Society for Zoology 13 (A):67-84. Baha El Din S (2011) Distribution and Recent Range Extension of Natrix tessellata in Egypt. Mertensiella 18:401-403. Bedir MA et al. (1999) Ecological studies on two lizard species inhabiting Wadi El-Arbein at Saint Katherine Area, South Sinai with special reference to biotic elements. Journal of the Egyptian-German Society for Zoology 28(A): 485–503. Farid M (1977) On some fishes, Amphibia, and reptilia from Siwa oasis. Proceedings of the Zoological Society of the A.R.E. 6: 234-231. Fowler HW (1914) Fishes and Reptiles from Assuan, Egypt. Copeia 8: 1-2. Ghazali OF & Baha El Din S (2005) On the occurrence of Psammophis punctulatus Bibron & Dumeril 1854 in Egypt. Herpetological Bulletin 94: 4-5. Ghobashi A (1990) Herpetofaunal survey of Al-Arish area (Norh Sinai) with special reference to their habitat and seasonal distribution. Proceedings of the Zoological Society of the A.R.E. 21: 273-290. Ibrahim A (2000) A radiotelemetric study of the body temperature of Varanus griseus (Sauria: Varanidae) in Zaranik Protected Area, North Sinai, Egypt. Egyptian Journal of Biology 2: 57-66. Ibrahim A (2000) Geographic distribution: Hemidactyllls flaviviridis. Herpetological Review 31(3): 185. Ibrahim A (2000) Geographic distribution: Tarentola annularis annularis. Herpetological Review 31(3): 185. Ibrahim A (2001) A Note on the diet of the white-spotted gecko, Tarentola a. annularis. Gecko 2: 6. Ibrahim A. (2001) Geographic distribution: Bufo regularis. Herpetological Review 32(2): 112. Ibrahim A (2001) Geographic distribution: Coluber florulentus florulentus. Herpetological
- 149 - Review 32(1): 59. Ibrahim A (2001) Geographic distribution: Malpolon monspessulanus insignitus. Herpetological Review 32(2):123. Ibrahim A (2001) Geographic distribution: Ptychadena mascareniensis. Herpetological Review 32(2): 115. Ibrahim A (2001) Geographic distribution: Ptyodactylus h. hasselquistii. Herpetological Review 32(2):120. Ibrahim A (2002) Activity area, movement patterns, and habitat use of the desert monitor, Varanus griseus, in the Zaranik Protected Area, North Sinai, Egypt. African Journal of Herpetology 51(1): 35-45. Ibrahim A (2002) Activity patterns and microhabitat use of the Starred agama Laudakia stellio (Linnaeus, 1758) in Al-Arish city, North Sinai, Egypt. Egyptian Journal of Zoology 39:173-196. Ibrahim A (2007) Ecology of the Mediterranean Gecko, Hemidactylus turcicus (Linnaeus,
- (Reptilia-Gekonidae), in North Sinai, Egypt. Zoology in the Middle East 41:41-54. Ibrahim A (2008) Amphibians and reptiles of the Suez Canal University campuses, Egypt. Herpetological Bulletin, 105:1-9. Ibrahim A et al. (2000) On the ecology of the fringe-toed lizard, Acanthodactylus scutellatus (Sauria: Lacertidae) in north Sinai, Egypt. Journal of the Egyptian- German Society for Zoology 32 (A):335-355. Ibrahim A (2003) Diet and reproduction of the Indian Leaf-toed gecko, Hemidactylus flaviviridis (Sauria: Gekkonnidae), in Ismailia governorate, with consideration to its distribution in the Suez Canal area, Egypt. Journal of the Union of Arab Biologists, Cairo 19(A): Zoology, 125-151. Kamal A (1966) New record of the Amphibians and reptiles of two other districts of the western Egyptian desert. Bulletin de l’Institute du desert d’Egypte 16: 159-167. Kamal A et al. (1966) New record of the Amphibians and reptiles of some districts of the western Egyptian desert. Bulletin de l’Institute du desert d’Egypte 16: 145-157. Kark S et al. (1997) Polymorphism in the snake Psammophis schokari on both sides of the desert edge in Israel and Sinai. Journal of Arid Environments 37: 513-527. Lachman E et al. (2006) Subspeciation befogged by the ‘‘Seligmann effect’’: the case of Laudakia stellio (Reptilia: Sauria: Agamidae) in southern Sinai, Egypt. Journal of Natural History 40(19–20): 1259-1284. Matthew LJ et al. (2007) Recent surveys and comparisons of birds and reptiles in St.
- 150 - Katherine Protectorate, Egypt. Operation Wallacea/BioMAP Report. Meakin K et al. (2005) Monitoring birds, reptiles and butterflies in the St Katherine Protectorate, Egypt. Egyptian Journal of Biology 7: 66-95. Saber SA (1994) Checklist of the Saurian fauna of Souhern Sinai, Egypt. Al-Azhar Bulletin of Science 5(1): 183-201. Saber SA (1994) Herpetological survey of Suez province, Egypt. Bulletin of the Biological Society of Egypt 42. Saber SA (1994) Ophidian community of Sinai, Egypt. Al-Azhar Bulletin of Science 5(1): 203-217. Schmidt KP & Marx H (1957) Results of the NAMRU-3 southeastern Egypt expedition,
- Reptiles and amphibians. Zoological Society of Egypt Bulletin 13:16–28. Shifman S et al. (1999) Tropioclotes nattereri (Reptilia, Gekkonidae): comments on validity, variation and distribution. Zoology in the Middle East, 17:51-66. Tawfik A (1994) Taxonomic analysis of two chamaeleon species (Chamaeleontidae, Reptilia): morphological and biochemical studies. Journal of the Egyptian-German Society for Zoology 15(A): 191-203. Wagner P & Böhme W (2006) A new species of the genus Trapelus Cuvier, 1816 (Squamata: Agamidae) from arid central Africa. Bonner Zoologischer Beiträge 55 (2): 81-87. Wallach V (1999) Geographic distribution: Ramphotyphlops braminus (Brahminy Blind Snake). Herpetological Review 30(4):236. Werner YL & Ashkenazi S (2010) Notes on some Egyptian Lacertidae, including a new subspecies of Mesalina, involving the Seligmann effect. Turkish Journal of Zoology 34: 123-133. Werner YL (1968) Distribution of the Saharan Sphenops sepsoides (Reptilia: Scincidae) in Israel and Jordan. Herpetologica 24(3): 238-242. Werner YL (1983) Lizards and snakes from eastern Lower Egypt in the Hebrew University of Jerusalem and Tel Aviv University with range extensions. Herpetological Review 14(1): 29-31.
- 151 - Appendix 2: List of species not included in this study due to the paucity of unique records:
- Ablepharus rueppellii
- Atractaspis engaddensis
- Dasypeltis scabra
- Dolichophis jugularis
- Hemidactylus foudaii
- Hemidactylus mindiae
- Hemidactylus sinaitus
- Hemorrohis algirus
- Hemorrohis nummifer
- Latastia longicaudata
- Leptotyphlops nursii
- Lycophidion capense
- Mesalina brevirostris
- Mesalina martini
- Ophisops elbaensis
- Ophisops elegans
- Philochortus zolii
- Platyceps sinai
- Psammophis punctulatus
- Pseuderemias mucronata
- Pseudocerastes persicus
- Ptyodactylus ragazzi
- Ramphotyphlops braminus
- Rhyncocalamus melanocephalus
- Telescopus hoogstraali
- Trachemys scripta
- Tropiocolotes nubicus
- Typhlops vermicularis